Long Range Sequence Generation via Multiresolution Adversarial Training
user-5ebe28934c775eda72abcddd(2018)
摘要
We present a multi-resolution approach for generative modeling of spatiotemporal sequences. Our approach focuses on a key challenge of modeling long-term temporal dependencies that is common in complex spatiotemporal phenomena. For instance, realistic modeling of basketball players behavior requires capturing long-term goals and how they influence short-term decisions. Our multi-resolution approach has several attractive properties. First, it is completely unsupervised, and requires no additional labeling of high-level semantics such as long-term goals. Second, the multi-resolution aspect allows us to model conditional distributions beyond forward sampling, such as conditioning on future behavior. Finally, our approach integrates generative adversarial training, which enables us to learn generative models that significantly outperform conventional generative sequence modeling. We validate the effectiveness of our model on synthetic sequences and spatiotemporal basketball player trajectory generation.
更多查看译文
AI 理解论文
溯源树
样例
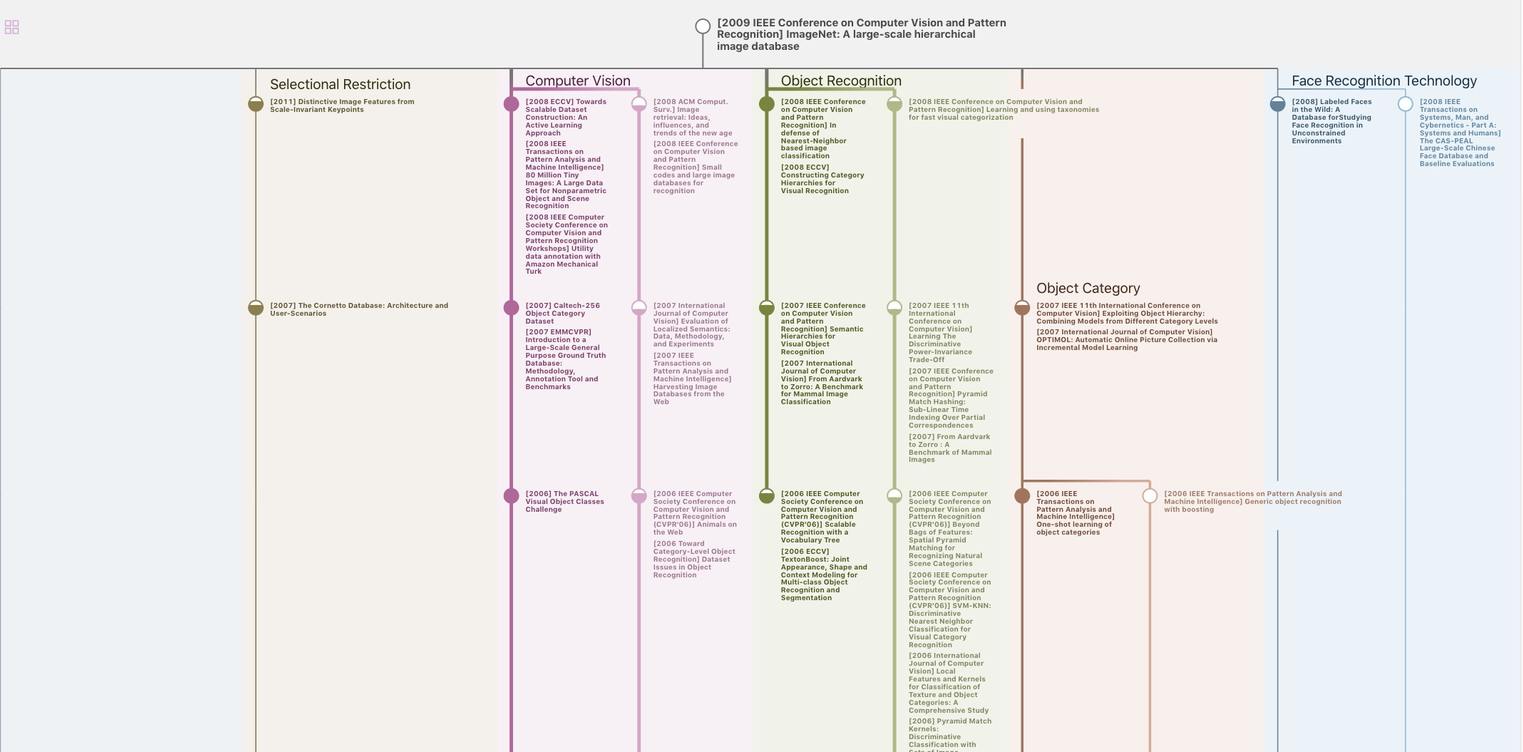
生成溯源树,研究论文发展脉络
Chat Paper
正在生成论文摘要