Conditional Safety Margins For Less Conservative Peak Local Sar Assessment: A Probabilistic Approach
MAGNETIC RESONANCE IN MEDICINE(2020)
摘要
Purpose The introduction of a linear safety factor to address peak local specific absorption rate (pSAR(10g)) uncertainties (eg, intersubject variation, modeling inaccuracies) bears one considerable drawback: It often results in over-conservative scanning constraints. We present a more efficient approach to define a variable safety margin based on the conditional probability density function of the effectively obtained pSAR(10g) value, given the estimated pSAR(10g) value.Methods The conditional probability density function can be estimated from previously simulated data. A representative set of true and estimated pSAR(10g) samples was generated by means of our database of 23 subject-specific models with an 8-fractionated dipole array for prostate imaging at 7 T. The conditional probability density function was calculated for each possible estimated pSAR(10g) value and used to determine the corresponding safety margin with an arbitrary low probability of underestimation. This approach was applied to five state-of-the-art local SAR estimation methods, namely: (1) using just the generic body model "Duke"; (2) using our model library to assess the maximum pSAR(10g) value over all models; (3) using the most representative "local SAR model"; (4) using the five most representative local SAR models; and (5) using a recently developed deep learning-based method.Results Compared with the more conventional safety factor, the conditional safety-margin approach results in lower (up to 30%) mean overestimation for all investigated local SAR estimation methods.Conclusion The proposed probabilistic approach for pSAR(10g) correction allows more accurate local SAR assessment with much lower overestimation, while a predefined level of underestimation is accepted (eg, 0.1%).
更多查看译文
关键词
parallel transmit, safety factor, SAR model library, SAR model selection, specific absorption rate, subject-specific local SAR assessment
AI 理解论文
溯源树
样例
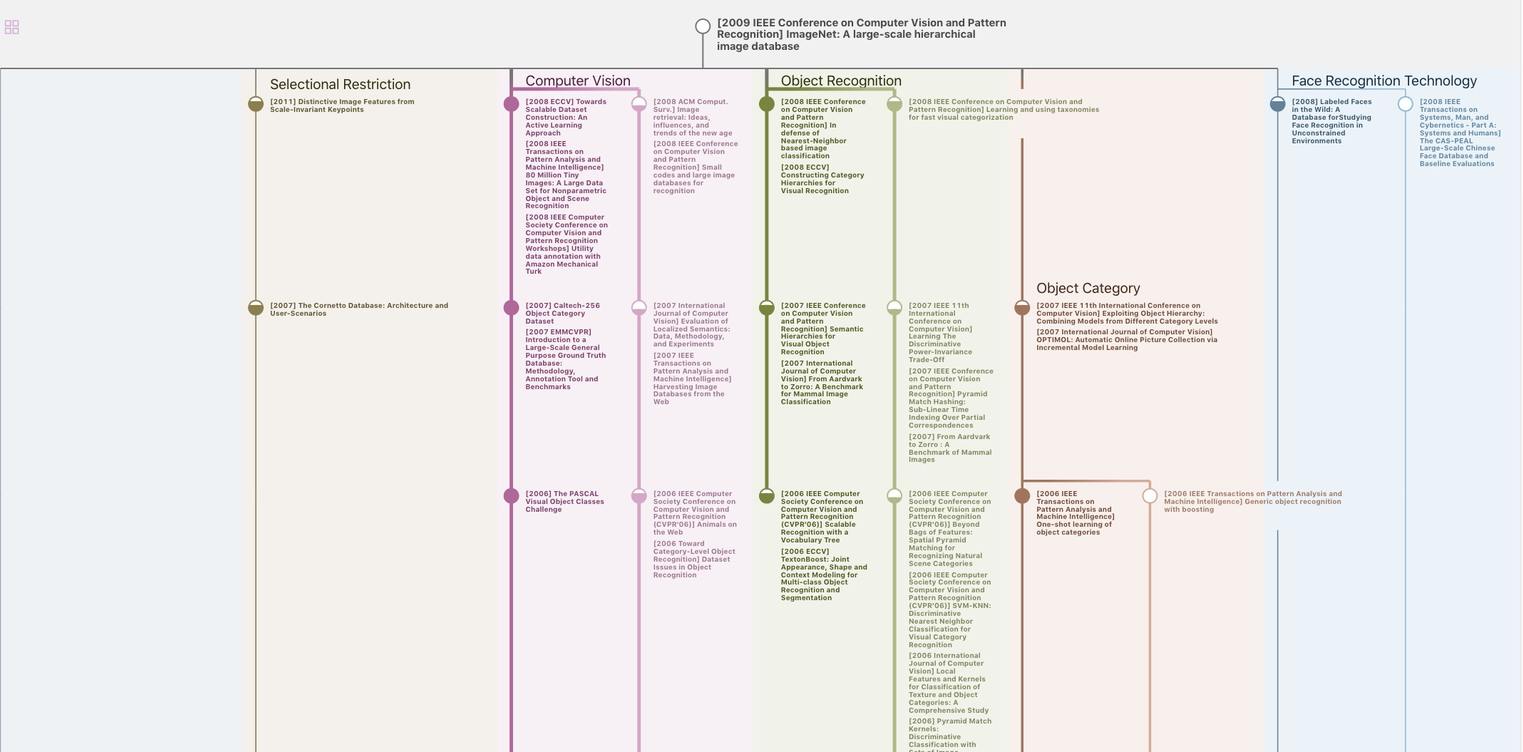
生成溯源树,研究论文发展脉络
Chat Paper
正在生成论文摘要