Meta-Model-Based Meta-Policy Optimization.
ACML(2021)
摘要
Model-based reinforcement learning (MBRL) has been applied to meta-learning settings and demonstrated its high sample efficiency. However, in previous MBRL for meta-learning settings, policies are optimized via rollouts that fully rely on a predictive model for an environment, and thus its performance in a real environment tends to degrade when the predictive model is inaccurate. In this paper, we prove that the performance degradation can be suppressed by using branched meta-rollouts. Based on this theoretical analysis, we propose meta-model-based meta-policy optimization (M3PO), in which the branched meta-rollouts are used for policy optimization. We demonstrate that M3PO outperforms existing meta reinforcement learning methods in continuous-control benchmarks.
更多查看译文
关键词
optimization,meta-model-based,meta-policy
AI 理解论文
溯源树
样例
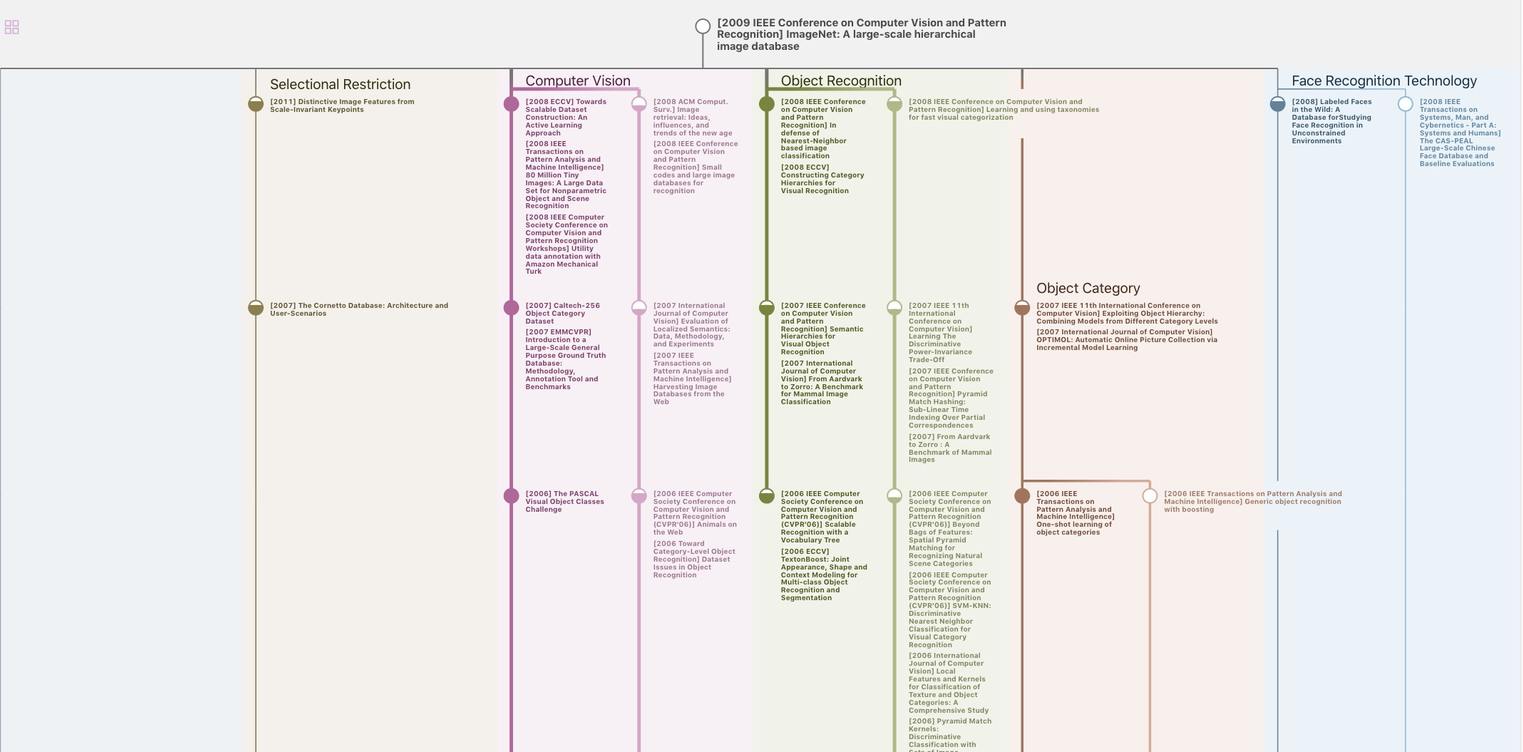
生成溯源树,研究论文发展脉络
Chat Paper
正在生成论文摘要