Parsing as Tagging.
LREC(2020)
摘要
We propose a simple yet accurate method for dependency parsing that treats parsing as tagging (PaT). That is, our approach addresses the parsing of dependency trees with a sequence model implemented with a bidirectional LSTM over BERT embeddings, where the "tag" to be predicted at each token position is the relative position of the corresponding head. For example, for the sentence John eats cake, the tag to be predicted for the token cake is -1 because its head (eats) occurs one token to the left. Despite its simplicity, our approach performs well. For example, our approach outperforms the state-of-the-art method of (Fernandez-Gonzalez and Gomez-Rodriguez, 2019) on Universal Dependencies (UD) by 1.76% unlabeled attachment score (UAS) for English, 1.98% UAS for French, and 1.16% UAS for German. On average, on 15 UD languages, our method with minimal tuning performs comparably with this state-of-the-art approach, being only 0.16% UAS, and 0.82% LAS behind.
更多查看译文
关键词
dependency parsing, sequence methods
AI 理解论文
溯源树
样例
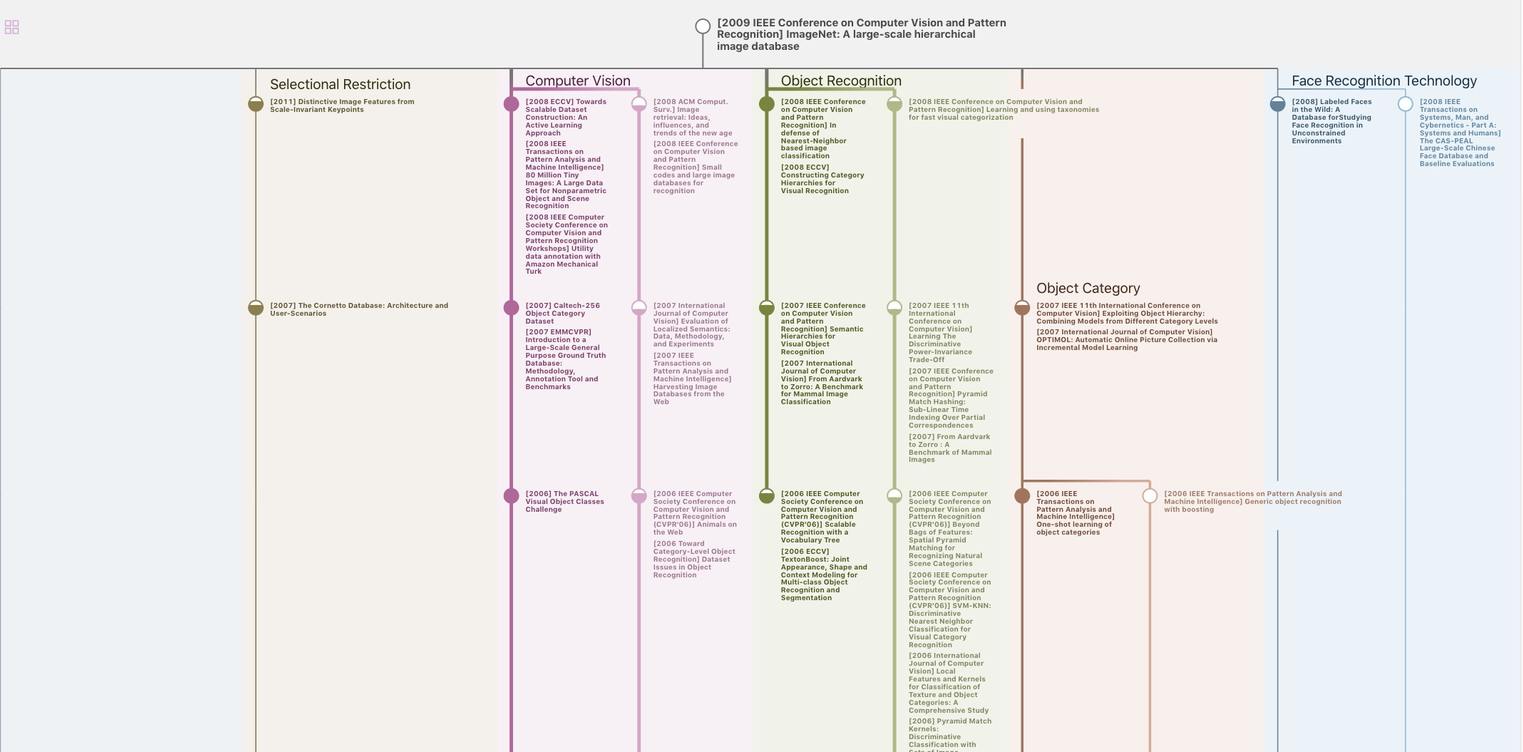
生成溯源树,研究论文发展脉络
Chat Paper
正在生成论文摘要