Learning across label confidence distributions using Filtered Transfer Learning
ICMLA(2020)
摘要
Performance of neural network models relies on the availability of large datasets with minimal levels of uncertainty. Transfer Learning (TL) models have been proposed to resolve the issue of small dataset size by letting the model train on a bigger, task-related reference dataset and then fine-tune on a smaller, task-specific dataset. In this work, we apply a transfer learning approach to improve predictive power in noisy data systems with large variable confidence datasets. We propose a deep neural network method called Filtered Transfer Learning (FTL) that defines multiple tiers of data confidence as separate tasks in a transfer learning setting. The deep neural network is fine-tuned in a hierarchical process by iteratively removing (filtering) data points with lower label confidence, and retraining. In this report we use FTL for predicting the interaction of drugs and proteins. We demonstrate that using FTL to learn stepwise, across the label confidence distribution, results in higher performance compared to deep neural network models trained on a single confidence range. We anticipate that this approach will enable the machine learning community to benefit from large datasets with uncertain labels in fields such as biology and medicine.
更多查看译文
关键词
label confidence distributions,learning,transfer
AI 理解论文
溯源树
样例
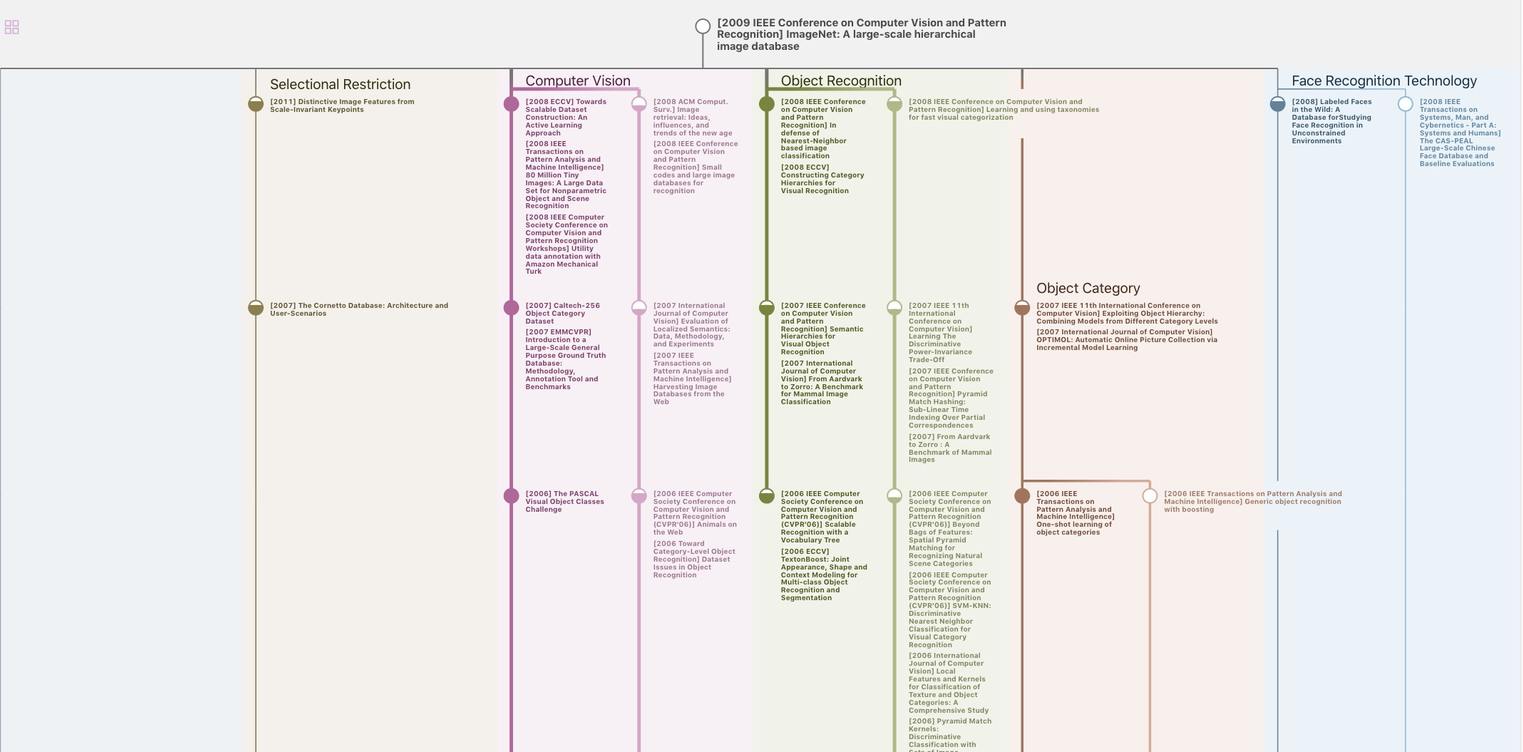
生成溯源树,研究论文发展脉络
Chat Paper
正在生成论文摘要