Inject Machine Learning into Significance Test for Misspecified Linear Models
arxiv(2020)
摘要
Due to its strong interpretability, linear regression is widely used in social science, from which significance test provides the significance level of models or coefficients in the traditional statistical inference. However, linear regression methods rely on the linear assumptions of the ground truth function, which do not necessarily hold in practice. As a result, even for simple non-linear cases, linear regression may fail to report the correct significance level. In this paper, we present a simple and effective assumption-free method for linear approximation in both linear and non-linear scenarios. First, we apply a machine learning method to fit the ground truth function on the training set and calculate its linear approximation. Afterward, we get the estimator by adding adjustments based on the validation set. We prove the concentration inequalities and asymptotic properties of our estimator, which leads to the corresponding significance test. Experimental results show that our estimator significantly outperforms linear regression for non-linear ground truth functions, indicating that our estimator might be a better tool for the significance test.
更多查看译文
关键词
inject machine learning,misspecified linear
AI 理解论文
溯源树
样例
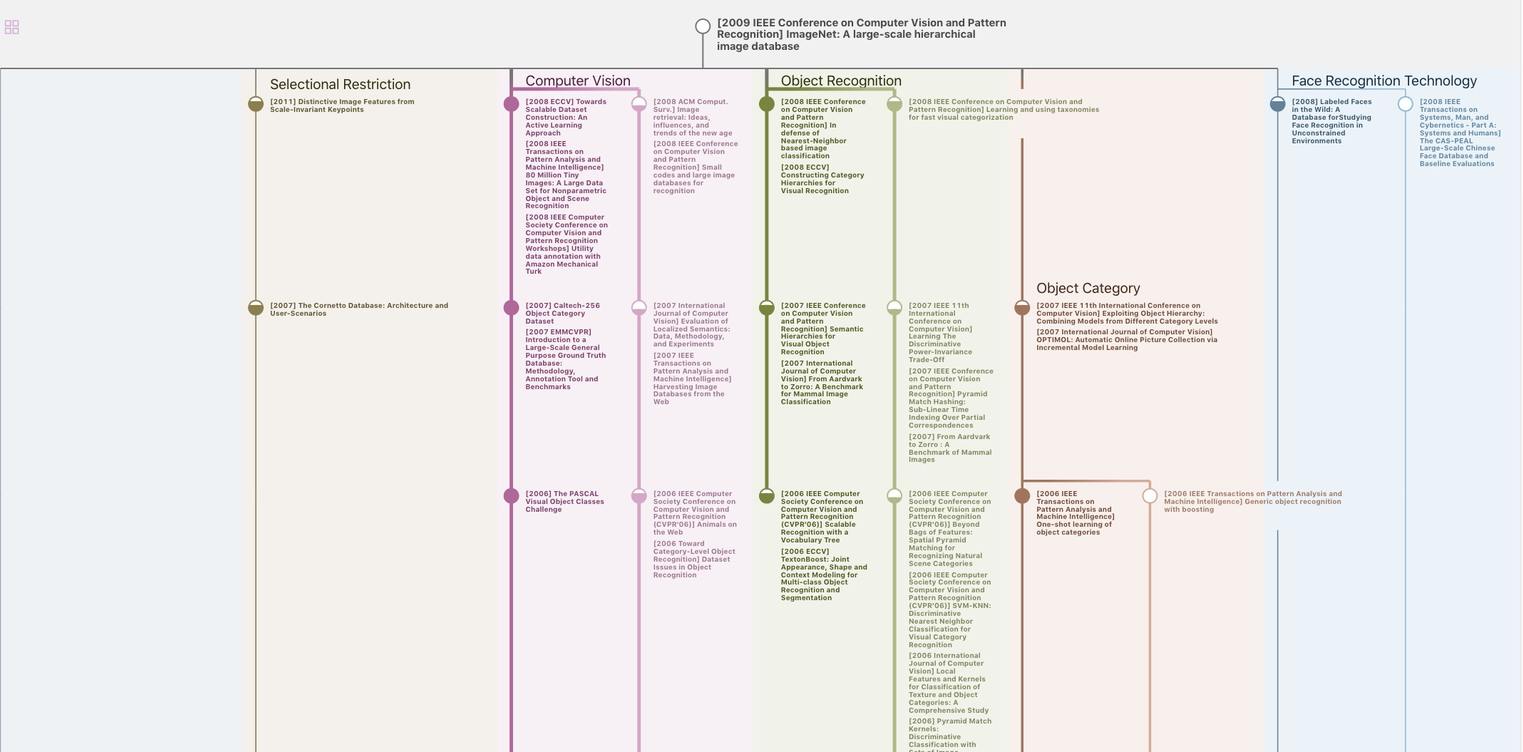
生成溯源树,研究论文发展脉络
Chat Paper
正在生成论文摘要