Transferring Inductive Biases through Knowledge Distillation
arxiv(2020)
摘要
Having the right inductive biases can be crucial in many tasks or scenarios where data or computing resources are a limiting factor, or where training data is not perfectly representative of the conditions at test time. However, defining, designing and efficiently adapting inductive biases is not necessarily straightforward. In this paper, we explore the power of knowledge distillation for transferring the effect of inductive biases from one model to another. We consider families of models with different inductive biases, LSTMs vs. Transformers and CNNs vs. MLPs, in the context of tasks and scenarios where having the right inductive biases is critical. We study how the effect of inductive biases is transferred through knowledge distillation, in terms of not only performance but also different aspects of converged solutions.
更多查看译文
关键词
inductive biases,knowledge
AI 理解论文
溯源树
样例
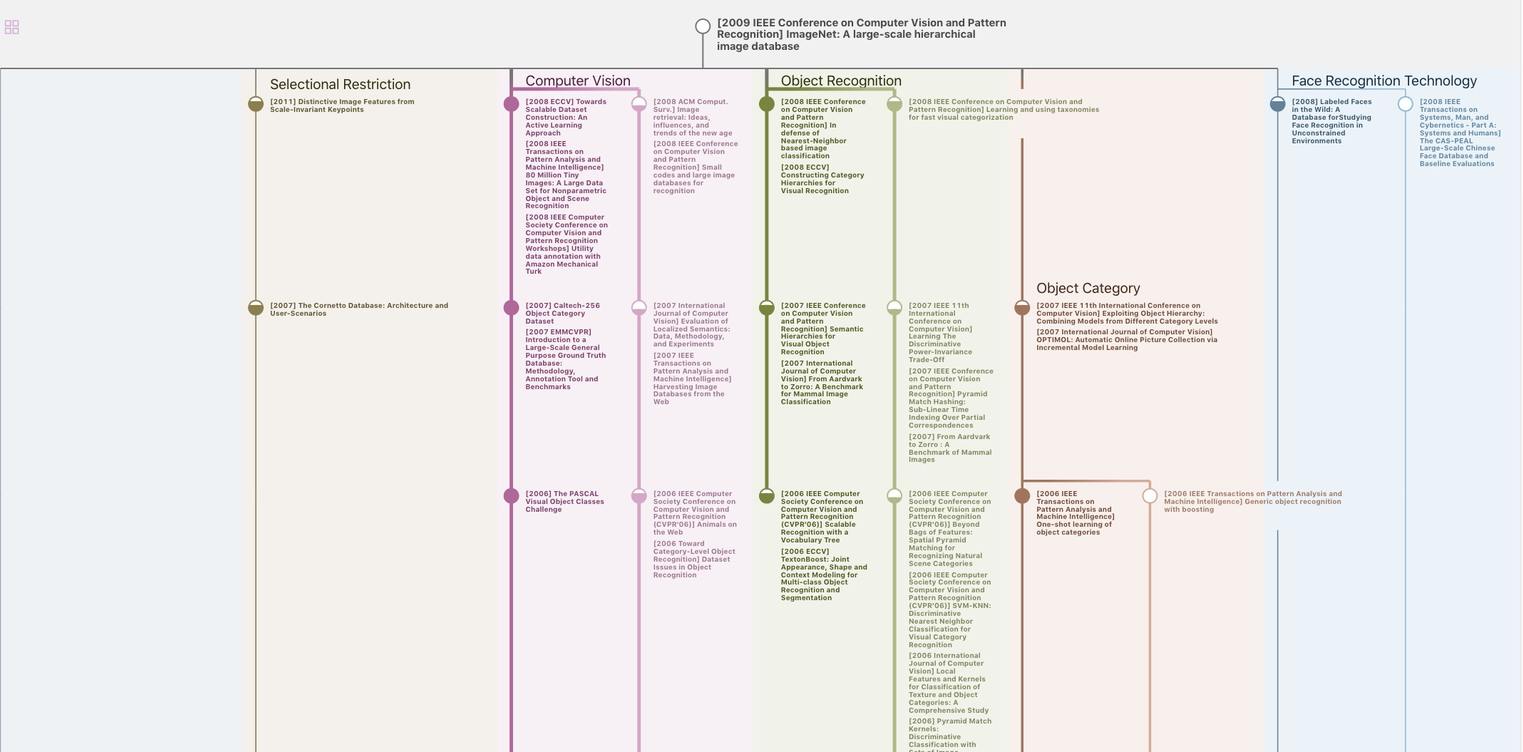
生成溯源树,研究论文发展脉络
Chat Paper
正在生成论文摘要