NormalF-Net: Normal filtering neural network for feature-preserving mesh denoising
Computer-Aided Design(2020)
摘要
Normal filtering is a fundamental step of feature-preserving mesh denoising. Methods based on convolutional neural networks (CNNs) have recently made their debut for normal filtering. However, they require complicated voxelization and/or projection operations for regularization, and afford an overall denoising accuracy with few powers of preserving surface features. We devise a novel normal filtering neural network algorithm, which we call as NormalF-Net. NormalF-Net consists of two cascaded subnetworks with a comprehensive loss function. The first subnetwork learns mapping from non-local patch-group normal matrices (NPNMs) to their ground-truth low-rank counterparts for denoising, and the second subnetwork learns mapping from the recovered NPNMs to the ground-truth normals for normal refinement. Different from existing learning-based methods, NormalF-Net, which bridges the connection between CNNs and geometry domain knowledge of non-local similarity, can not only preserve surface features when removing different levels and types of noise, but be free of voxelization/projection. NormalF-Net has been validated on different datasets of meshes with multi-scale features yet corrupted by noise of different distributions. Experimental results consistently demonstrate clear improvements of our method over the state-of-the-arts in both noise-robustness and feature awareness.
更多查看译文
关键词
NormalF-Net,Normal filtering,Neural network,Low-rank matrix recovery
AI 理解论文
溯源树
样例
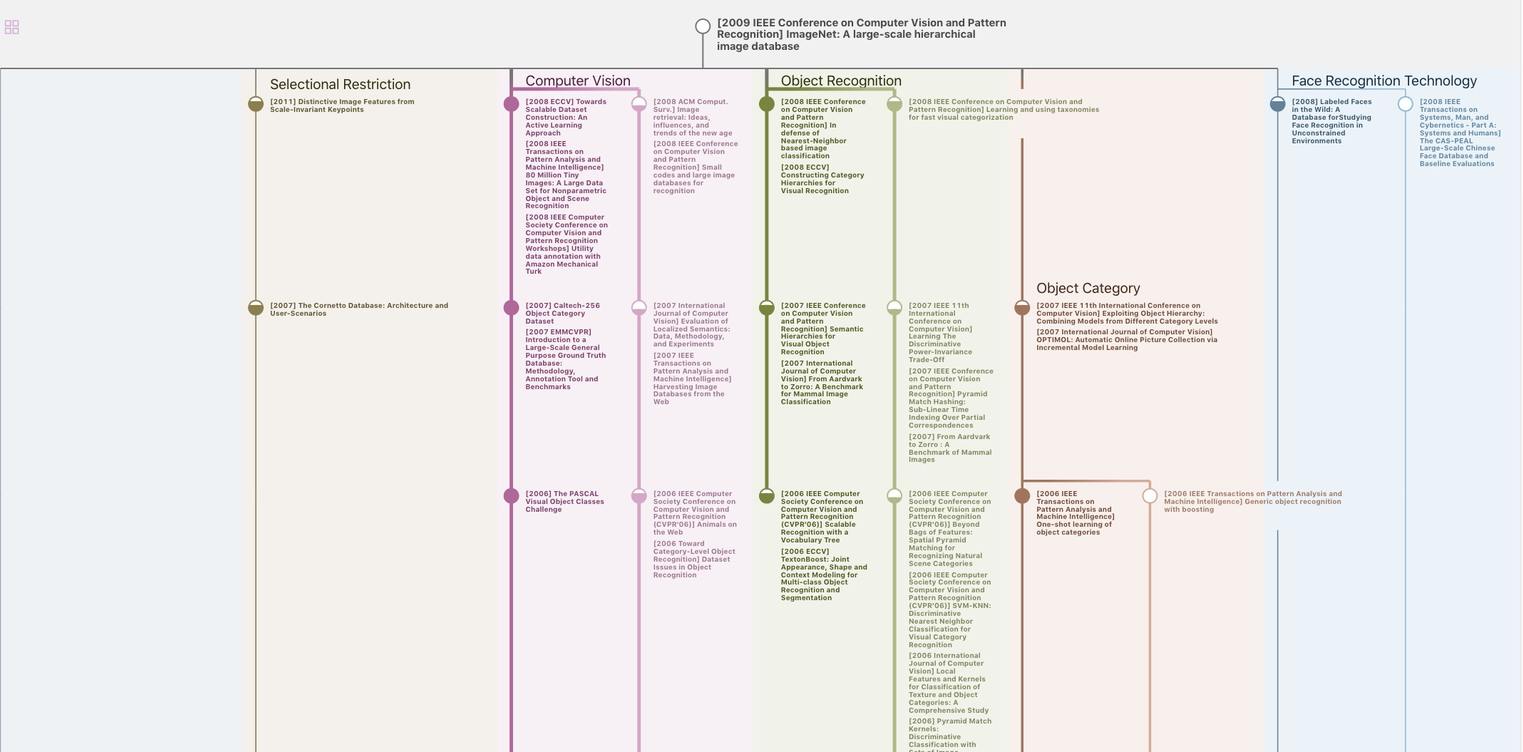
生成溯源树,研究论文发展脉络
Chat Paper
正在生成论文摘要