Machine-learning Assisted Screening of Energetic Materials.
JOURNAL OF PHYSICAL CHEMISTRY A(2020)
摘要
In this work, machine learning (ML), materials informatics (MI), and thermochemical data are combined to screen potential candidates of energetic materials. To directly characterize energetic performance, the heat of explosion Delta H-e is used as the target property. The critical descriptors of cohesive energy, averaged over all constituent elements and the oxygen balance, are found by forward stepwise selection from a large number of possible descriptors. With them and a theoretically labeled Delta H-e training data set, a satisfactory surrogate ML model is trained. The ML model is applied to large databases ICSD and PubChem to predict Delta H-e. At the gross-level filtering by the ML model, 2732 molecular candidates based on carbon, hydrogen, nitrogen, and oxygen (CHNO) with high Delta H-e values are predicted. Afterward, a fine-level thermochemical screening is carried out on the 2732 materials, resulting in 262 candidates with TNT equivalent power index P-e(TNT) greater than 1.5. Raising P-e(TNT) further to larger than 1.8, 29 potential candidates are found from the 2732 materials, all are new to the current reservoir of well-known energetic materials.
更多查看译文
AI 理解论文
溯源树
样例
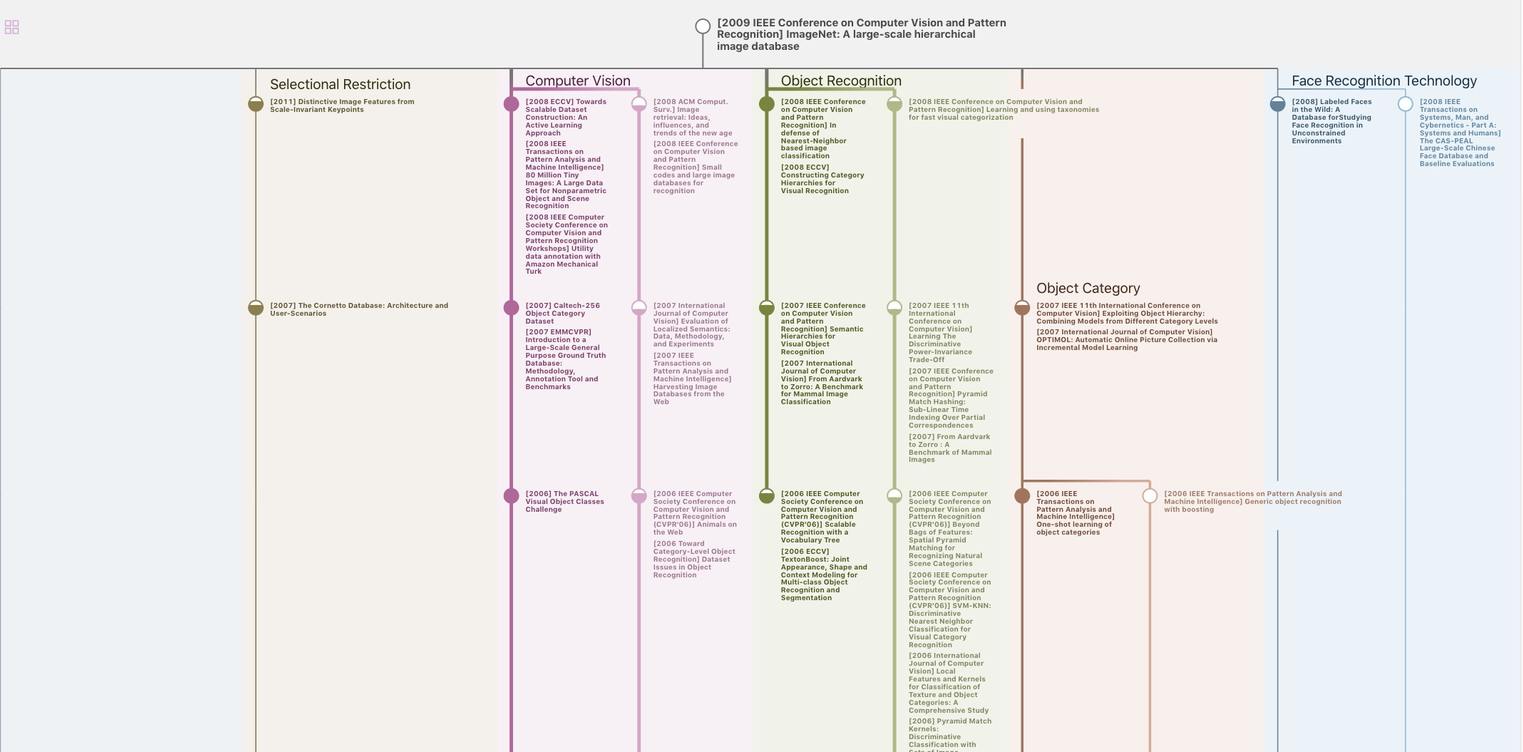
生成溯源树,研究论文发展脉络
Chat Paper
正在生成论文摘要