Mutual purification for unsupervised domain adaptation in person re-identification
Neural Computing and Applications(2022)
摘要
Unsupervised domain adaptation person re-identification aims to adapt the model learned on a labeled source domain to an unlabeled target domain. It has attracted extensively attention in the computer vision community due to its important applications in security and surveillance. Existing approaches are primary to utilize pseudo labels assigned by clustering algorithm as supervision information on target domain. One of the most successful methods exploits neighbors of each unlabeled image to generate pseudo labels, in which contain noise and errors inevitably. In this paper, we propose a Mutual Purification Network (MP-Net) to alleviate errors of pseudo-labels and errors accumulation. MP-Net aims to utilize two independent pre-trained models to learn external auxiliary knowledge from each other, which consists of mutual purification neighbors-exploiting (MPNE) and mutual purification selection (MPS). MPNE focuses on using the common and unique knowledge of the two models to mine more robust neighbor relationships for alleviating the noise and errors from the normal neighbors exploiting. MPS aims to filter outliers and construct reliable triplets during training with triplet loss in unsupervised scenario. Each model selects more reliable and informative positives and negatives for peer models based on dynamic ranking and isolation computation. Extensive experiments on three large-scale public datasets, including Market-1501, DukeMTMC and MSMT17 illustrate that our MP-Net outperforms or shows comparable results to the state-of-the-art methods.
更多查看译文
关键词
Person re-identification,Unsupervised domain adaptation,K-reciprocal encoding,Triplet loss
AI 理解论文
溯源树
样例
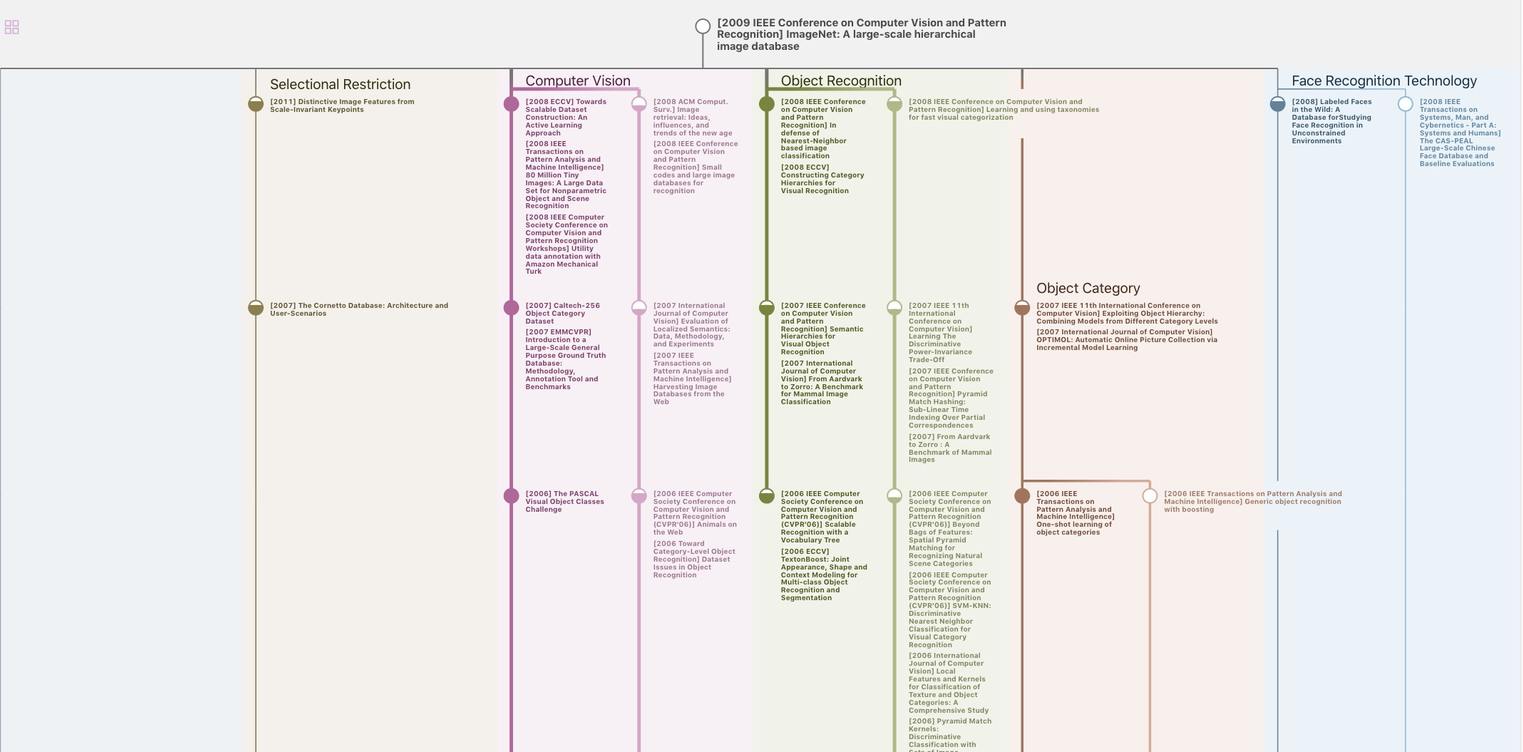
生成溯源树,研究论文发展脉络
Chat Paper
正在生成论文摘要