Real-Time Spatial-Temporal Context Approach For 3d Object Detection Using Lidar
PROCEEDINGS OF THE 6TH INTERNATIONAL CONFERENCE ON VEHICLE TECHNOLOGY AND INTELLIGENT TRANSPORT SYSTEMS (VEHITS)(2020)
摘要
This paper proposes a real-time spatial-temporal context approach for BEV object detection and classification using LiDAR point-clouds. Current state-of-art BEV object-detection approaches focused mainly on single-frame point-clouds while the temporal factor is rarely exploited. In current approach, we aggregate 3D LiDAR point clouds over time to produce a 4D tensor, which is then fed to a one-shot fully convolutional detector to predict oriented 3D object bounding-box information along with object class. Four different techniques are evaluated to incorporate the temporal dimension; a) joint training b) CLSTM c) non-local context network (NLCN) d) spatial-temporal context network (STCN). The experiments are conducted on large-scale Argoverse dataset and results shows that by using NLCN and STCN, mAP accuracy is increased by a large margin over single frame 3D object detector and YOLO4D 3D object detection with our approach running at a speed of 28fps.
更多查看译文
关键词
Bird's-Eye-View (BEV), Convolutional Neural Network (CNN), Non-Local Context Network (NLCN), YOLO, Convolutional LSTM (CLSTM), Spatial-Temporal Context Network (STCN)
AI 理解论文
溯源树
样例
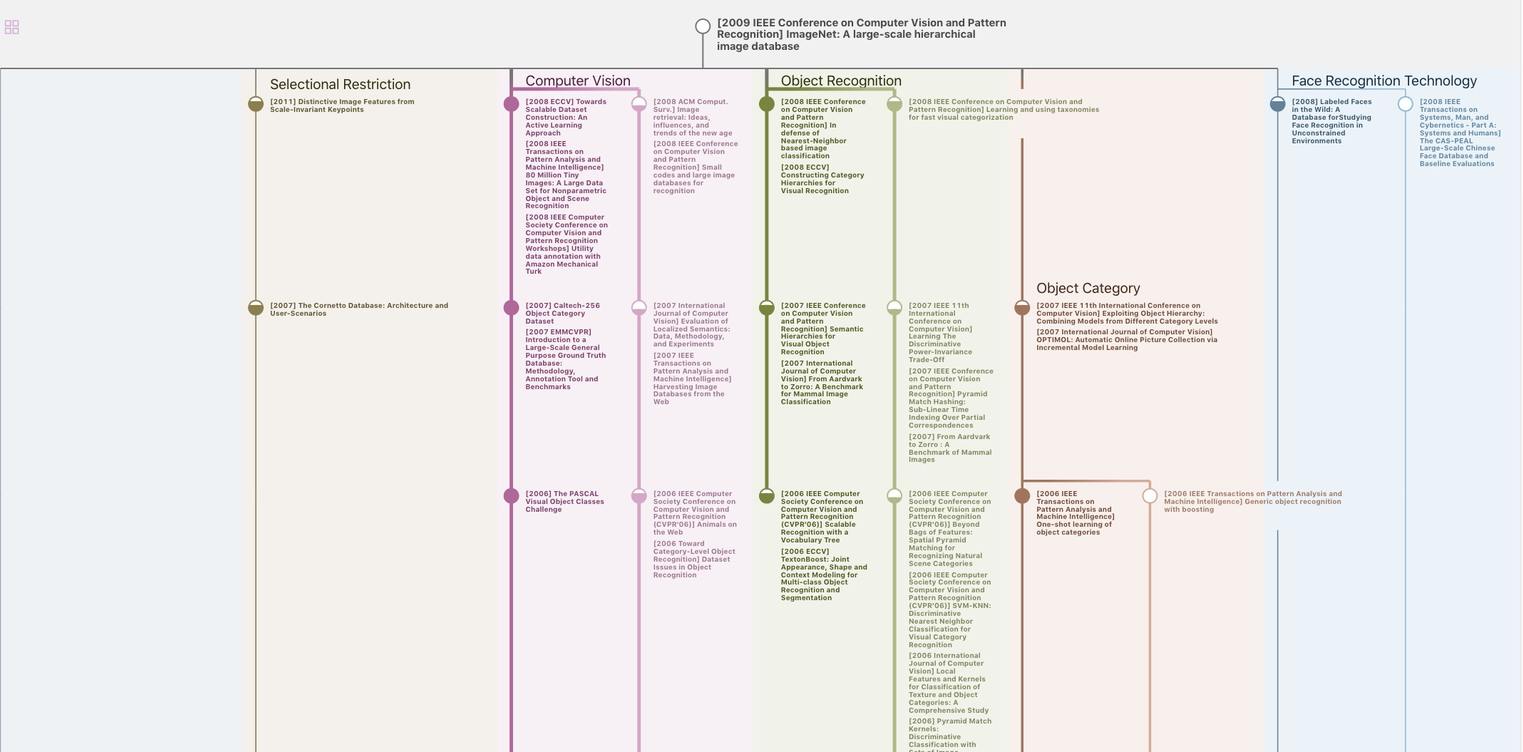
生成溯源树,研究论文发展脉络
Chat Paper
正在生成论文摘要