Neural Architecture Search without Training
INTERNATIONAL CONFERENCE ON MACHINE LEARNING, VOL 139(2021)
摘要
The time and effort involved in hand-designing deep neural networks is immense. This has prompted the development of Neural Architecture Search (NAS) techniques to automate this design. However, NAS algorithms tend to be slow and expensive; they need to train vast numbers of candidate networks to inform the search process. This could be alleviated if we could partially predict a network's trained accuracy from its initial state. In this work, we examine the overlap of activations between datapoints in untrained networks and motivate how this can give a measure which is usefully indicative of a network's trained performance. We incorporate this measure into a simple algorithm that allows us to search for powerful networks without any training in a matter of seconds on a single GPU, and verify its effectiveness on NAS-Bench-101, NAS-Bench-201, NATS-Bench, and Network Design Spaces. Our approach can be readily combined with more expensive search methods; we examine a simple adaptation of regularised evolutionary search. Code for reproducing our experiments is available at https : / /github. com/BayesWatch/nas-without-training.
更多查看译文
关键词
neural architecture search,training
AI 理解论文
溯源树
样例
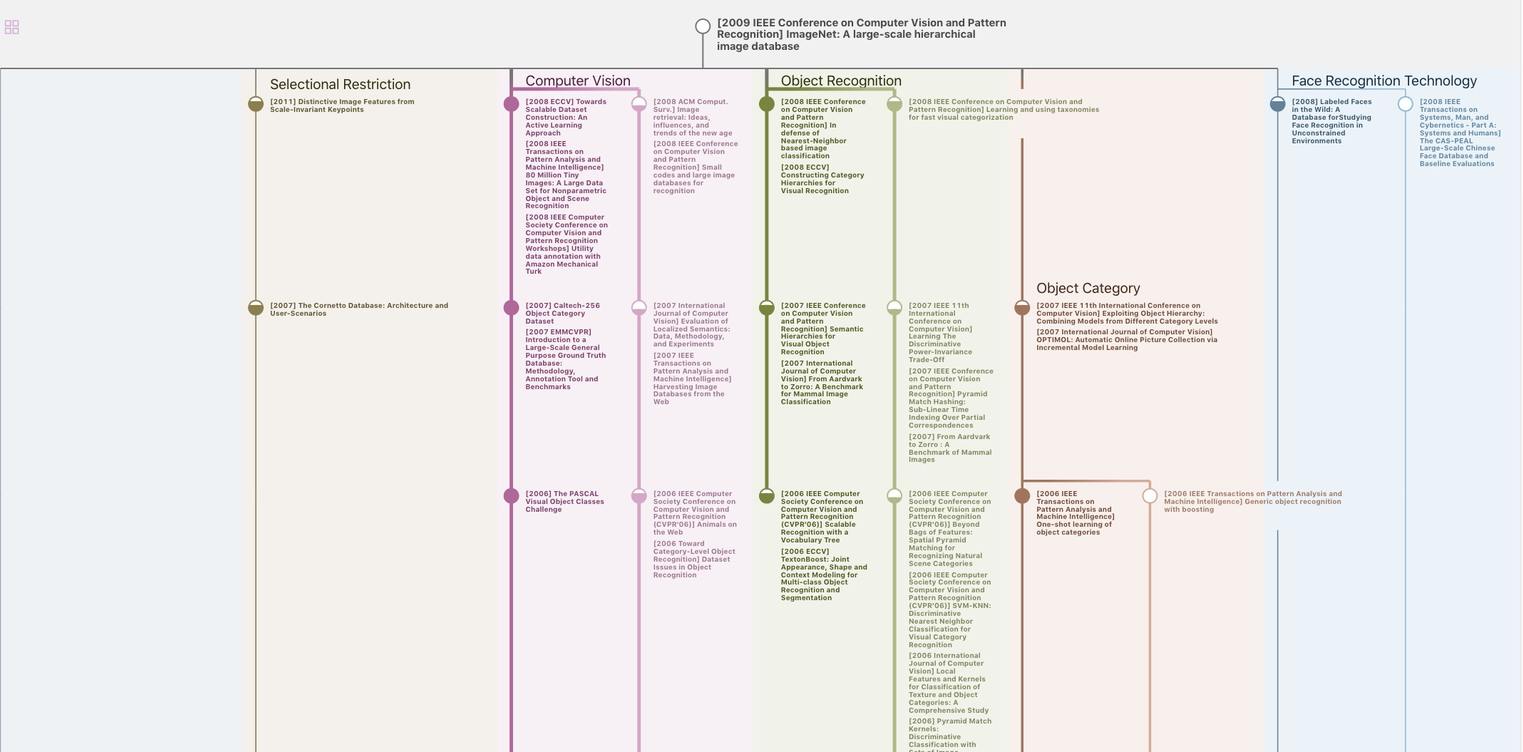
生成溯源树,研究论文发展脉络
Chat Paper
正在生成论文摘要