On the Importance of Views in Unsupervised Representation Learning
preprint(2020)
摘要
In recent years, several self-supervised algorithms in vision have been shown to learn rich representations that perform remarkably well on downstream transfer tasks. We show that these state-of-the-art visual representation learning methods maximize an objective function that is a lower bound on the mutual information between two or more “views", where a view is defined by a composition of image augmentations. This formulation provides a unified theory that connects these vision models (eg Instance Discrimination, Local Aggregation) with well-known language representation learning methods like BERT. To do this, we introduce a new lower bound on mutual information inspired by InfoNCE that we prove supports drawing negative samples from a restricted class of variational distributions. Using the mutual information perspective, we find that the choice of (1) negative samples and (2)“views" are critical to the success of this family of algorithms.
更多查看译文
AI 理解论文
溯源树
样例
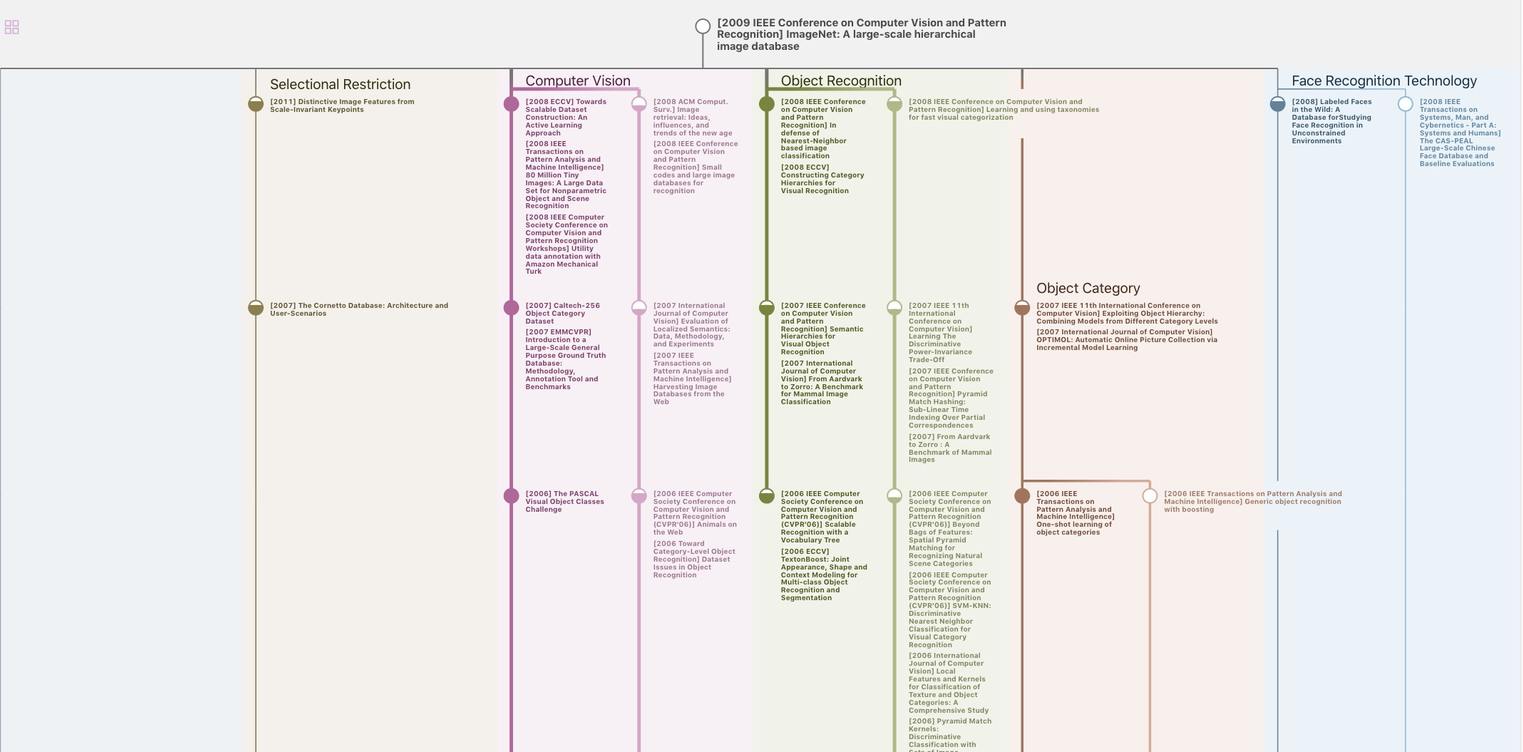
生成溯源树,研究论文发展脉络
Chat Paper
正在生成论文摘要