Online learning of both state and dynamics using ensemble kalman filters
Foundations of Data Science(2021)
摘要
The reconstruction of the dynamics of an observed physical system as a surrogate model has been brought to the fore by recent advances in machine learning. To deal with partial and noisy observations in that endeavor, machine learning representations of the surrogate model can be used within a Bayesian data assimilation framework. However, these approaches require to consider long time series of observational data, meant to be assimilated all together. This paper investigates the possibility to learn both the dynamics and the state online, i.e. to update their estimates at any time, in particular when new observations are acquired. The estimation is based on the ensemble Kalman filter (EnKF) family of algorithms using a rather simple representation for the surrogate model and state augmentation. We consider the implication of learning dynamics online through (i) a global EnKF, (i) a local EnKF and (iii) an iterative EnKF and we discuss in each case issues and algorithmic solutions. We then demonstrate numerically the efficiency and assess the accuracy of these methods using one-dimensional, one-scale and two-scale chaotic Lorenz models.
更多查看译文
关键词
Data assimilation, machine learning, chaotic dynamical systems, parameter estimation, ensemble Kalman filter, local ensemble Kalman filter, iterative ensemble Kalman filter
AI 理解论文
溯源树
样例
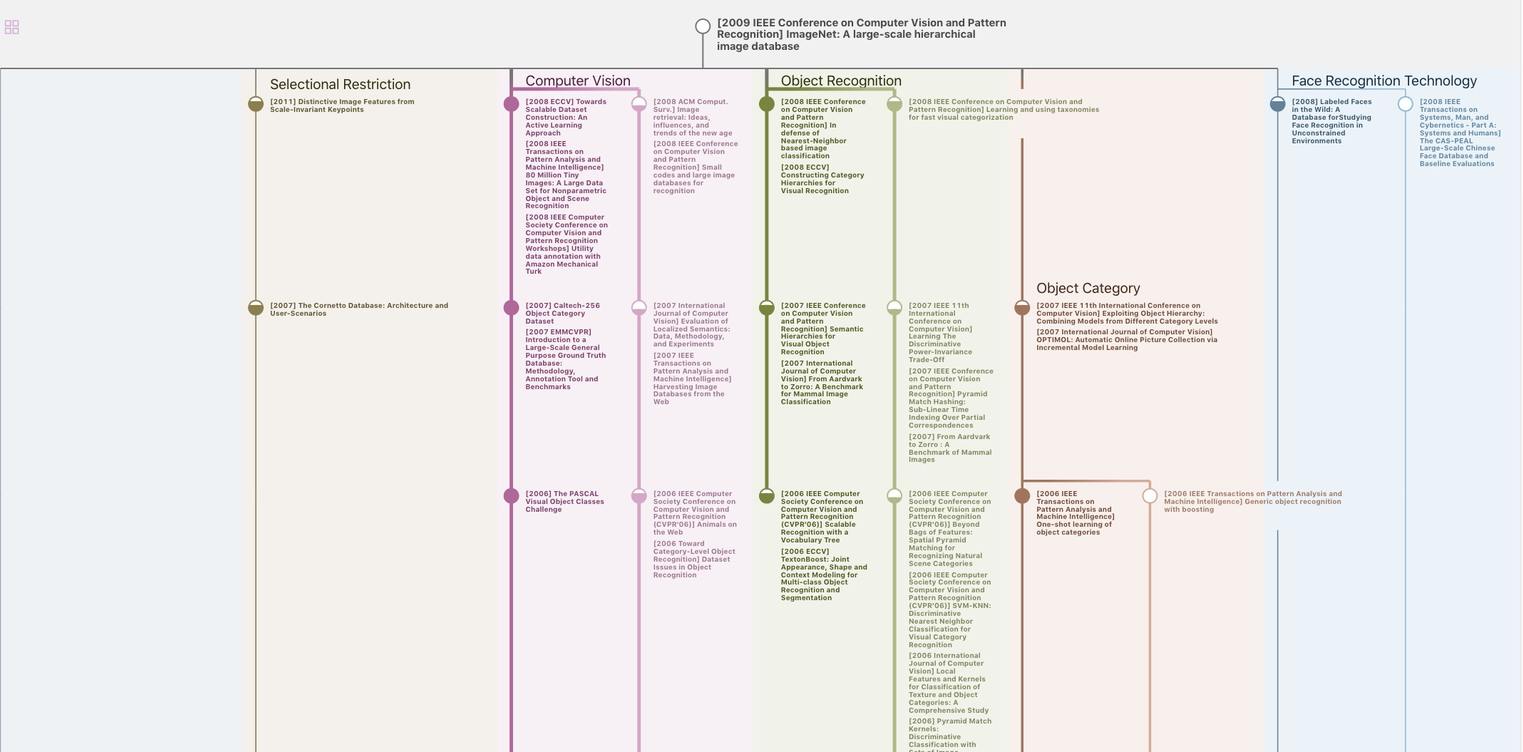
生成溯源树,研究论文发展脉络
Chat Paper
正在生成论文摘要