Reservoir Computing for Jurkat T-cell Segmentation in High Resolution Live Cell Ca2+ Fluorescence Microscopy
2020 IEEE 17th International Symposium on Biomedical Imaging (ISBI)(2020)
摘要
The reservoir computing (RC) paradigm is exploited to detect Jurkat T cells and antibody-coated beads in fluorescence microscopy data. Recent progress in imaging of subcellular calcium (Ca
2
+) signaling offers a high spatial and temporal resolution to characterize early signaling events in T cells. However, data acquisition with dual-wavelength Ca
2
+ indicators, the photo-bleaching at high acquisition rate, low signal-to-noise ratio, and temporal fluctuations of image intensity entail corporation of post-processing techniques into Ca
2
+ imaging systems. Besides, although continuous recording enables real-time Ca
2
+ signal tracking in T cells, reliable automated algorithms must be developed to characterize the cells, and to extract the relevant information for conducting further statistical analyses. Here, we present a robust two-channel segmentation algorithm to detect Jurkat T lymphocytes as well as antibody-coated beads that are utilized to mimic cell-cell interaction and to activate the T cells in microscopy data. Our algorithm uses the reservoir computing framework to learn and recognize the cells - taking the spatiotemporal correlations between pixels into account. A comparison of segmentation accuracy on testing data between our proposed method and the deep learning U-Net model confirms that the developed model provides accurate and computationally cheap solution to the cell segmentation problem.
更多查看译文
关键词
Ca2+ imaging,Jurkat T-cells,Reservoir computing,Segmentation,Machine learning
AI 理解论文
溯源树
样例
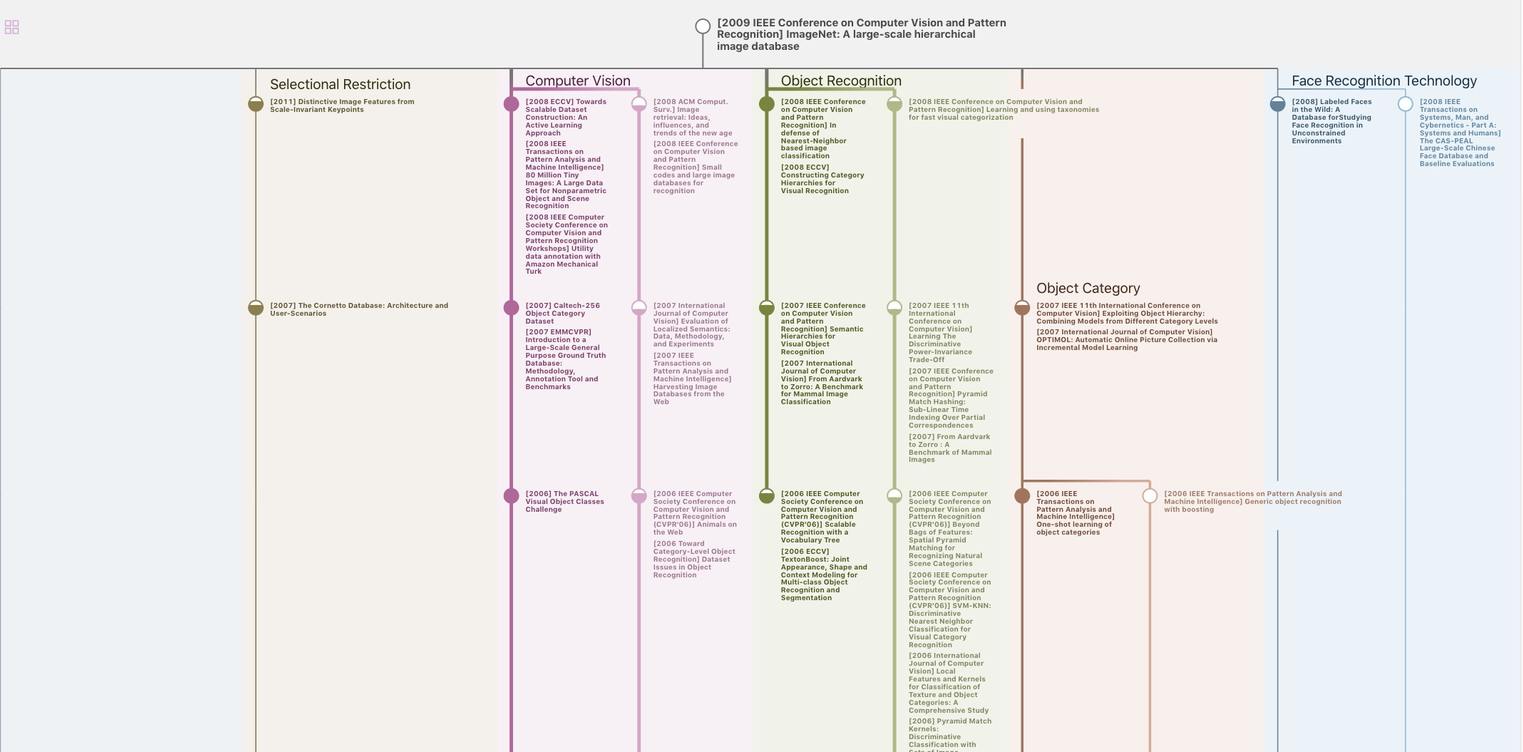
生成溯源树,研究论文发展脉络
Chat Paper
正在生成论文摘要