Learning Active Task-Oriented Exploration Policies For Bridging The Sim-To-Real Gap
ROBOTICS: SCIENCE AND SYSTEMS XVI(2020)
摘要
Training robotic policies in simulation suffers from the sim-to-real gap, as simulated dynamics can be different from real-world dynamics. Past works tackled this problem through domain randomization and online system-identification. The former is sensitive to the manually-specified training distribution of dynamics parameters and can result in behaviors that are overly conservative. The latter requires learning policies that concurrently perform the task and generate useful trajectories for system identification. In this work, we propose and analyze a framework for learning exploration policies that explicitly perform task-oriented exploration actions to identify task-relevant system parameters. These parameters are then used by model based trajectory optimization algorithms to perform the task in the real world. We instantiate the framework in simulation with the Linear Quadratic Regulator as well as in the real world with pouring and object dragging tasks. Experiments show that task oriented exploration helps model-based policies adapt to systems with initially unknown parameters, and it leads to better task performance than task-agnostic exploration.
更多查看译文
关键词
exploration policies,task-oriented,sim-to-real
AI 理解论文
溯源树
样例
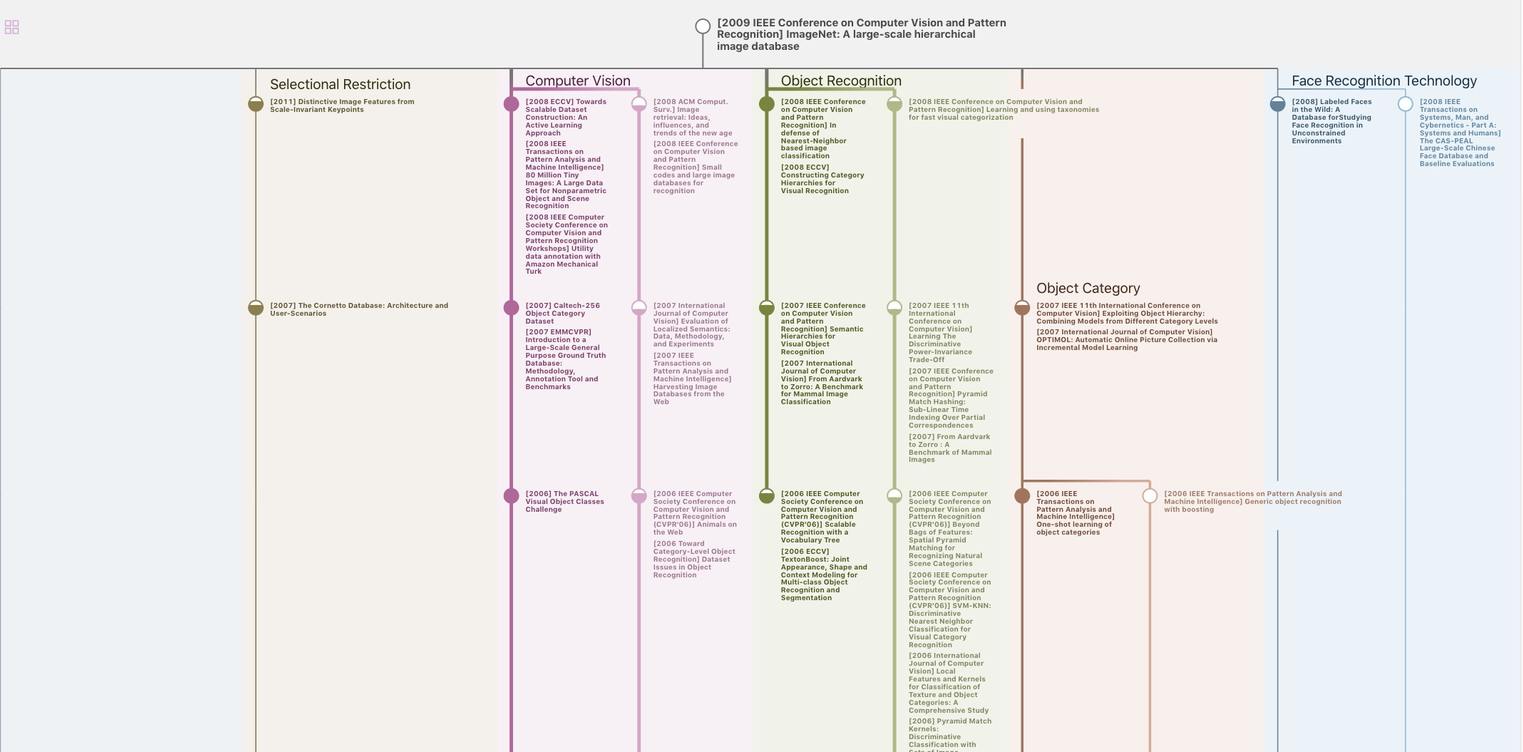
生成溯源树,研究论文发展脉络
Chat Paper
正在生成论文摘要