Equivariant Neural Rendering
ICML(2020)
摘要
We propose a framework for learning neural scene representations directly from images, without 3D supervision. Our key insight is that 3D structure can be imposed by ensuring that the learned representation transforms like a real 3D scene. Specifically, we introduce a loss which enforces equivariance of the scene representation with respect to 3D transformations. Our formulation allows us to infer and render scenes in real time while achieving comparable results to models requiring minutes for inference. In addition, we introduce two challenging new datasets for scene representation and neural rendering, including scenes with complex lighting and backgrounds. Through experiments, we show that our model achieves compelling results on these datasets as well as on standard ShapeNet benchmarks.
更多查看译文
关键词
rendering
AI 理解论文
溯源树
样例
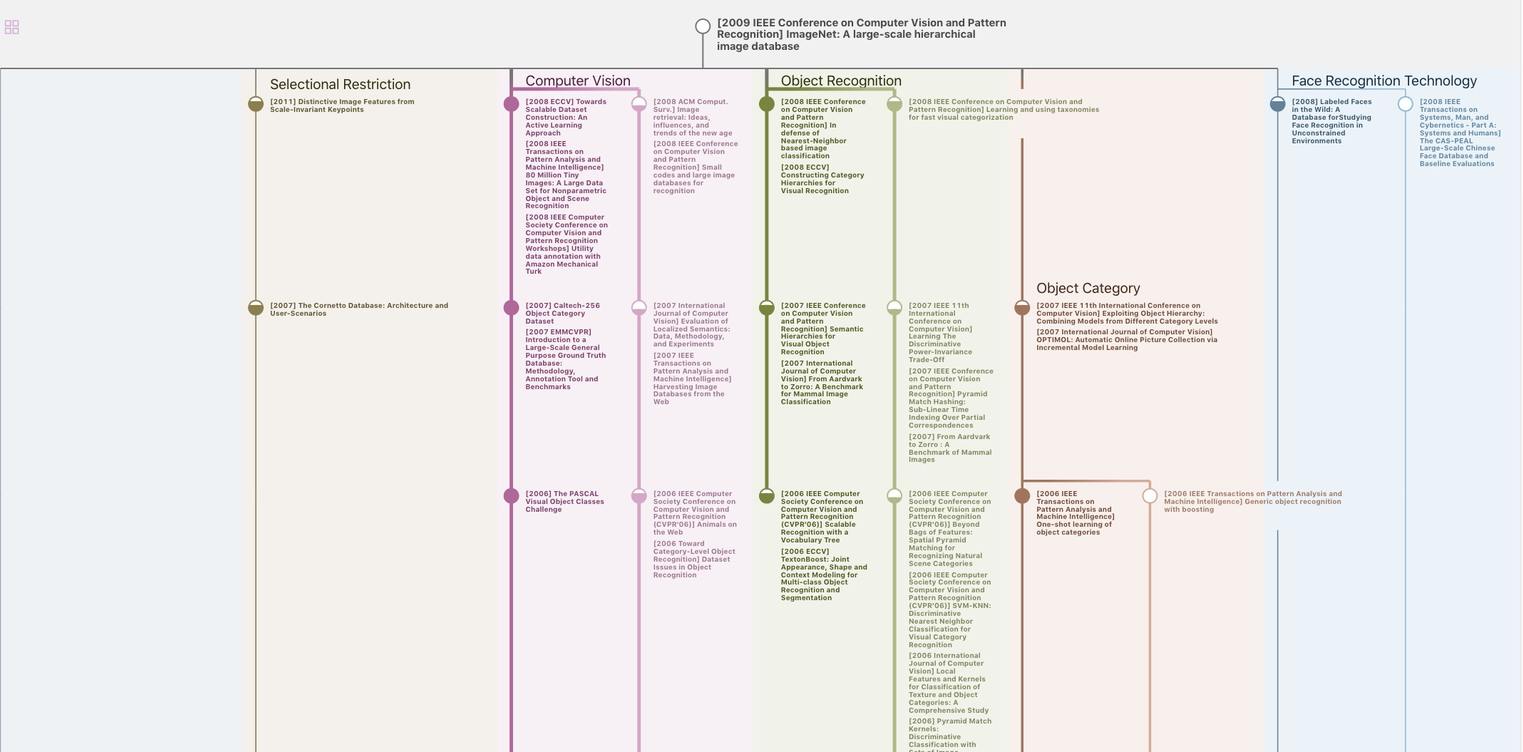
生成溯源树,研究论文发展脉络
Chat Paper
正在生成论文摘要