A Machine Learning Approach to Identifying Changes in Suicidal Language.
SUICIDE AND LIFE-THREATENING BEHAVIOR(2020)
摘要
Objective With early identification and intervention, many suicidal deaths are preventable. Tools that include machine learning methods have been able to identify suicidal language. This paper examines the persistence of this suicidal language up to 30 days after discharge from care. Method In a multi-center study, 253 subjects were enrolled into either suicidal or control cohorts. Their responses to standardized instruments and interviews were analyzed using machine learning algorithms. Subjects were re-interviewed approximately 30 days later, and their language was compared to the original language to determine the presence of suicidal ideation. Results The results show that language characteristics used to classify suicidality at the initial encounter are still present in the speech 30 days later (AUC = 89% (95% CI: 85-95%), p .0001) and that algorithms trained on the second interviews could also identify the subjects that produced the first interviews (AUC = 85% (95% CI: 81-90%), p .0001). Conclusions This approach explores the stability of suicidal language. When using advanced computational methods, the results show that a patient's language is similar 30 days after first captured, while responses to standard measures change. This can be useful when developing methods that identify the data-based phenotype of a subject.
更多查看译文
AI 理解论文
溯源树
样例
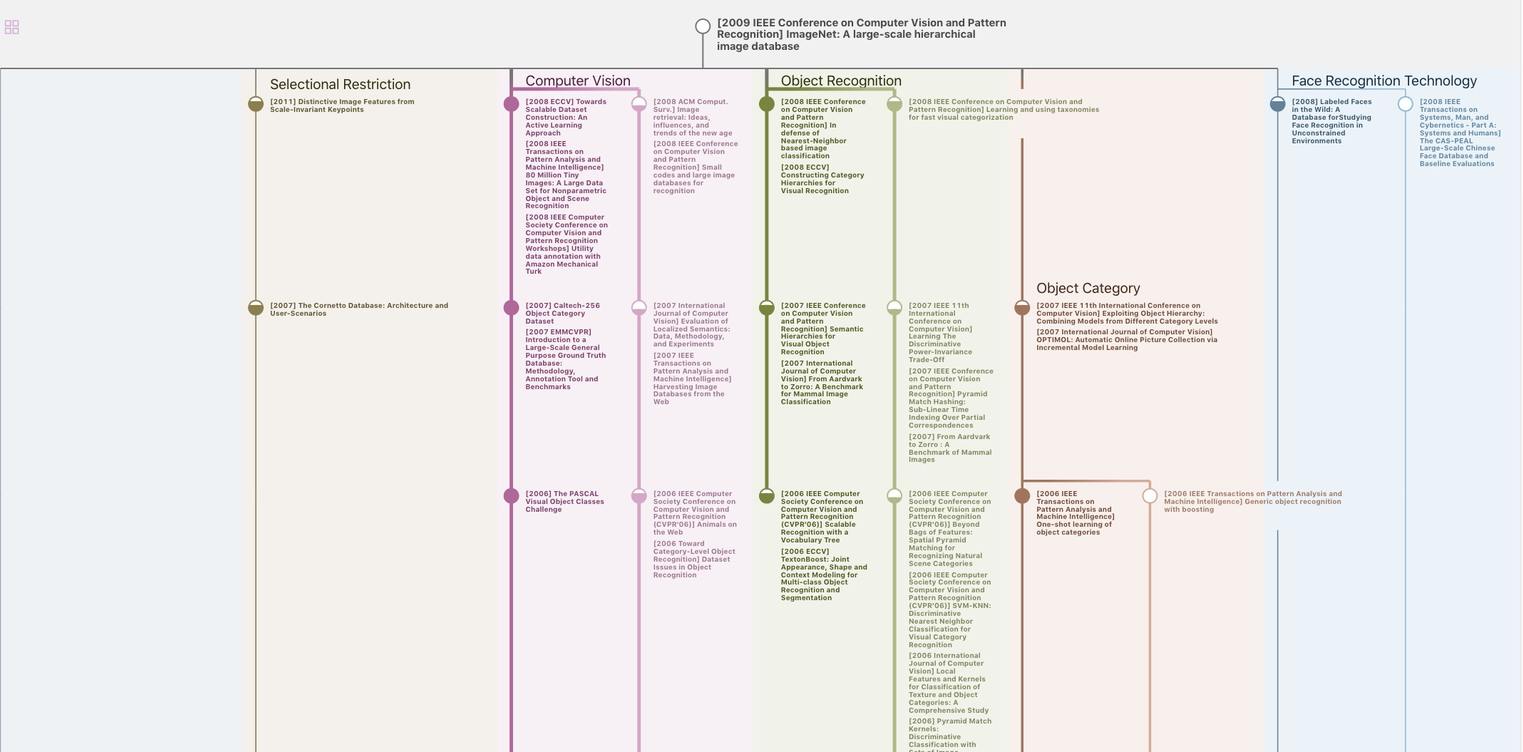
生成溯源树,研究论文发展脉络
Chat Paper
正在生成论文摘要