EDCompress: Energy-Aware Model Compression for Dataflows
IEEE TRANSACTIONS ON NEURAL NETWORKS AND LEARNING SYSTEMS(2024)
摘要
Edge devices demand low energy consumption, cost, and small form factor. To efficiently deploy convolutional neural network (CNN) models on the edge device, energy-aware model compression becomes extremely important. However, existing work did not study this problem well because of the lack of considering the diversity of dataflow types in hardware architectures. In this article, we propose EDCompress (EDC), an energy-aware model compression method for various dataflows. It can effectively reduce the energy consumption of various edge devices, with different dataflow types. Considering the very nature of model compression procedures, we recast the optimization process to a multistep problem and solve it by reinforcement learning algorithms. We also propose a multidimensional multistep (MDMS) optimization method, which shows higher compressing capability than the traditional multistep method. Experiments show that EDC could improve 20x, 17x, and 26x energy efficiency in VGG-16, MobileNet, and LeNet-5 networks, respectively, with negligible loss of accuracy. EDC could also indicate the optimal dataflow type for specific neural networks in terms of energy consumption, which can guide the deployment of CNN on hardware.
更多查看译文
关键词
Energy consumption,Quantization (signal),Hardware,Convolutional neural networks,Adders,Data models,Adaptation models,Dataflow,edge device,efficient artificial intelligence (AI),energy-aware,model compression
AI 理解论文
溯源树
样例
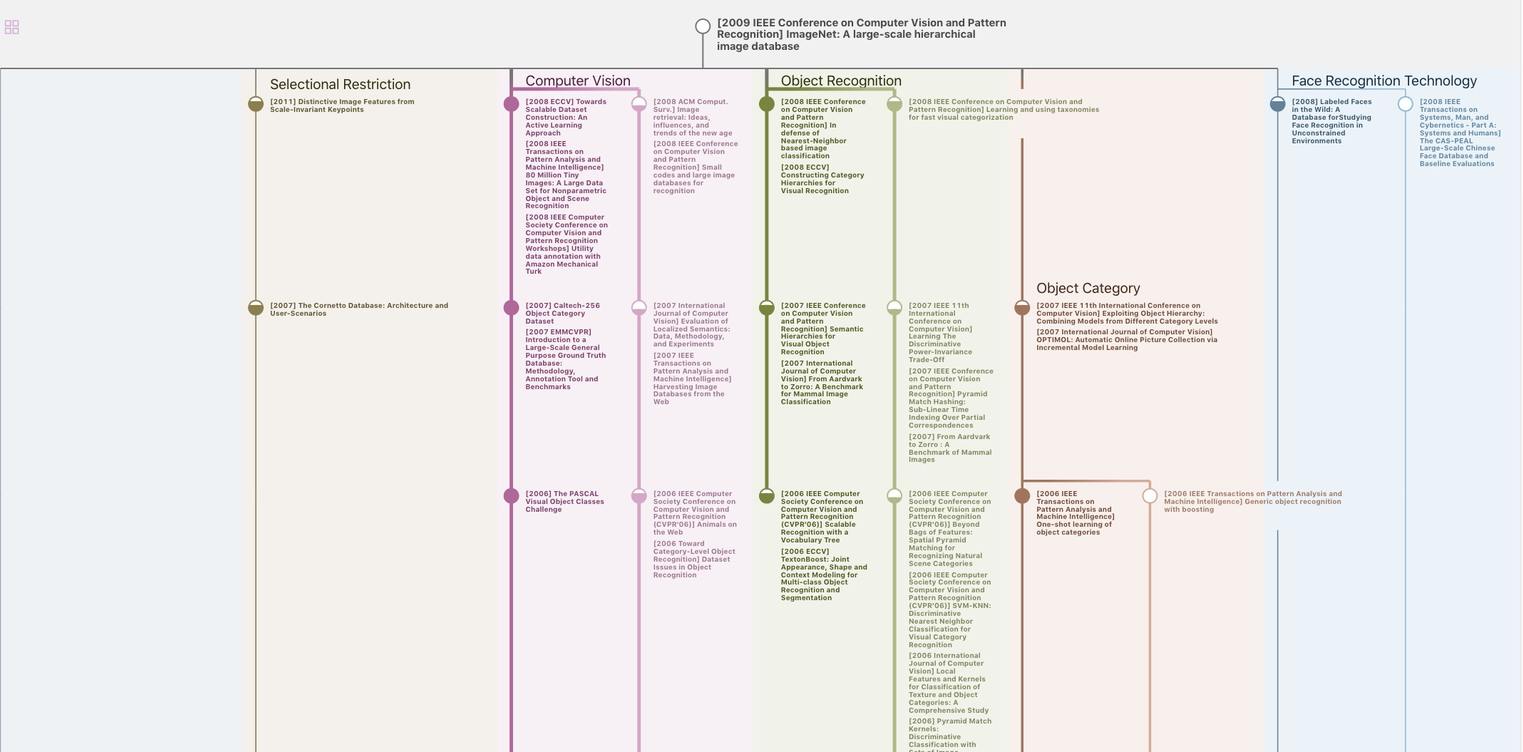
生成溯源树,研究论文发展脉络
Chat Paper
正在生成论文摘要