Ltspauc: Learning Time-Series Shapelets For Optimizing Partial Auc
PROCEEDINGS OF THE 2020 SIAM INTERNATIONAL CONFERENCE ON DATA MINING (SDM)(2020)
摘要
Shapelets are time-series segments effective for classifying time-series instances. Joint learning of both classifiers and shapelets has been studied in recent years, because such methods provide both interpretable results and superior accuracy. Partial Area Under the ROC curve (pAUC) for a low range of False Positive Rates (FPR) is an important performance measure for practical cases in industries such as medicine, manufacturing, and maintenance. In this study, we propose a method that jointly learns both shapelets and a classifier for pAUC optimization in any FPR range, including the full AUC. We demonstrate superiority of pAUC on UCR time-series datasets and its effectiveness in industrial case studies.
更多查看译文
关键词
learning,time-series
AI 理解论文
溯源树
样例
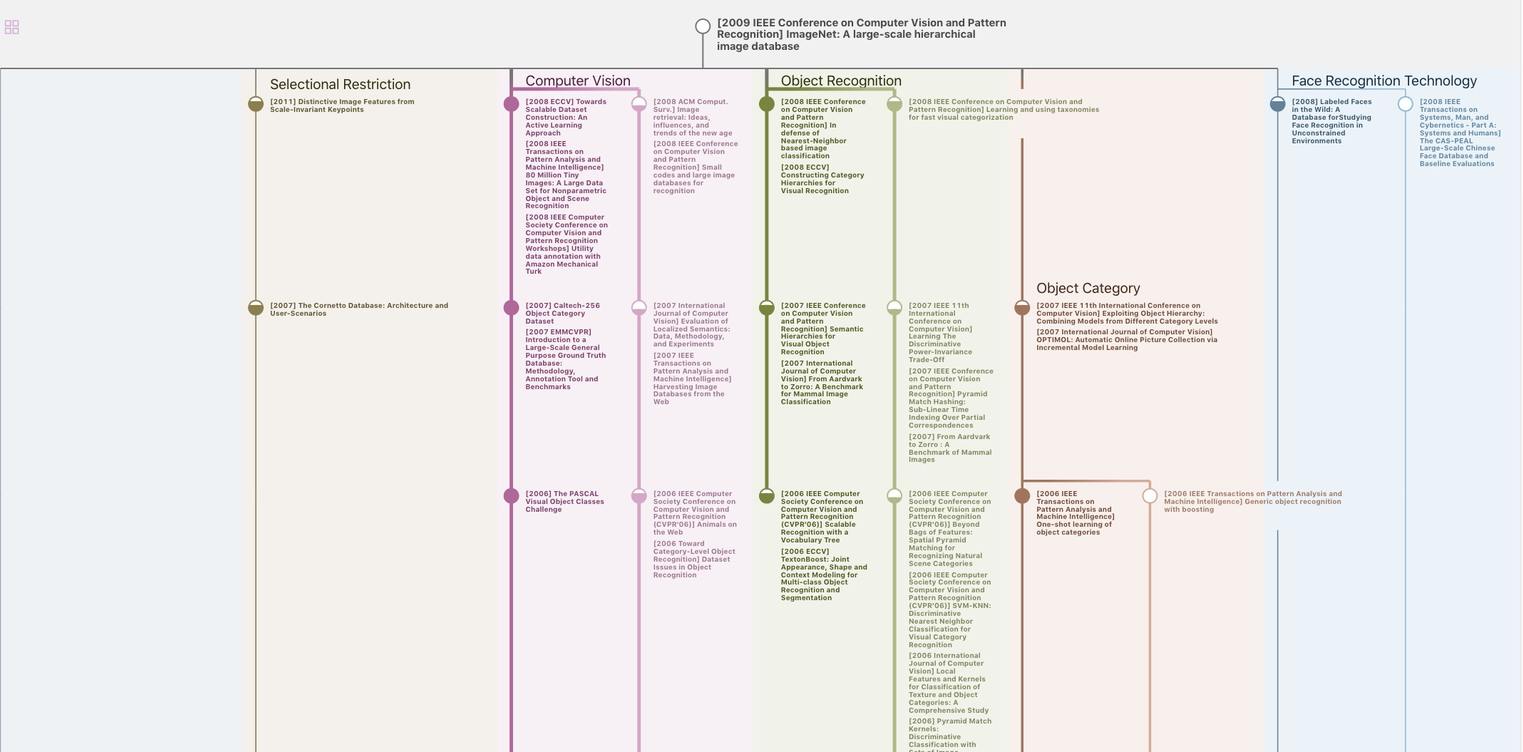
生成溯源树,研究论文发展脉络
Chat Paper
正在生成论文摘要