Deep Representation Learning with Induced Structural Priors
user-5ebe28d54c775eda72abcdf7(2018)
摘要
Neural networks have recently resurged, most prominently in deep learning (DL). The application of DL techniques to large training sets has yielded significant performance gains in image classification [HSK+12, LNC+10] and speech recognition [DYDA12]. However, even though hierarchical neural networks [Elm91, HOT06, LBBH98] have shown great promise in automatically learning thousands or even millions of features for pattern recognition, there nonetheless remain many fundamental open questions about DL. These open questions arise with various aspects of current DL frameworks: the features learned at hidden layers (early hidden layers in particular) are not always “transparent” in their meaning and at times display reduced discriminativeness [ZF14];“vanishing” gradients can sometimes lead to training difficulty [GB10, PMB14]; despite some theoretical work [ERFL14], mathematical understanding of DL is at an early stage. Notwithstanding such issues, DL has proven capable of automatically learning rich hierarchical features combined within an integrated network. Recent techniques such as dropout [HSK+12], dropconnect [LZZ+13], pre-training [DYDA12], and data augmentation [Sch12] bring enhanced performance from various perspec-
更多查看译文
AI 理解论文
溯源树
样例
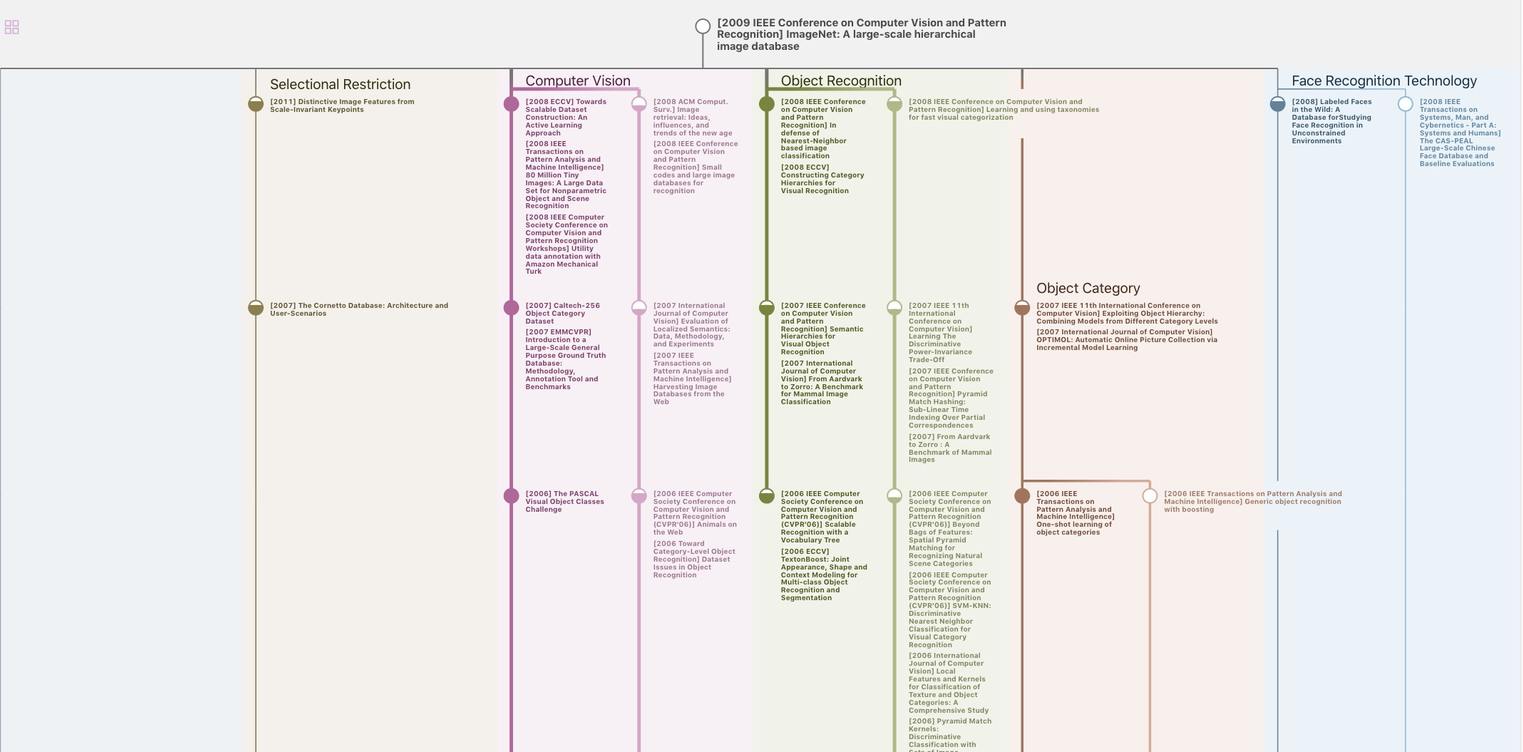
生成溯源树,研究论文发展脉络
Chat Paper
正在生成论文摘要