Content-Aware Inter-Scale Cost Aggregation for Stereo Matching
CoRR(2020)
摘要
Cost aggregation is a key component of stereo matching for high-quality depth estimation. Most methods use multi-scale processing to downsample cost volume for proper context information, but will cause loss of details when upsampling. In this paper, we present a content-aware inter-scale cost aggregation method that adaptively aggregates and upsamples the cost volume from coarse-scale to fine-scale by learning dynamic filter weights according to the content of the left and right views on the two scales. Our method achieves reliable detail recovery when upsampling through the aggregation of information across different scales. Furthermore, a novel decomposition strategy is proposed to efficiently construct the 3D filter weights and aggregate the 3D cost volume, which greatly reduces the computation cost. We first learn the 2D similarities via the feature maps on the two scales, and then build the 3D filter weights based on the 2D similarities from the left and right views. After that, we split the aggregation in a full 3D spatial-disparity space into the aggregation in 1D disparity space and 2D spatial space. Experiment results on Scene Flow dataset, KITTI2015 and Middlebury demonstrate the effectiveness of our method.
更多查看译文
关键词
stereo matching,aggregation,cost,content-aware,inter-scale
AI 理解论文
溯源树
样例
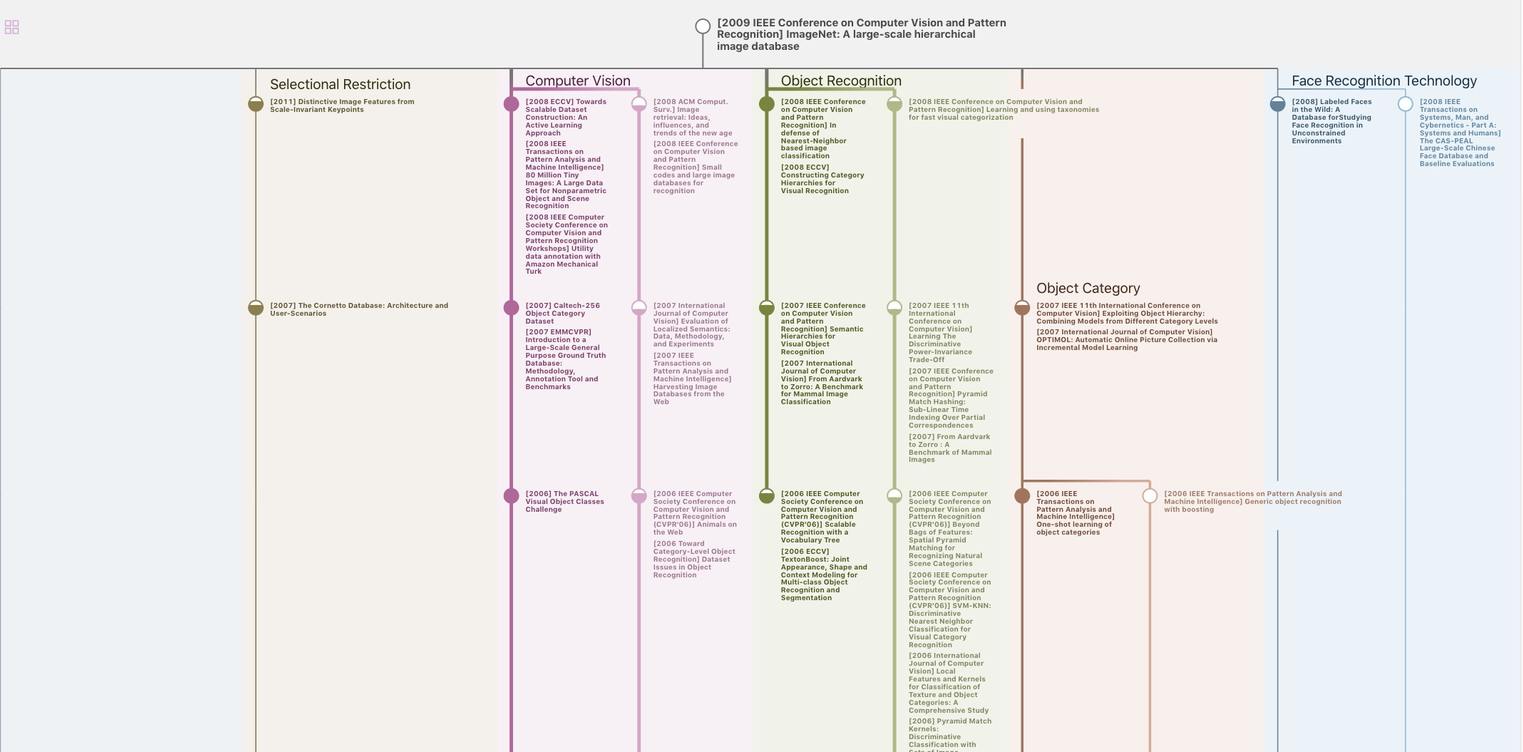
生成溯源树,研究论文发展脉络
Chat Paper
正在生成论文摘要