Non-intrusive Identification of Student Attentiveness and Finding Their Correlation with Detectable Facial Emotions
ACM Southeast Regional Conference(2020)
摘要
ABSTRACTTeachers use observational cues in the classroom to identify attentiveness of students and guide the pace of their lecture. However, effectiveness of this technique decreases with increasing class size. This paper presented an approach for automating these observational cues from the students' facial expressions and identifying their attentiveness via a neural network machine learning model. Results of the deep learning Convolutional Neural Network model were then compared with the range of confidence values obtained from a cloud-based emotion recognition service to identify the correlations with human observer. Real time videos of students were collected during classes and sample dataset were created for attentive and inattentive students in order to train the machine learning model. This system can be highly useful for inexperienced teachers for early identification of inattentive students in classroom and thereby taking necessary actions to enhance student learning.
更多查看译文
关键词
Classroom Observational Study, Machine Learning, Convolutional Neural Network, Deep Learning, Amazon Rekognition, Facial Emotion Detection
AI 理解论文
溯源树
样例
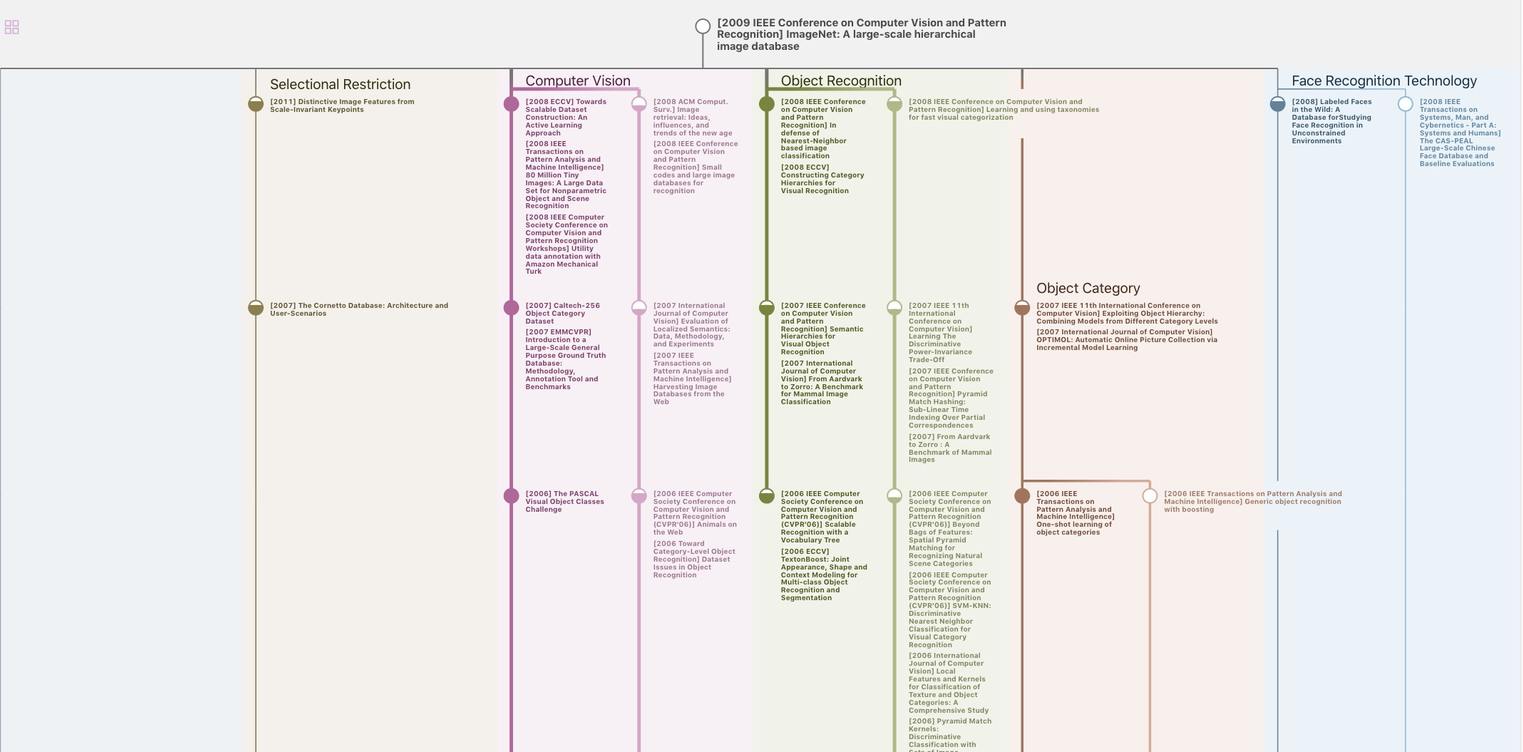
生成溯源树,研究论文发展脉络
Chat Paper
正在生成论文摘要