Nsvd: Normalized Singular Value Deviation Reveals Number Of Latent Factors In Tensor Decomposition
PROCEEDINGS OF THE 2020 SIAM INTERNATIONAL CONFERENCE ON DATA MINING (SDM)(2020)
摘要
Tensor decomposition has been shown, time and time again, to be an effective tool in multiaspect data mining, especially in exploratory applications where the interest is in discovering hidden interpretable structure from the data. In such exploratory applications, the number of such hidden structures is of utmost importance since incorrect selection may imply the discovery of noisy artifacts that do not really represent a meaningful pattern. Although extremely important, selection of this number of latent factors, also known as low-rank, is very hard, and in most cases, practitioners and researchers resort toad hoctrial-and-error or assume that somehow this number is known or is given via domain expertise. There has been a considerable amount of prior work that proposes heuristics for selecting this low rank. However, as we argue in this article, the state of the art in those heuristic methods is rather unstable and does not always reveal the correct answer. In this article, we propose the Normalized Singular Value Deviation (NSVD), a novel method for selecting the number of latent factors in Tensor Decomposition that is based on principled theoretical foundations. We extensively evaluate the effectiveness ofNSVDin synthetic and real data and demonstrate that it yields a more robust, stable, and reliable estimation than state of the art. Finally, we provide an efficient compression scheme for facilitating the use ofNSVDin very big tensors.
更多查看译文
关键词
compression, latent factor, tensor decomposition, tensor rank
AI 理解论文
溯源树
样例
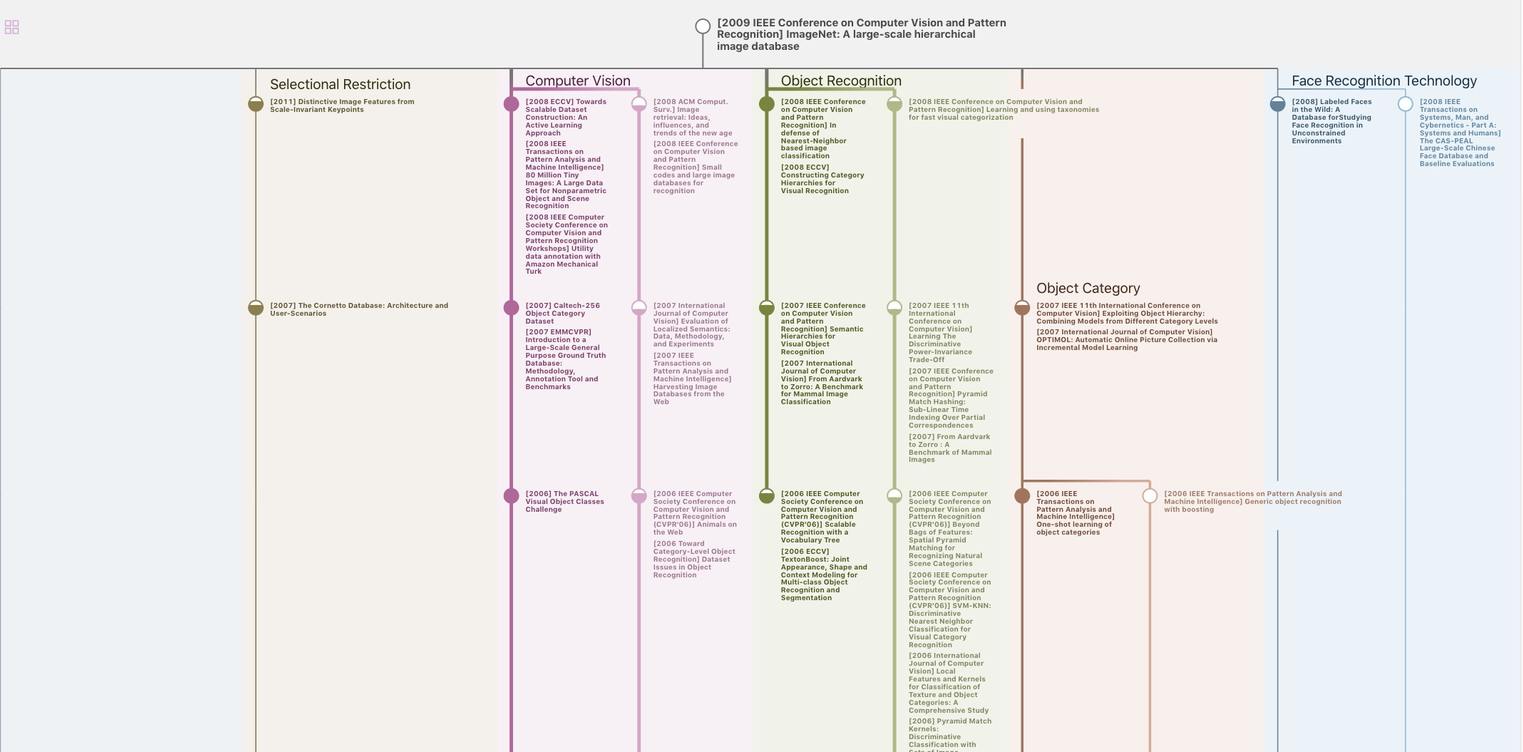
生成溯源树,研究论文发展脉络
Chat Paper
正在生成论文摘要