Metaheuristic macro scale traffic flow optimisation from urban movement data
arxiv(2020)
摘要
How can urban movement data be exploited in order to improve the flow of traffic within a city? Movement data provides valuable information about routes and specific roads that people are likely to drive on. This allows us to pinpoint roads that occur in many routes and are thus sensitive to congestion. Redistributing some of the traffic to avoid unnecessary use of these roads could be a key factor in improving traffic flow. Many proposed approaches to combat congestion are either static or do not incorporate any movement data. In this work, we present a method to redistribute traffic through the introduction of externally imposed variable costs to each road segment, assuming that all drivers seek to drive the cheapest route. We use a metaheuristic optimisation approach to minimise total travel times by optimising a set of road-specific variable cost parameters, which are used as input for an objective function based on traffic flow theory. The optimisation scenario for the city centre of Tokyo considered in this paper was defined using public spatial road network data, and movement data acquired from Foursquare. Experimental results show that our proposed scenario has the potential to achieve a 62.6\% improvement of total travel time in Tokyo compared to that of a currently operational road network configuration, with no imposed variable costs.
更多查看译文
关键词
traffic flow,urban movement data,optimisation
AI 理解论文
溯源树
样例
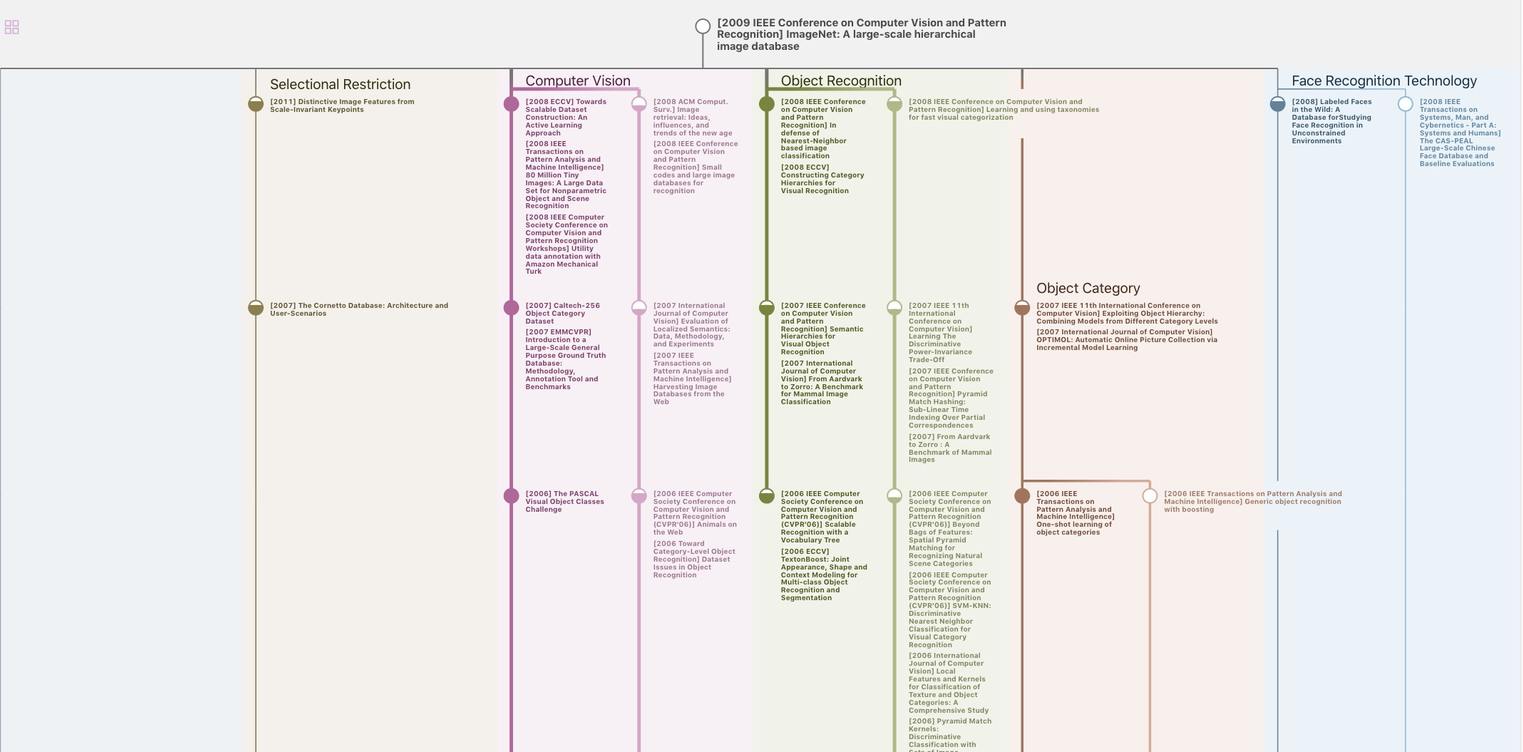
生成溯源树,研究论文发展脉络
Chat Paper
正在生成论文摘要