Fast and Powerful Conditional Randomization Testing via Distillation
arxiv(2020)
摘要
In relating a response variable $Y$ to covariates $(Z,X)$, a key question is whether $Y$ is independent of the covariate $X$ given $Z$. This question can be answered through conditional independence testing, and the conditional randomization test (CRT) was recently proposed by Cand\`es et al. (2018) as a way to use distributional information about $X\mid Z$ to exactly (non-asymptotically) test for conditional independence between $X$ and $Y$ using $any$ test statistic in any dimensionality without assuming anything about $Y\mid (Z,X)$. This flexibility in principle allows one to derive powerful test statistics from complex state-of-the-art machine learning algorithms while maintaining exact statistical control of Type 1 errors. Yet the direct use of such advanced test statistics in the CRT is prohibitively computationally expensive, especially with multiple testing, due to the CRT's requirement to recompute the test statistic many times on resampled data. In this paper we propose a novel approach, called $distillation$, to using state-of-the-art machine learning algorithms in the CRT while drastically reducing the number of times those algorithms need to be run, thereby taking advantage of their power and the CRT's statistical guarantees without suffering the usual computational expense associated with their use in the CRT. In addition to distillation, we propose a number of other tricks to speed up the CRT without sacrificing its strong statistical guarantees, and show in simulation that all our proposals combined lead to a test that has the same power as the CRT but requires orders of magnitude less computation, making it a practical and powerful tool even for large data sets. We demonstrate our method's speed and power on a breast cancer dataset by identifying biomarkers related to cancer stage.
更多查看译文
关键词
Conditional independence test, Conditional randomization test, High-dimensional inference, Machine learning, Model-X
AI 理解论文
溯源树
样例
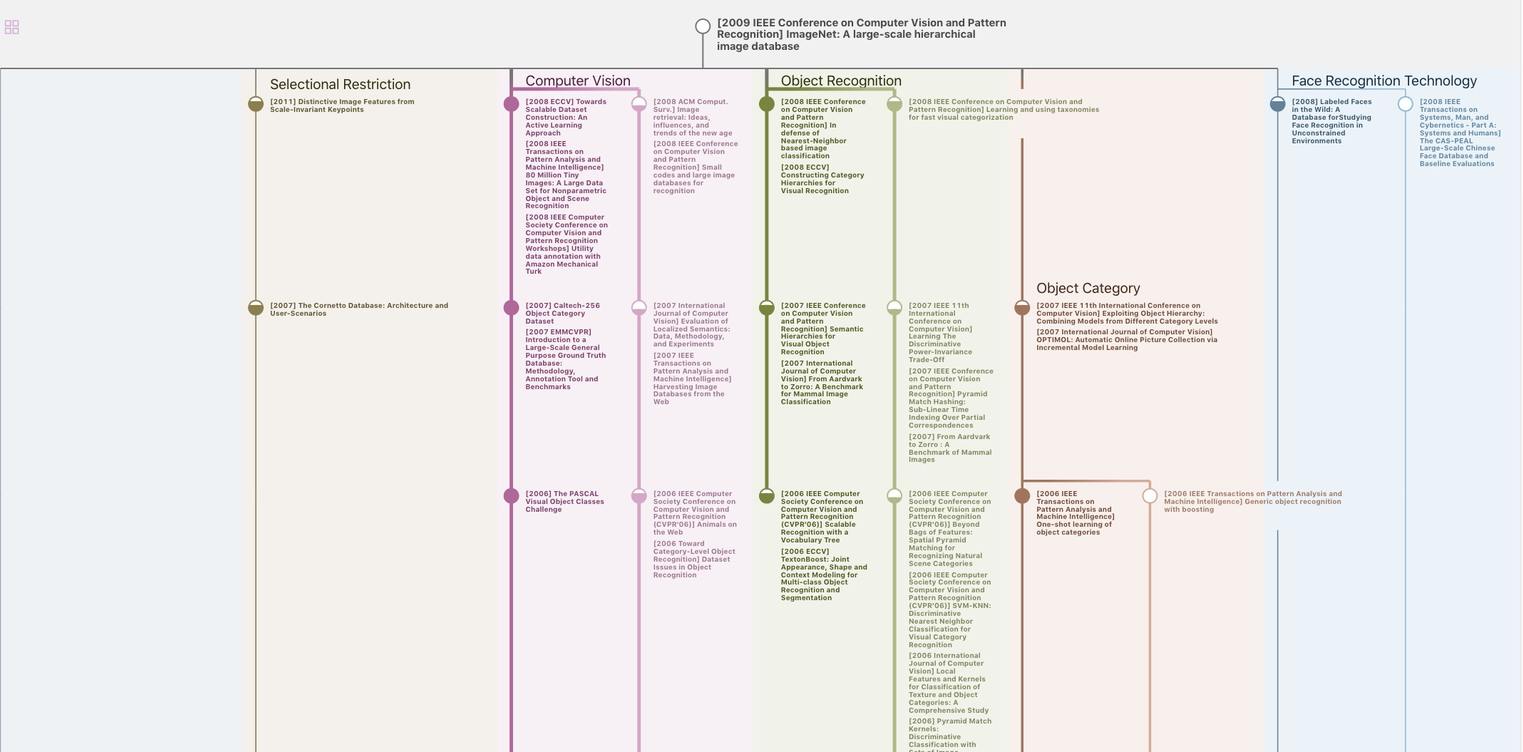
生成溯源树,研究论文发展脉络
Chat Paper
正在生成论文摘要