Dynamic System Identification of Underwater Vehicles Using Multi-Output Gaussian Processes
INTERNATIONAL JOURNAL OF AUTOMATION AND COMPUTING(2021)
摘要
Non-parametric system identification with Gaussian processes for underwater vehicles is explored in this research with the purpose of modelling autonomous underwater vehicle (AUV) dynamics with a low amount of data. Multi-output Gaussian processes and their aptitude for modelling the dynamic system of an underactuated AUV without losing the relationships between tied outputs are used. The simulation of a first-principle model of a Remus 100 AUV is employed to capture data for the training and validation of the multi-output Gaussian processes. The metric and required procedure to carry out multi-output Gaussian processes for AUV with 6 degrees of freedom (DoF) is also shown in this paper. Multi-output Gaussian processes compared with the popular technique of recurrent neural network show that multi-output Gaussian processes manage to surpass RNN for non-parametric dynamic system identification in underwater vehicles with highly coupled DoF with the added benefit of providing the measurement of confidence.
更多查看译文
关键词
Dependent Gaussian processes, dynamic system identification, multi-output Gaussian processes, non-parametric identification, autonomous underwater vehicle (AUV)
AI 理解论文
溯源树
样例
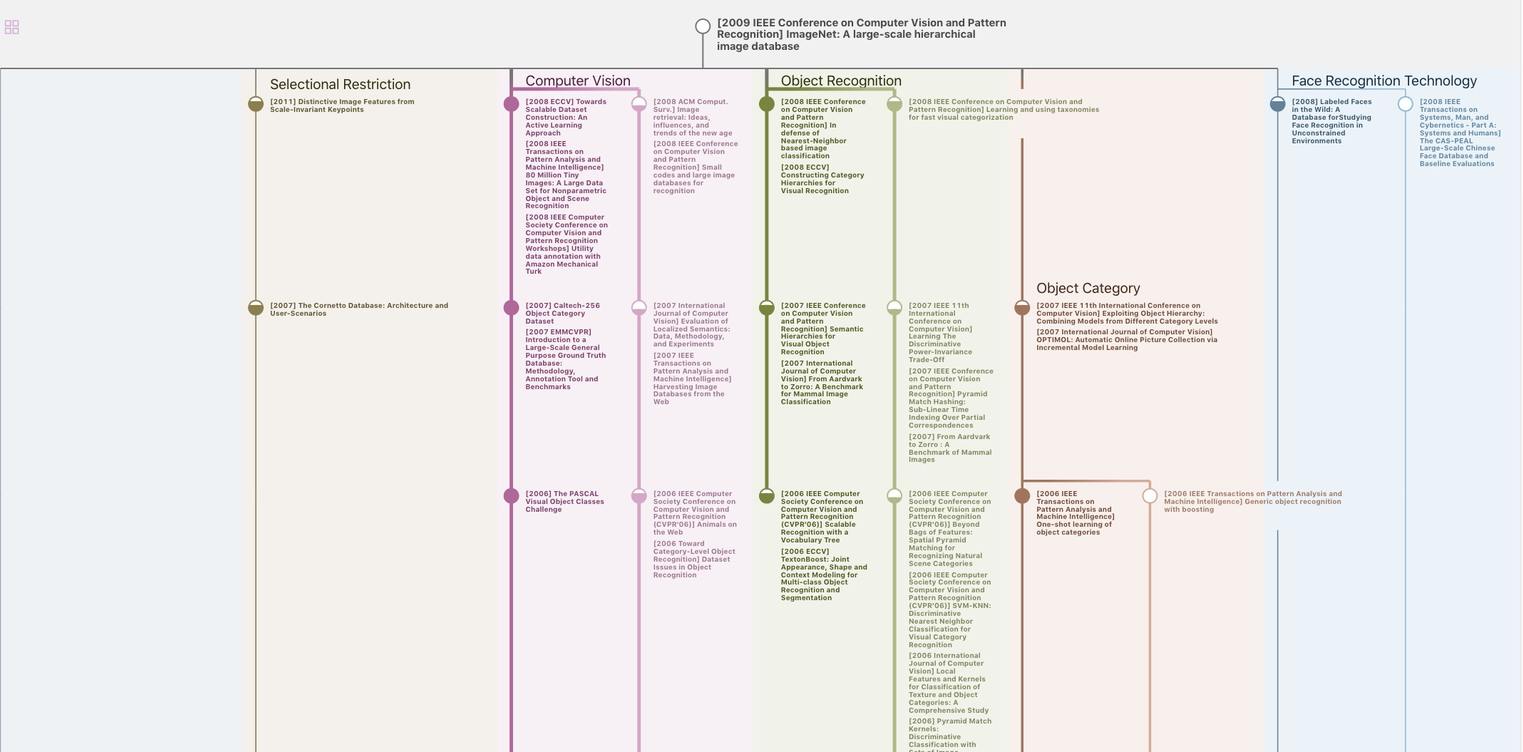
生成溯源树,研究论文发展脉络
Chat Paper
正在生成论文摘要