All your loss are belong to Bayes
NIPS 2020(2020)
摘要
Loss functions are a cornerstone of machine learning and the starting point of most algorithms. Statistics and Bayesian decision theory have contributed, via properness, to elicit over the past decades a wide set of admissible losses in supervised learning, to which most popular choices belong (logistic, square, Matsushita, etc.). Rather than making a potentially biased ad hoc choice of the loss, there has recently been a boost in efforts to fit the loss to the domain at hand while training the model itself. The key approaches fit a canonical link, a function which monotonically relates the closed unit interval to R and can provide a proper loss via integration. In this paper, we rely on a broader view of proper composite losses and a recent construct from information geometry, source functions, whose fitting alleviates constraints faced by canonical links. We introduce a trick on squared Gaussian Processes to obtain a random process whose paths are compliant source functions with many desirable properties in the context of link estimation. Experimental results demonstrate substantial improvements over the state of the art.
更多查看译文
关键词
bayes,loss
AI 理解论文
溯源树
样例
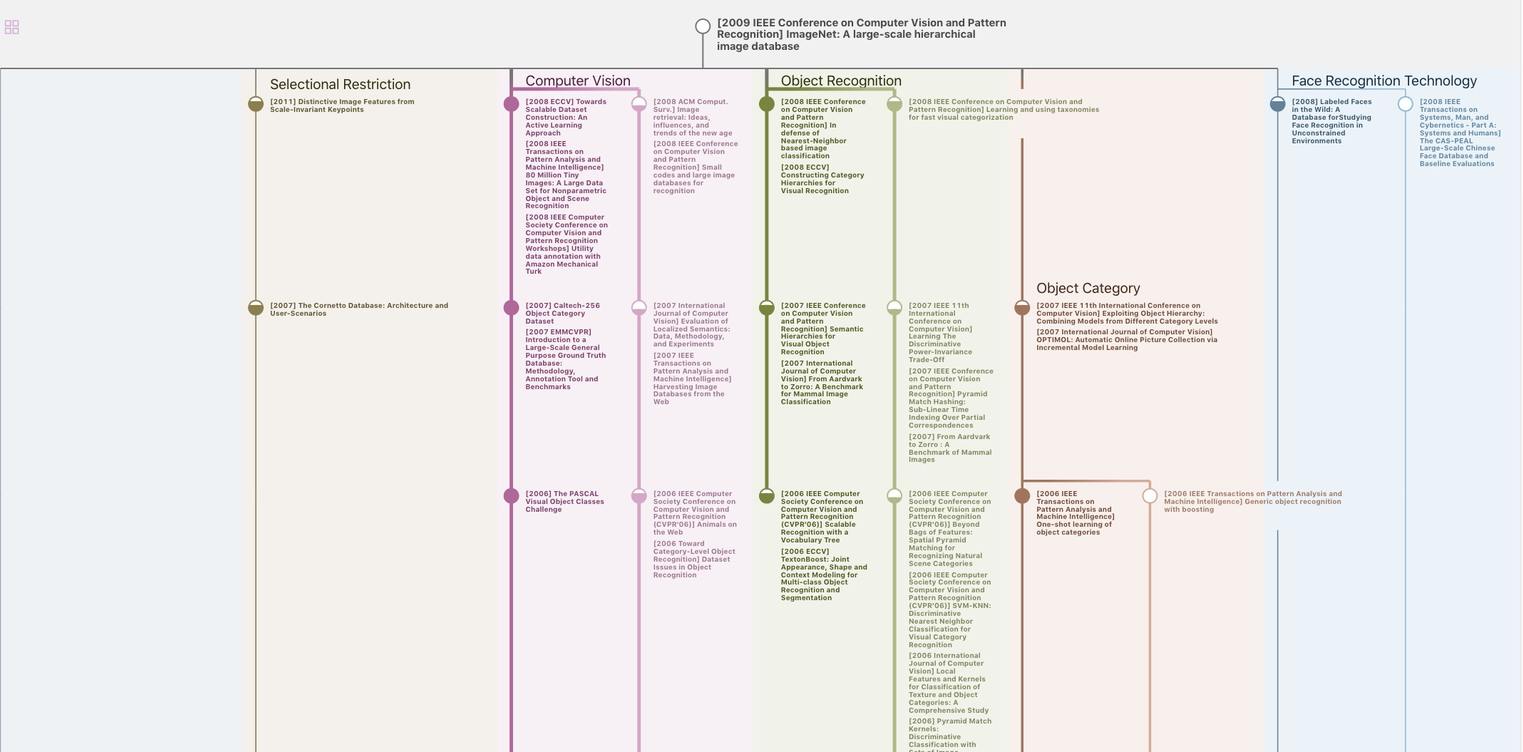
生成溯源树,研究论文发展脉络
Chat Paper
正在生成论文摘要