Clustering Causal Additive Noise Models
arxiv(2021)
摘要
Additive noise models are commonly used to infer the causal direction for a given set of observed data. Most causal models assume a single homogeneous population. However, observations may be collected under different conditions in practice. Such data often require models that can accommodate possible heterogeneity caused by different conditions under which data have been collected. We propose a clustering algorithm inspired by the $k$-means algorithm, but with unknown $k$. Using the proposed algorithm, both the labels and the number of components are estimated from the collected data. The estimated labels are used to adjust the causal direction test statistic. The adjustment significantly improves the performance of the test statistic in identifying the correct causal direction.
更多查看译文
关键词
causal additive noise models
AI 理解论文
溯源树
样例
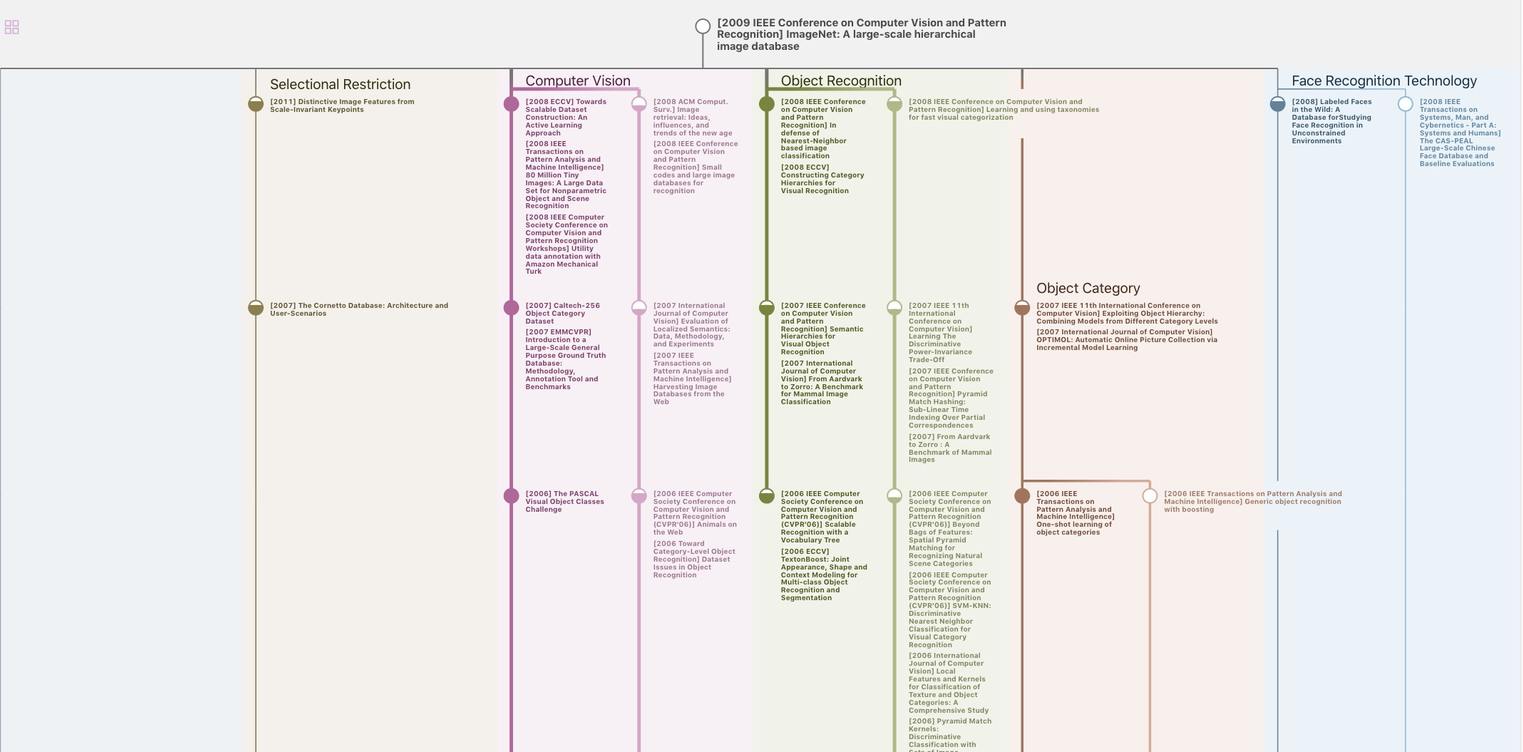
生成溯源树,研究论文发展脉络
Chat Paper
正在生成论文摘要