Toward Sampling For Deep Learning Model Diagnosis
2020 IEEE 36TH INTERNATIONAL CONFERENCE ON DATA ENGINEERING (ICDE 2020)(2020)
摘要
Deep learning (DL) models have achieved paradigm-changing performance in many fields with high dimensional data, such as images, audio, and text. However, the black-box nature of deep neural networks is not only a barrier to adoption in applications such as medical diagnosis, where interpretability is essential, but it also impedes diagnosis of under performing models. The task of diagnosing or explaining DL models requires the computation of additional artifacts, such as activation values and gradients. These artifacts are large in volume, and their computation, storage, and querying raise significant data management challenges.In this paper, we develop a novel data sampling technique that produces approximate but accurate results for these model debugging queries. Our sampling technique utilizes the lower dimension representation learned by the DL model and focuses on model decision boundaries for the data in this lower dimensional space.
更多查看译文
关键词
activation values,significant data management challenges,novel data sampling technique,model debugging queries,DL model,model decision boundaries,deep learning model diagnosis,deep learning models,high dimensional data,black-box nature,deep neural networks,medical diagnosis,performing models,additional artifacts
AI 理解论文
溯源树
样例
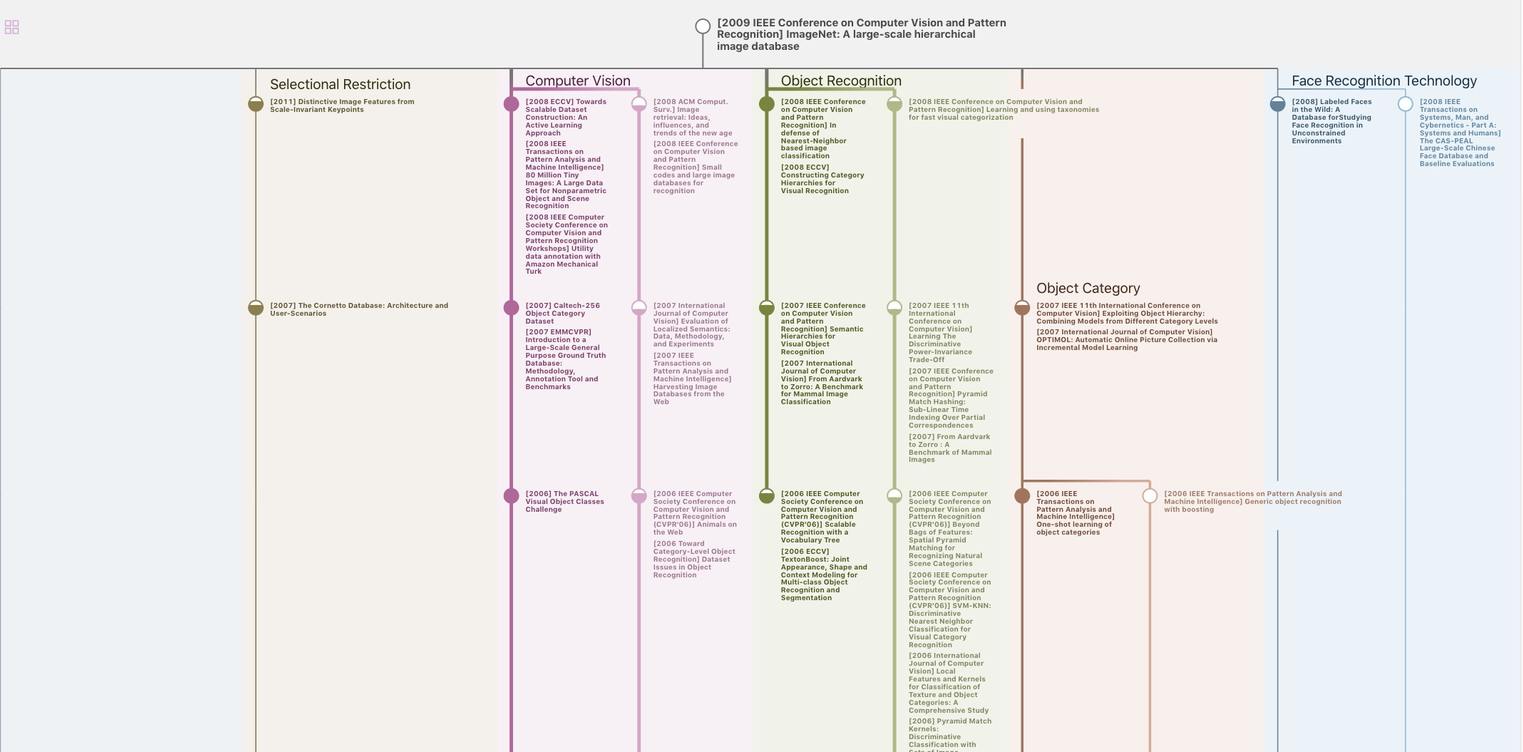
生成溯源树,研究论文发展脉络
Chat Paper
正在生成论文摘要