Assisted Learning and Imitation Privacy
arxiv(2020)
摘要
Motivated by the emerging needs of decentralized learners with personalized learning objectives, we present an Assisted Learning framework where a service provider Bob assists a learner Alice with supervised learning tasks without transmitting Bob's private algorithm or data. Bob assists Alice either by building a predictive model using Alice's labels, or by improving Alice's private learning through iterative communications where only relevant statistics are transmitted. The proposed learning framework is naturally suitable for distributed, personalized, and privacy-aware scenarios. For example, it is shown in some scenarios that two suboptimal learners could achieve much better performance through Assisted Learning. Moreover, motivated by privacy concerns in Assisted Learning, we present a new notion of privacy to quantify the privacy leakage at learning level instead of data level. This new privacy, named imitation privacy, is particularly suitable for a market of statistical learners each holding private learning algorithms as well as data.
更多查看译文
关键词
assisted learning,privacy
AI 理解论文
溯源树
样例
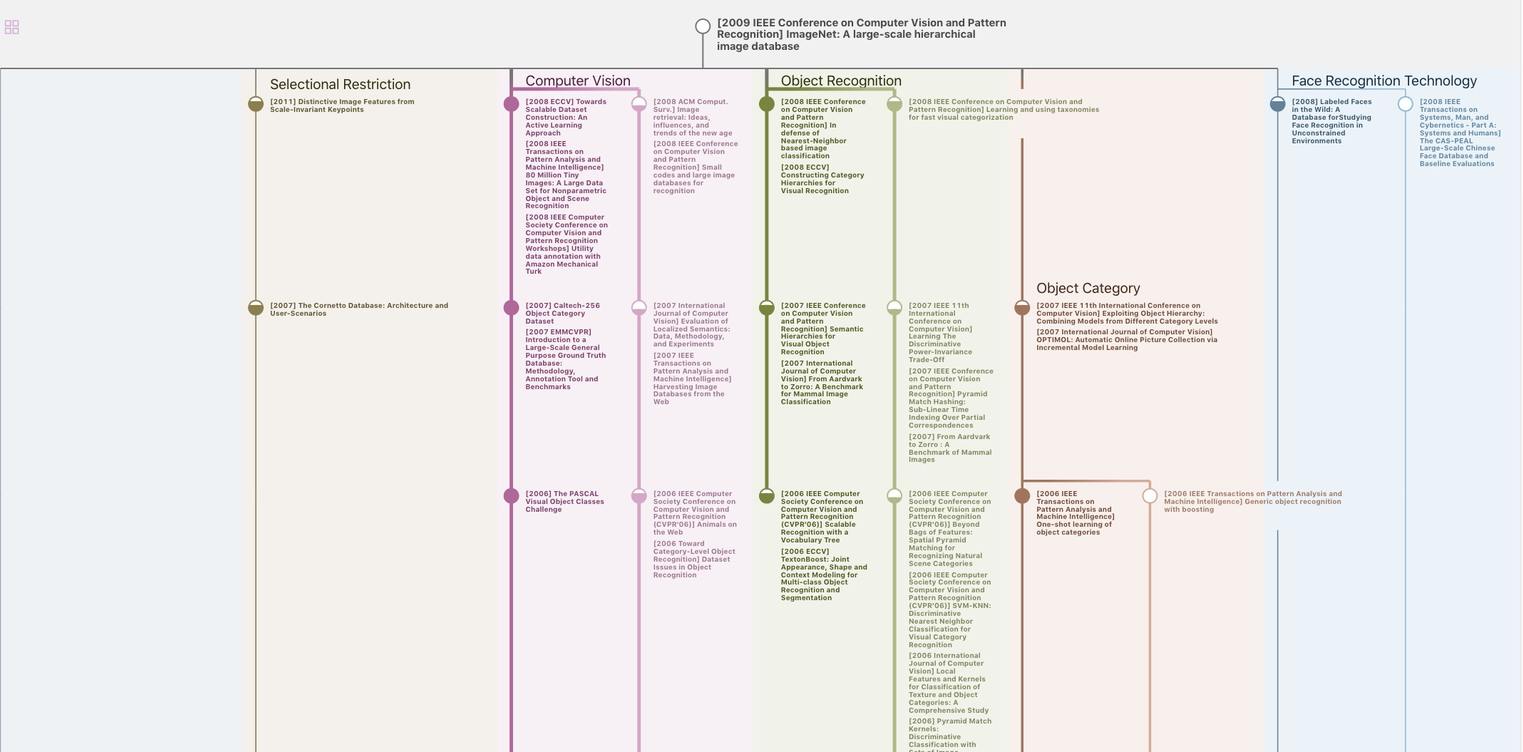
生成溯源树,研究论文发展脉络
Chat Paper
正在生成论文摘要