Network reparameterization for unseen class categorization
user-5e8423bd4c775ee160ac3e1a(2018)
摘要
Many problems with large-scale labeled training data have been impressively solved by deep learning. However, Unseen Class Categorization (UCC) with minimal information provided about target classes is the most commonly encountered setting in industry, which remains a challenging research problem in machine learning. Previous approaches to UCC either fail to generate a powerful discriminative feature extractor or fail to learn a flexible classifier that can be easily adapted to unseen classes. In this paper, we propose to address these issues through network reparameterization,\textit {ie}, reparametrizing the learnable weights of a network as a function of other variables, by which we decouple the feature extraction part and the classification part of a deep classification model to suit the special setting of UCC, securing both strong discriminability and excellent adaptability. Extensive experiments for UCC on several widely-used benchmark datasets in the settings of zero-shot and few-shot learning demonstrate that, our method with network reparameterization achieves state-of-the-art performance.
更多查看译文
AI 理解论文
溯源树
样例
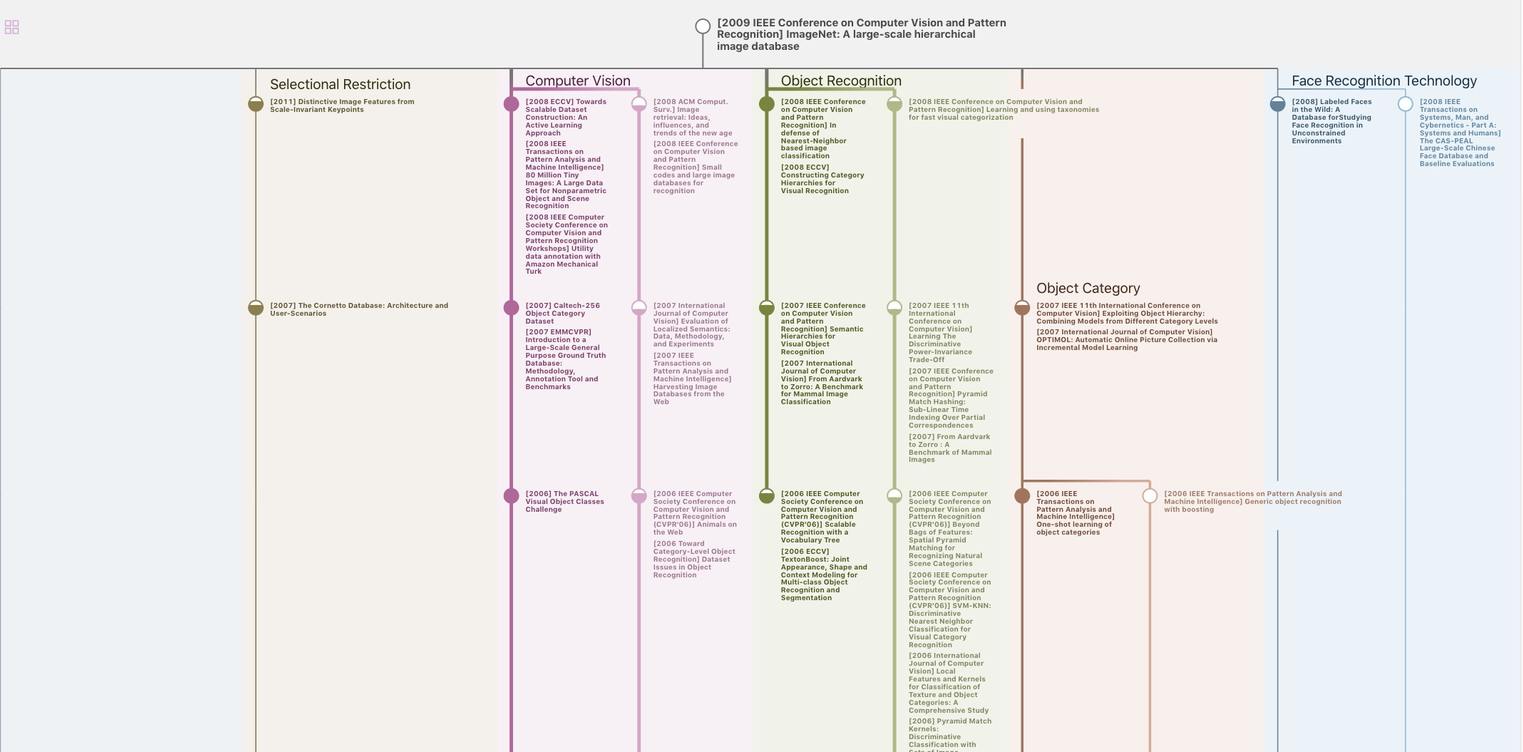
生成溯源树,研究论文发展脉络
Chat Paper
正在生成论文摘要