On the Effectiveness of Recurrent Neural Networks for Live Modeling of Cyber-Physical Systems
2019 IEEE International Conference on Industrial Internet (ICII)(2019)
摘要
Attention to cyber security of cyber-physical systems (CPS) has led to the development of innovative cyber-resilient methodologies to ensure early detection and mitigation of cyber anomalies and threats. The concept of Digital Twin (DT) has recently emerged as one of the approaches to achieve the objective of resilience. In the approach using DT, a software-based live model of a target CPS is used to continuously monitor, surveil and verify the correctness of the target CPS operation. In this paper, we empirically study the effectiveness of Recurrent Neural Network (RNN)-based models as the basis of DT-based resilience. We uncover the important characteristics of an RNN-based solution with experimentation on a lab-scale Canal Lock CPS emulator with live validations and attack scenarios. For the first time, we demonstrate actual, real-time use of a RNN-based model as a DT for performing live analysis on an operational CPS. Based on the observed results, we highlight the importance of a DT model's training interval, prediction interval and CPS polling interval in the process of anomaly detection. We uncover the limitations in anomaly detection due to real-time synchronization needs of the RNN-based DT. We highlight this uncovered tug of war between synchronization and anomaly detection is inherent in any complex CPS that is monitored and synchronized by relying on the same sensor streams of ground truth for both synchronization as well as anomaly detection.
更多查看译文
关键词
CPS live modeling,RNN based Digital Twin,CPS cyber security
AI 理解论文
溯源树
样例
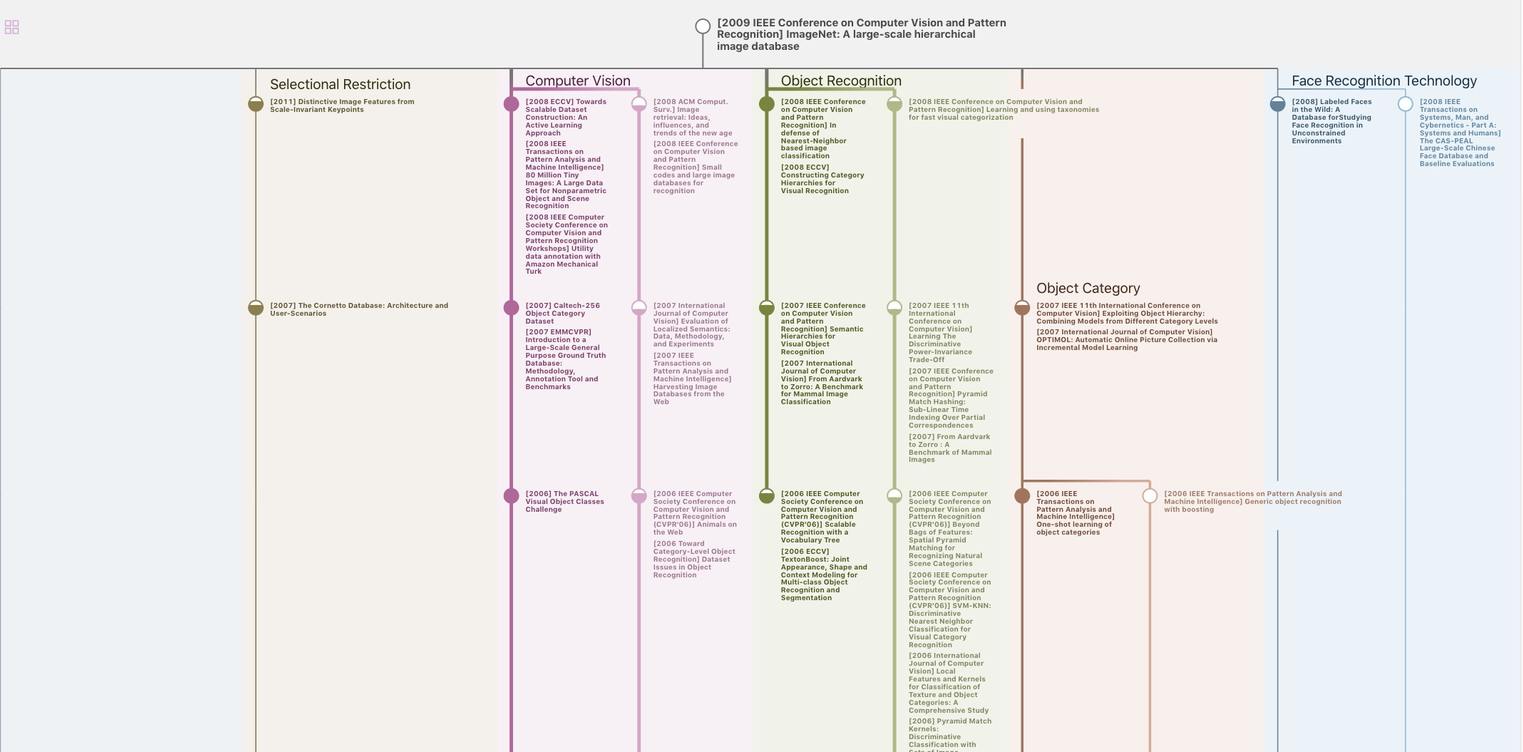
生成溯源树,研究论文发展脉络
Chat Paper
正在生成论文摘要