Empirical Properties of Optima in Free Semidefinite Programs.
Experimental mathematics(2021)
摘要
Semidefinite programming is based on optimization of linear functionals over convex sets defined by linear matrix inequalities, namely, inequalities of the form L-A(X)=I-A(1)X(1)- horizontal ellipsis -A(g)X(g) >= 0. Here, the X-j are real numbers and the set of solutions is called a spectrahedron. These inequalities make sense when the X-i are symmetric matrices of any size, n x n, and enter the formula though tensor product Ai circle times Xi: The solution set of LA(X)>= 0 is called a free spectrahedron since it contains matrices of all sizes and the defining "linear pencil" is "free" of the sizes of the matrices. In this article, we report on empirically observed properties of optimizers obtained from optimizing linear functionals over free spectrahedra restricted to matrices X-i of fixed size n x n. The optimizers we find are always classical extreme points. Surprisingly, in many reasonable parameter ranges, over 99.9% are also free extreme points. Moreover, the dimension of the active constraint, ker(L-A(X-l)), is about twice what we expected. Another distinctive pattern regards reducibility of optimizing tuples (X-1(l), ... ,X-g(l)). We give an algorithm for representing elements of a free spectrahedron as matrix convex combinations of free extreme points; these representations satisfy a very low bound on the number of free extreme points needed.
更多查看译文
关键词
Matrix convex set,extreme point,linear matrix inequality,spectrahedron,Arveson boundary,free semidefinite programming
AI 理解论文
溯源树
样例
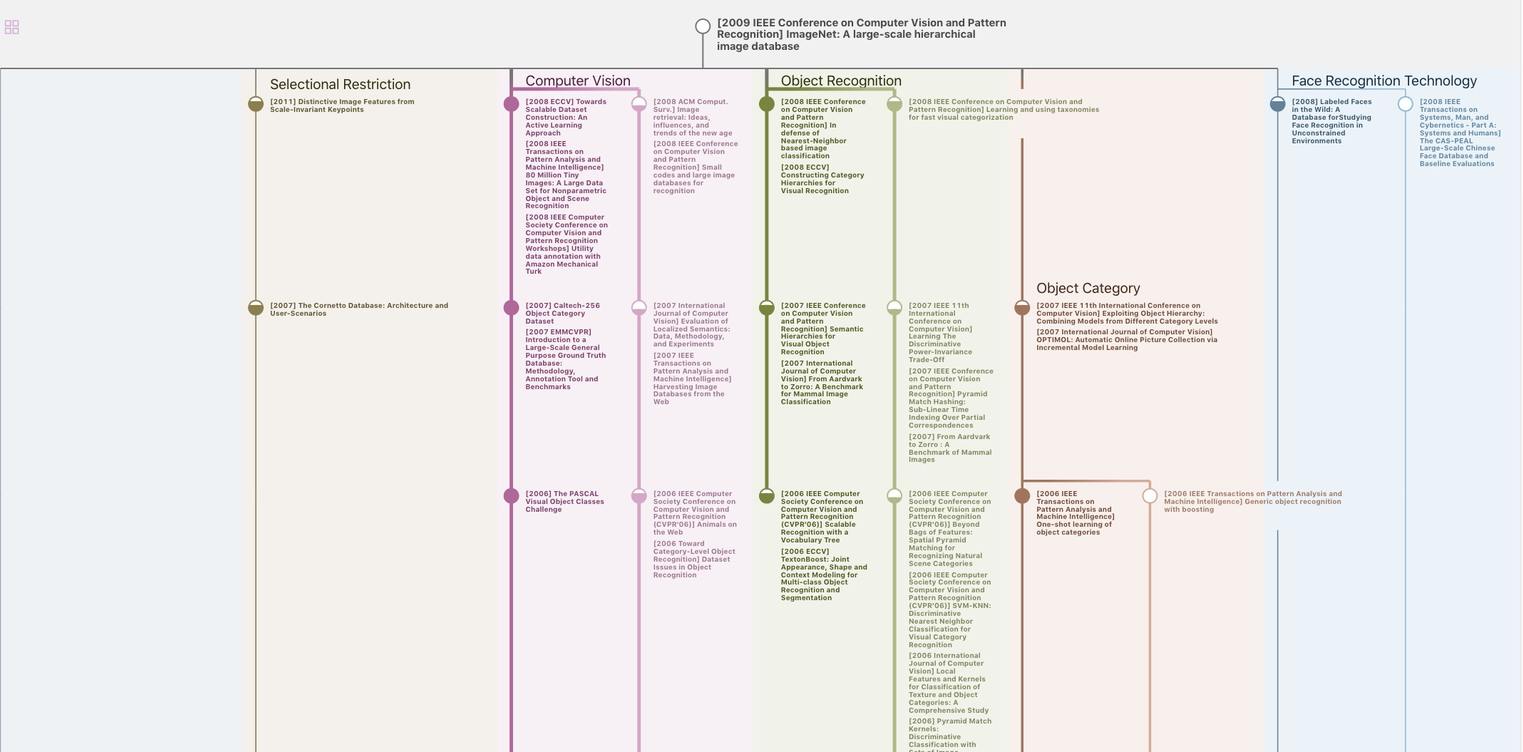
生成溯源树,研究论文发展脉络
Chat Paper
正在生成论文摘要