Generating Realistic Ride-Hailing Datasets Using GANs
ACM Transactions on Spatial Algorithms and Systems(2020)
摘要
AbstractThis article focuses on the synthetic generation of human mobility data in urban areas. We present a novel application of generative adversarial networks (GANs) for modeling and generating human mobility data. We leverage actual ride requests from ride-sharing/hailing services from four major cities to train our GANs model. Our model captures the spatial and temporal variability of the ride request patterns observed for all four cities over a typical week. Previous works have characterized the spatial and temporal properties of human mobility datasets using the fractal dimensionality and the densification power law, respectively, which we utilize to validate our GANs-generated synthetic datasets. We also validate the synthetic datasets using a dynamic vehicle placement application. Such synthetic datasets can avoid privacy concerns and be extremely useful for researchers and policy makers on urban mobility.
更多查看译文
关键词
Ride-sharing, human mobility, datasets, real-time learning, spatial computing
AI 理解论文
溯源树
样例
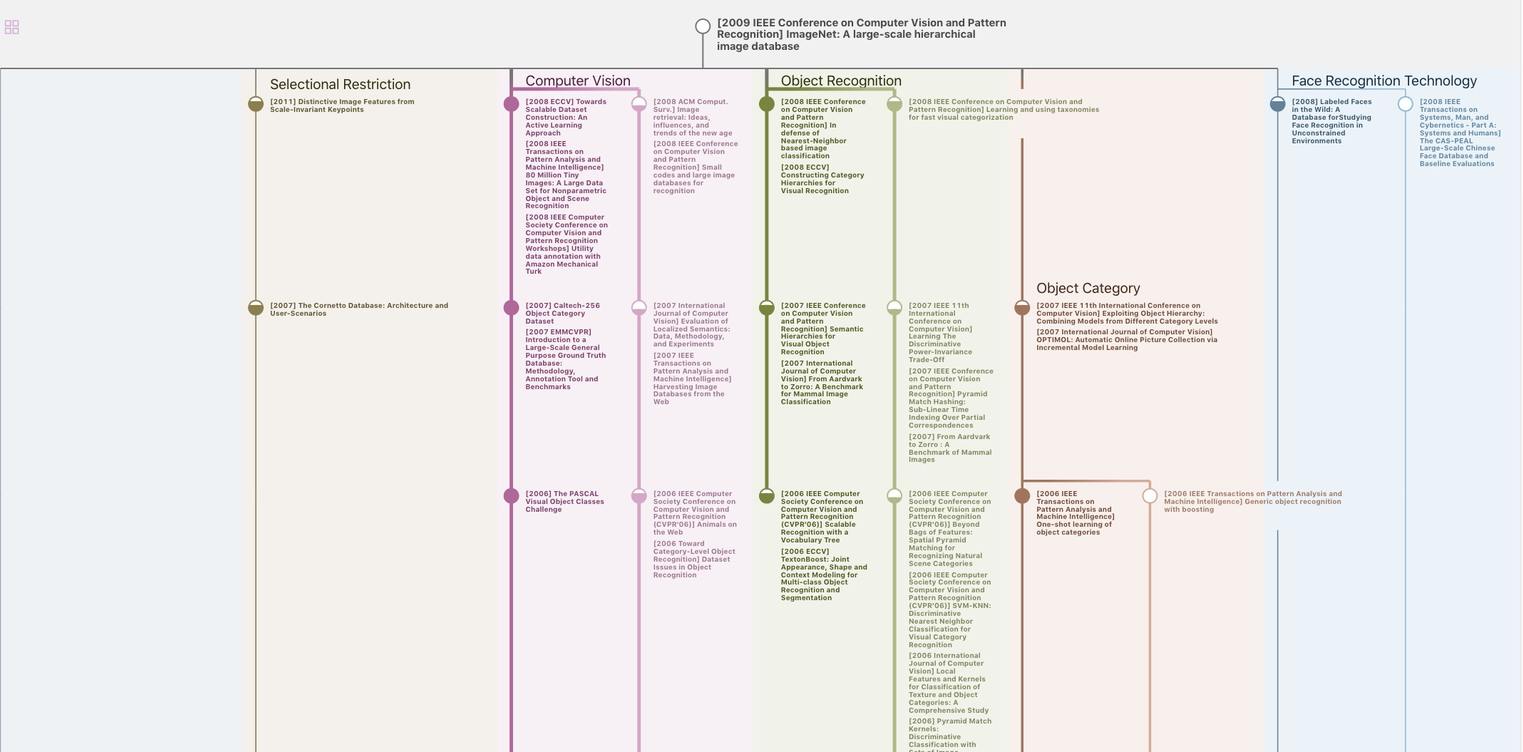
生成溯源树,研究论文发展脉络
Chat Paper
正在生成论文摘要