Semi-Supervised Contrastive Learning with Generalized Contrastive Loss and Its Application to Speaker Recognition
APSIPA(2020)
摘要
This paper introduces a semi-supervised contrastive learning framework and its application to text-independent speaker verification. The proposed framework employs generalized contrastive loss (GCL). GCL unifies losses from two different learning frameworks, supervised metric learning and unsupervised contrastive learning, and thus it naturally determines the loss for semi-supervised learning. In experiments, we applied the proposed framework to text-independent speaker verification on the VoxCeleb dataset. We demonstrate that GCL enables the learning of speaker embeddings in three manners, supervised learning, semi-supervised learning, and unsupervised learning, without any changes in the definition of the loss function.
更多查看译文
关键词
different learning frameworks,supervised metric learning,unsupervised contrastive learning,semisupervised learning,text-independent speaker verification,GCL,speaker embeddings,supervised learning,unsupervised learning,loss function,semisupervised contrastive learning,generalized contrastive loss,speaker recognition,contrastive learning framework
AI 理解论文
溯源树
样例
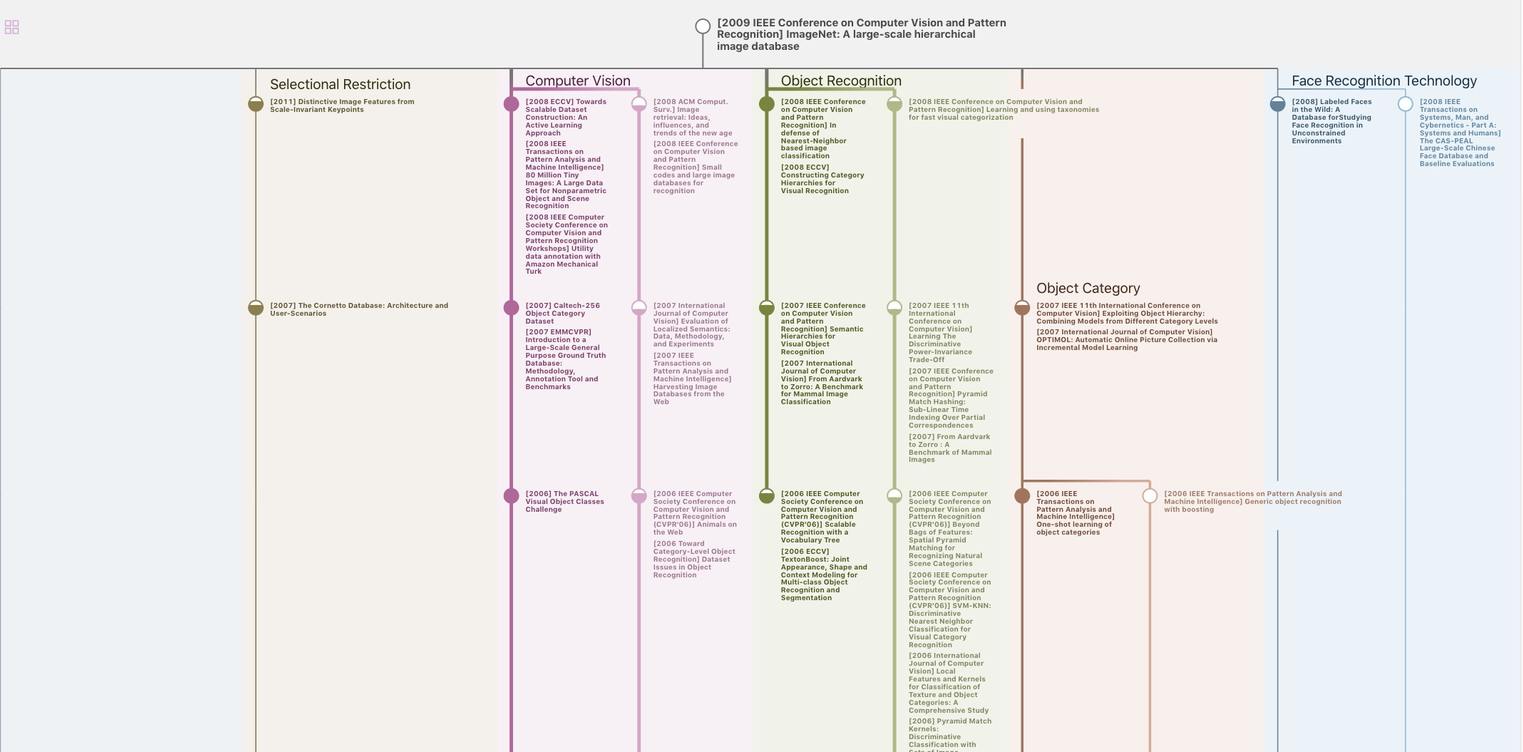
生成溯源树,研究论文发展脉络
Chat Paper
正在生成论文摘要