Provable Guarantees for Decision Tree Induction: The Agnostic Setting
ICML(2020)
摘要
We give strengthened provable guarantees on the performance of widely employed and empirically successful top-down decision tree learning heuristics. While prior works have focused on the realizable setting, we consider the more realistic and challenging agnostic setting. We show that for all monotone functions f and s is an element of IN, these heuristics construct a decision tree of size s((O) over tilde((log s)/epsilon 2)) that achieves error <= opt(s) + epsilon, where opt s denotes the error of the optimal size-s decision tree for f . Previously such a guarantee was not known to be achievable by any algorithm, even one that is not based on top-down heuristics. We complement our algorithmic guarantee with a near-matching s S-(Omega) over tilde (logs) lower bound.
更多查看译文
关键词
decision tree induction,provable guarantees
AI 理解论文
溯源树
样例
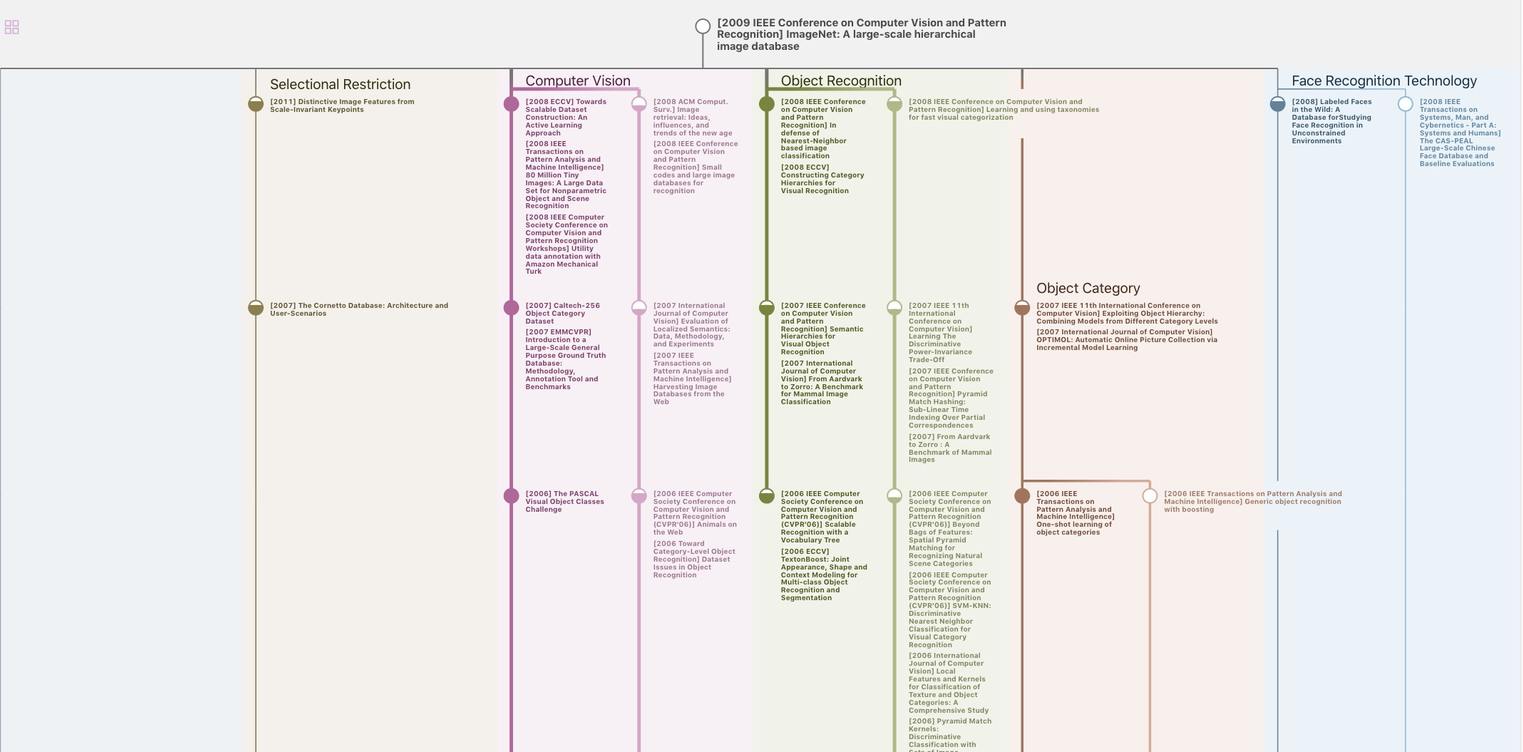
生成溯源树,研究论文发展脉络
Chat Paper
正在生成论文摘要