Constructing and mining structured heterogeneous information networks from massive text corpora
user-5ebe28d54c775eda72abcdf7(2019)
摘要
In today's information society, we are soaked with overwhelming amounts of natural-language text data, ranging from news articles and social media posts to research literature, medical records, and corporate reports. A grand challenge for data miners is to develop effective and scalable methods to mine such massive unstructured text corpora to discover hidden structures and generate structured heterogeneous information networks, from which actionable knowledge can be generated based on user's need. There are three major questions as follows. Can machines automatically ``digest'' a given (domain-specific) corpus and identify real-world entities and their relations mentioned in the corpus? Can human experts efficiently understand and consume the sophisticated, gigantic structured networks constructed by machines? Can such machine-extracted information benefit downstream applications in various fields? The massive and messy nature of text data poses significant challenges to creating techniques for automatic processing and algorithmic analysis of contents that scale with text volume. State-of-the-art information extraction approaches rely on heavy task-specific annotations (e.g., annotating terrorist attack-related entities in web forum posts written in Arabic) to build (deep) machine learning models. In contrast, our research harnesses ``the power of massive data'' and develops a family of data-driven approaches for automatic knowledge discovery. Our methods, to alleviate the need for heavy human annotation, utilize distant supervision from existing, open knowledge bases and statistical signals (e.g., frequency and point-wise …
更多查看译文
AI 理解论文
溯源树
样例
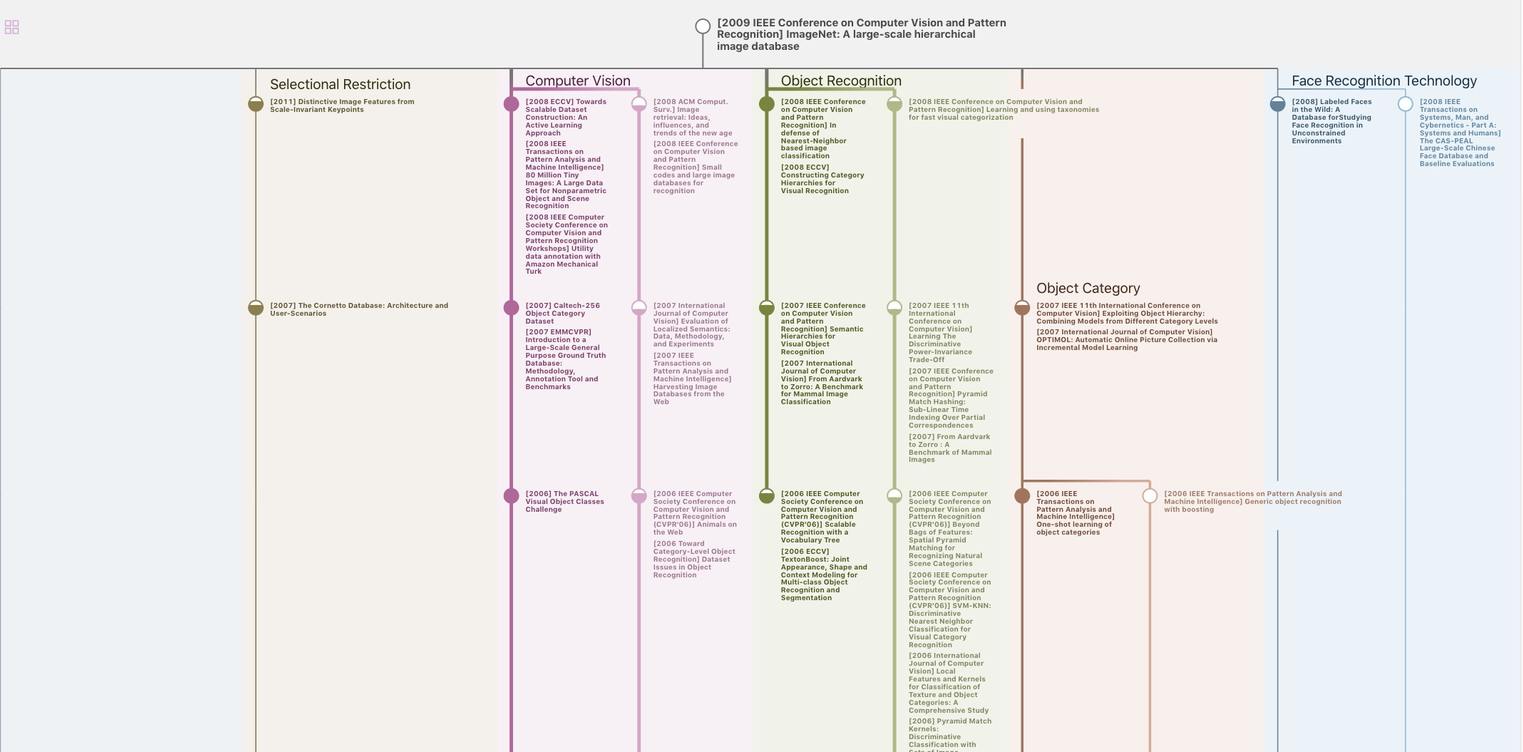
生成溯源树,研究论文发展脉络
Chat Paper
正在生成论文摘要