Deep-Learning-Enabled Fast Optical Identification and Characterization of 2D Materials.
ADVANCED MATERIALS(2020)
摘要
Advanced microscopy and/or spectroscopy tools play indispensable roles in nanoscience and nanotechnology research, as they provide rich information about material processes and properties. However, the interpretation of imaging data heavily relies on the "intuition" of experienced researchers. As a result, many of the deep graphical features obtained through these tools are often unused because of difficulties in processing the data and finding the correlations. Such challenges can be well addressed by deep learning. In this work, the optical characterization of 2D materials is used as a case study, and a neural-network-based algorithm is demonstrated for the material and thickness identification of 2D materials with high prediction accuracy and real-time processing capability. Further analysis shows that the trained network can extract deep graphical features such as contrast, color, edges, shapes, flake sizes, and their distributions, based on which an ensemble approach is developed to predict the most relevant physical properties of 2D materials. Finally, a transfer learning technique is applied to adapt the pretrained network to other optical identification applications. This artificial-intelligence-based material characterization approach is a powerful tool that would speed up the preparation, initial characterization of 2D materials and other nanomaterials, and potentially accelerate new material discoveries.
更多查看译文
关键词
2D materials,deep learning,machine learning,material characterization,optical microscopy
AI 理解论文
溯源树
样例
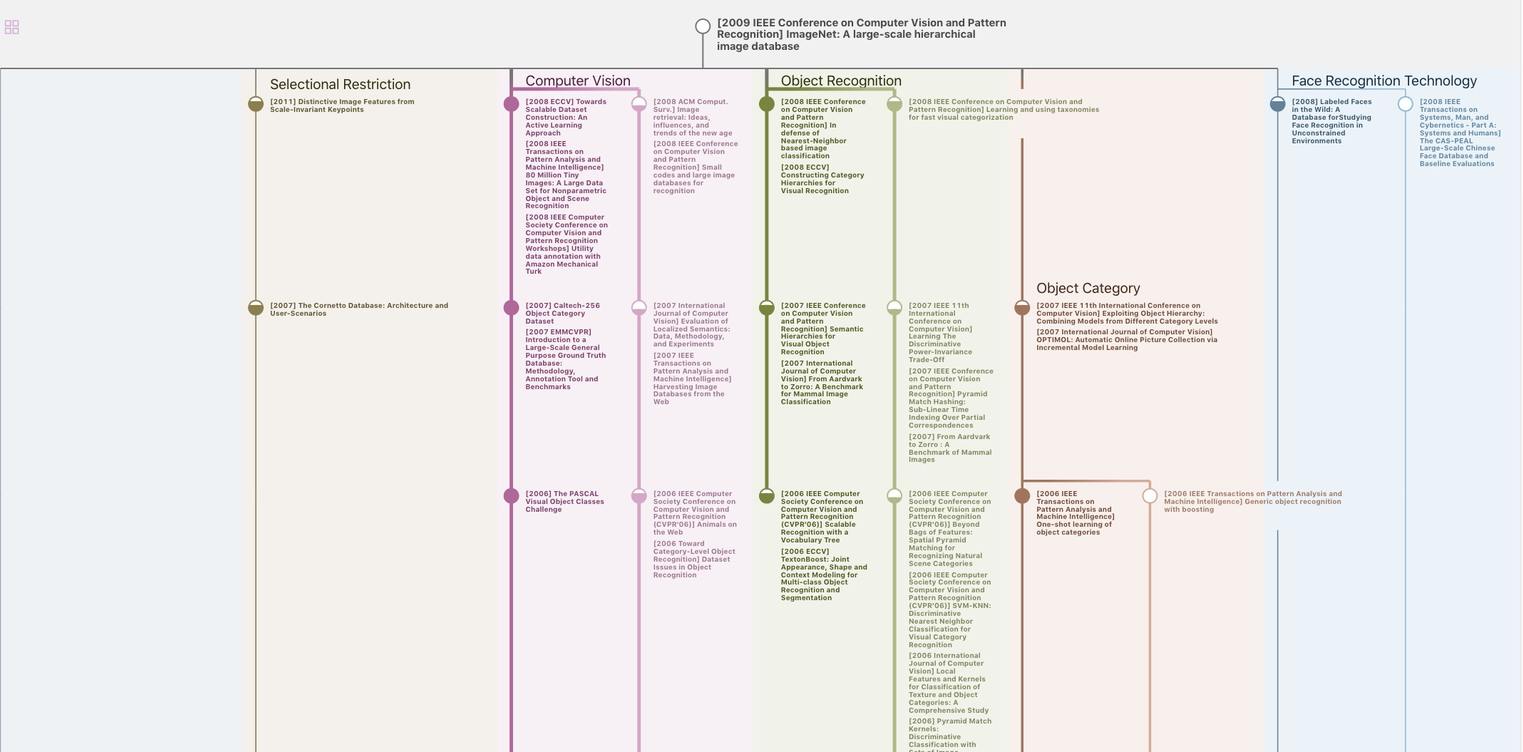
生成溯源树,研究论文发展脉络
Chat Paper
正在生成论文摘要