Schrödinger Principal-Component Analysis: on the Duality Between Principal-Component Analysis and the Schrödinger Equation.
Physical Review E(2021)SCI 3区
MIT | Peking Univ | CALTECH
Abstract
Principal component analysis (PCA) has been applied to analyze random fields in various scientific disciplines. However, the explainability of PCA remains elusive unless strong domain-specific knowledge is available. This paper provides a theoretical framework that builds a duality between the PCA eigenmodes of a random field and eigenstates of a Schrodinger equation. Based on the duality we propose the Schrodinger PCA algorithm to replace the expensive PCA solver with a more sample-efficient Schrodinger equation solver. We verify the validity of the theory and the effectiveness of the algorithm with numerical experiments.
MoreTranslated text
PDF
View via Publisher
AI Read Science
AI Summary
AI Summary is the key point extracted automatically understanding the full text of the paper, including the background, methods, results, conclusions, icons and other key content, so that you can get the outline of the paper at a glance.
Example
Background
Key content
Introduction
Methods
Results
Related work
Fund
Key content
- Pretraining has recently greatly promoted the development of natural language processing (NLP)
- We show that M6 outperforms the baselines in multimodal downstream tasks, and the large M6 with 10 parameters can reach a better performance
- We propose a method called M6 that is able to process information of multiple modalities and perform both single-modal and cross-modal understanding and generation
- The model is scaled to large model with 10 billion parameters with sophisticated deployment, and the 10 -parameter M6-large is the largest pretrained model in Chinese
- Experimental results show that our proposed M6 outperforms the baseline in a number of downstream tasks concerning both single modality and multiple modalities We will continue the pretraining of extremely large models by increasing data to explore the limit of its performance
Try using models to generate summary,it takes about 60s
Must-Reading Tree
Example
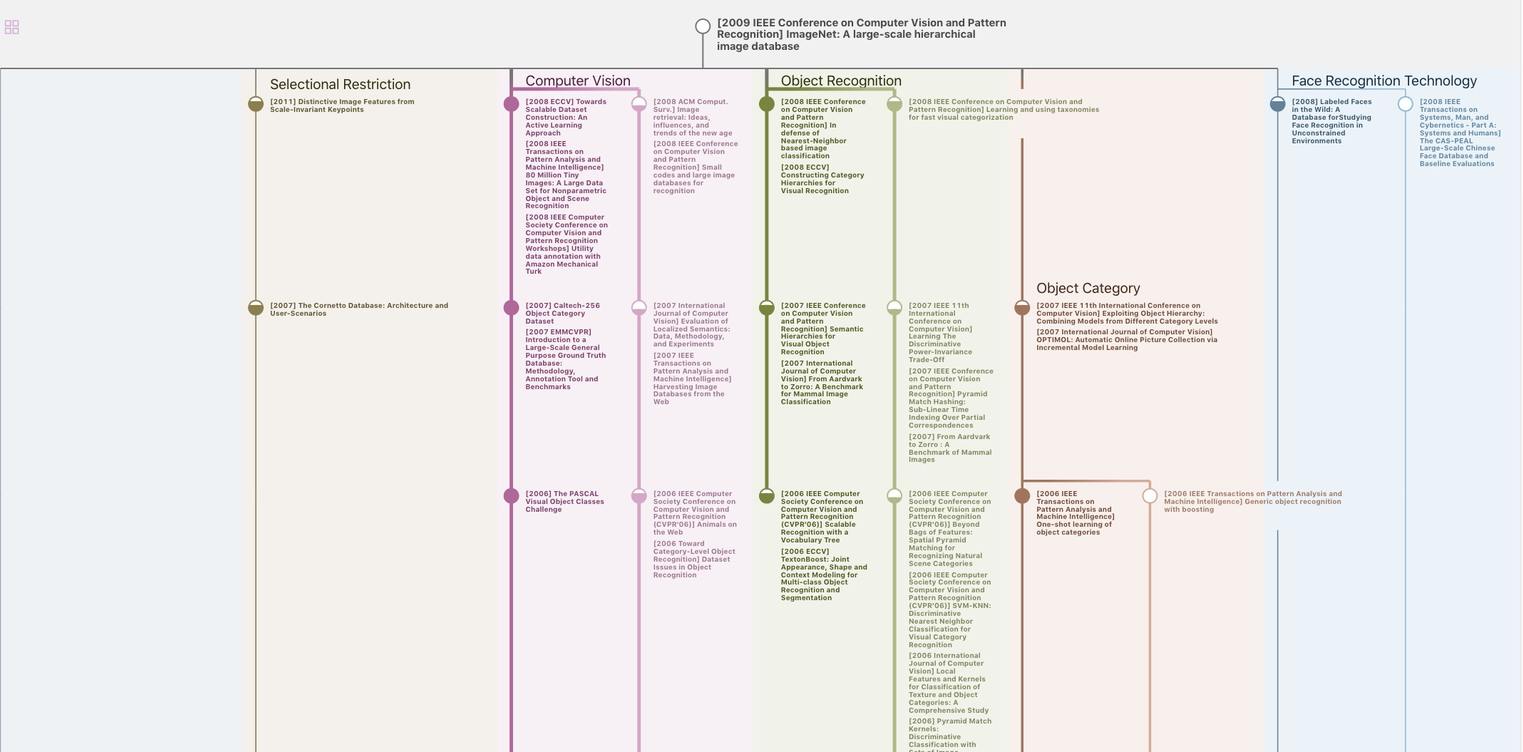
Generate MRT to find the research sequence of this paper
Related Papers
Molecules 2022
被引用1
JOURNAL OF BIOMOLECULAR STRUCTURE & DYNAMICS 2024
被引用1
Journal of Biomolecular Structure and Dynamics 2023
被引用0
Data Disclaimer
The page data are from open Internet sources, cooperative publishers and automatic analysis results through AI technology. We do not make any commitments and guarantees for the validity, accuracy, correctness, reliability, completeness and timeliness of the page data. If you have any questions, please contact us by email: report@aminer.cn
Chat Paper
去 AI 文献库 对话