On Regularizability and Its Application to Online Control of Unstable LTI Systems
IEEE Transactions on Automatic Control(2022)
摘要
Learning, say through direct policy updates, often requires assumptions such as knowing
a priori
that the initial policy (gain) is stabilizing, or persistently exciting (PE) input–output data, is available. In this article, we examine online regulation of (possibly unstable) partially unknown linear systems with no prior access to an initial stabilizing controller nor PE input–output data; we instead leverage the knowledge of the input matrix for online regulation. First, we introduce and characterize the notion of “regularizability” for linear systems that gauges the extent by which a system can be regulated in finite-time in contrast to its asymptotic behavior (commonly characterized by stabilizability/controllability). Next, having access only to the input matrix, we propose the data-guided regulation (DGR) synthesis procedure that—as its name suggests—regulates the underlying state while also generating informative data that can subsequently be used for data-driven stabilization or system identification. We further improve the computational performance of DGR via a rank-one update and demonstrate its utility in online regulation of the X-29 aircraft.
更多查看译文
关键词
Iterative control,online regulation,single trajectory learning,unstable linear systems
AI 理解论文
溯源树
样例
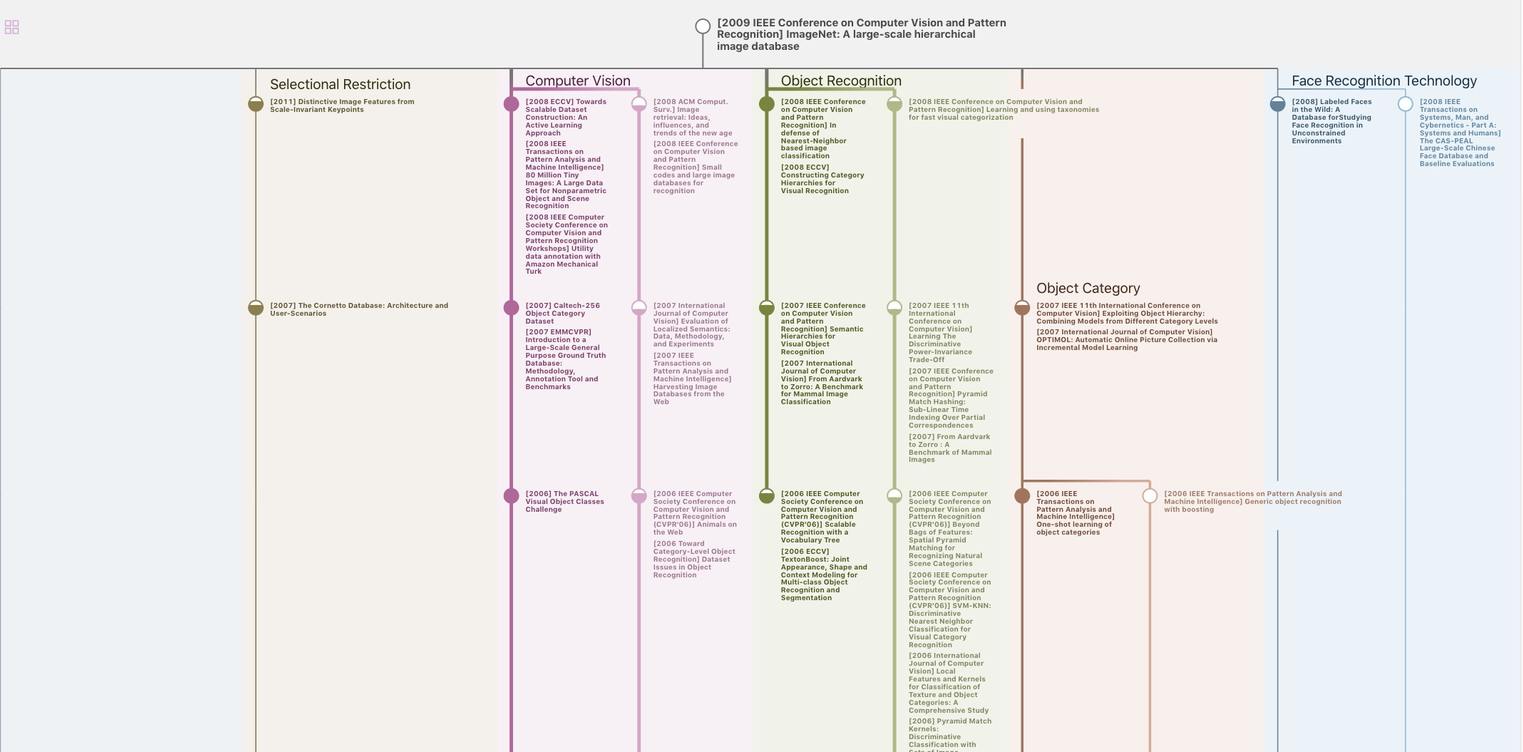
生成溯源树,研究论文发展脉络
Chat Paper
正在生成论文摘要