Meta Clustering for Collaborative Learning
arxiv(2023)
摘要
In collaborative learning, learners coordinate to enhance each of their learning performances. From the perspective of any learner, a critical challenge is to filter out unqualified collaborators. We propose a framework named meta clustering to address the challenge. Unlike the classical problem of clustering data points, meta clustering categorizes learners. Assuming each learner performs a supervised regression on a standalone local dataset, we propose a Select-Exchange-Cluster (SEC) method to classify the learners by their underlying supervised functions. We theoretically show that the SEC can cluster learners into accurate collaboration sets. Empirical studies corroborate the theoretical analysis and demonstrate that SEC can be computationally efficient, robust against learner heterogeneity, and effective in enhancing single-learner performance. Also, we show how the proposed approach may be used to enhance data fairness. Supplementary materials for this article are available online.
更多查看译文
关键词
Data integration,Distributed computing,Fairness,Meta clustering,Regression
AI 理解论文
溯源树
样例
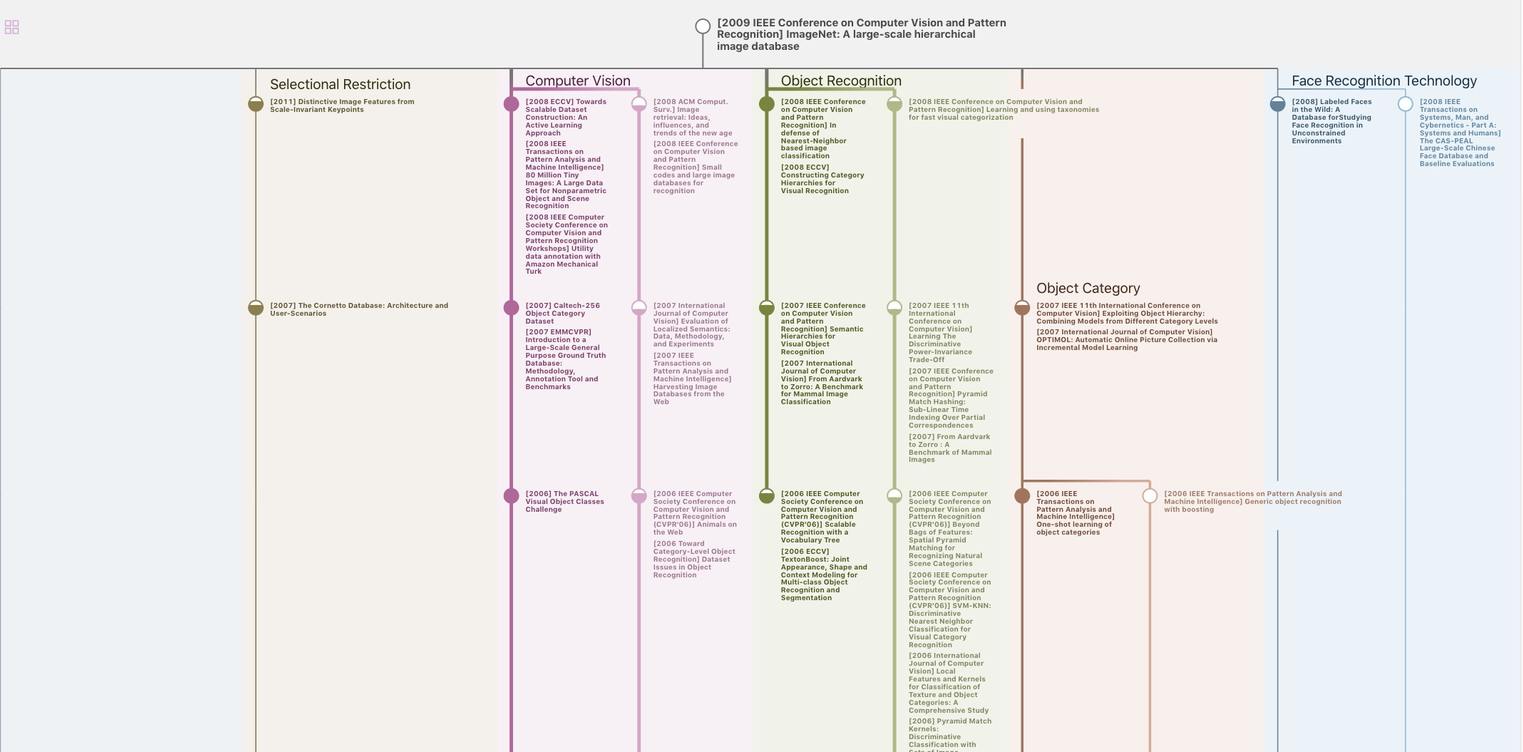
生成溯源树,研究论文发展脉络
Chat Paper
正在生成论文摘要