Learning Context-Aware Policies from Multiple Smart Homes via Federated Multi-Task Learning
2020 IEEE/ACM Fifth International Conference on Internet-of-Things Design and Implementation (IoTDI)(2020)
摘要
Internet-of-Things (IoT) devices deployed in smart homes expose users to cyber threats that can cause privacy leakage (e.g., smart TV eavesdropping) or physical hazards (e.g., smart stove causing fire). Prior work has argued that to effectively detect and prevent such threats, contextual policies are needed to decide if an access to an IoT device should be allowed. Today, however, such contextual access control policies need to be manually generated by IoT developers or users via preinstallation or runtime prompts. Both approaches suffer from potential misconfigurations and often fail to provide coverage over the space of policies. In this paper, our goal is to build a machine learning framework to automatically learn the contextual access control policies from the observed behavioral patterns of users in smart homes. Designing such a learning framework is challenging on two fronts. First, the accuracy is constrained by insufficient data in some smart homes and the diversity of IoT access patterns across different smart homes. Second, since we rely on usage patterns of IoT devices, users will have privacy concerns. We address these challenges in designing LoFTI, a federated multi-task learning framework that learns customized context-aware policies from multiple smart homes in a privacy-preserving manner. Based on prior user studies, we identify six general types of features to capture contextual access patterns. We build a simple machine learning model with temporal structure to achieve a good trade-off between accuracy and communication/computation cost. We design a custom data augmentation mechanism to address the issue of unbalanced data in learning (i.e., few negative vs. normal samples). We show that LoFTI can achieve low false positives/false negatives, reducing the false negative rate by 24.2% and false positive rate by 49.5%, comparing with the state-of-the-art single-home learning and all-home learning mechanism.
更多查看译文
关键词
learning context-aware policies,multiple smart homes,Internet-of-Things devices,smart TV eavesdropping,smart stove,contextual policies,IoT device,contextual access control policies,IoT developers,observed behavioral patterns,IoT access patterns,federated multitask learning framework,customized context-aware policies,contextual access patterns,simple machine learning model,all-home learning mechanism,single-home learning
AI 理解论文
溯源树
样例
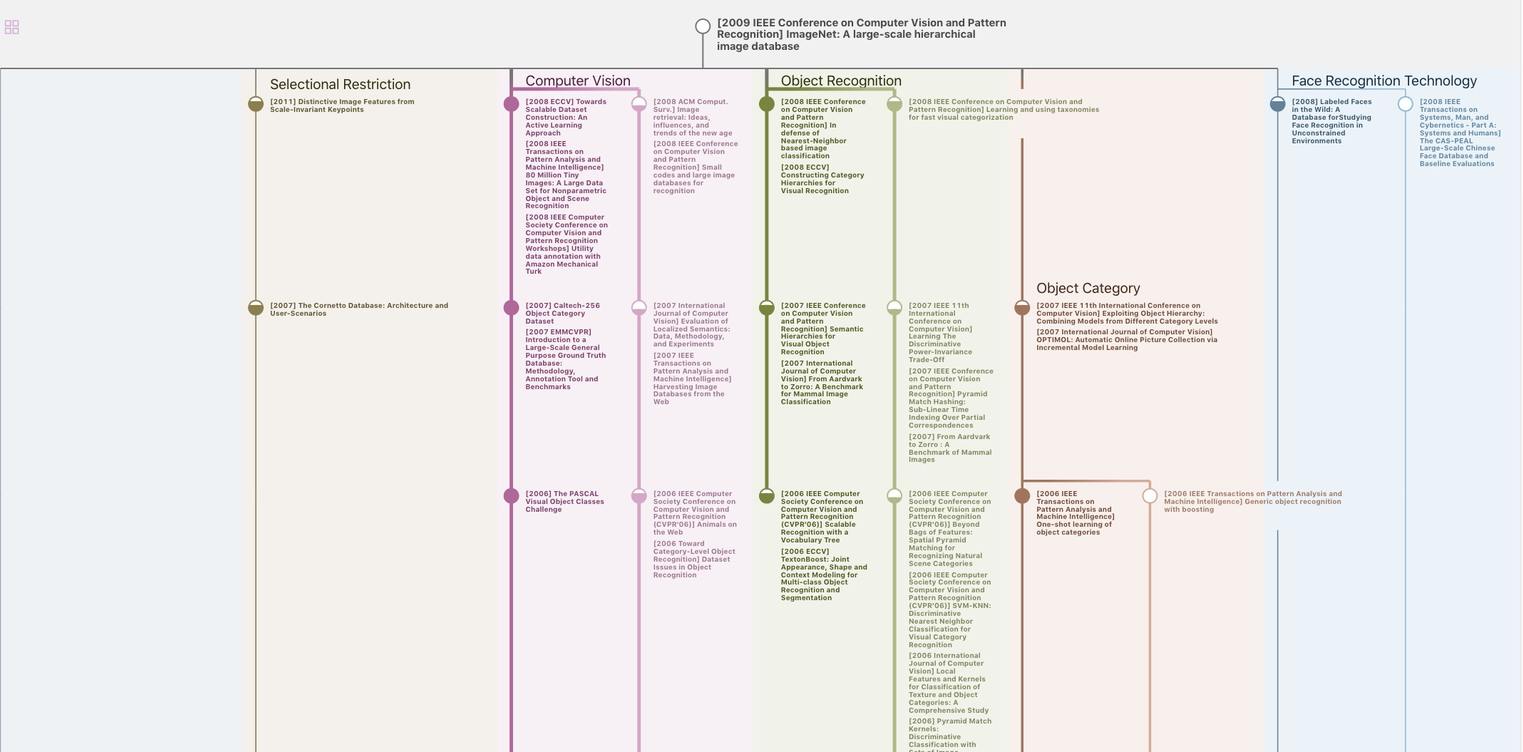
生成溯源树,研究论文发展脉络
Chat Paper
正在生成论文摘要