Regret Balancing for Bandit and RL Model Selection
arxiv(2020)
摘要
We consider model selection in stochastic bandit and reinforcement learning problems. Given a set of base learning algorithms, an effective model selection strategy adapts to the best learning algorithm in an online fashion. We show that by estimating the regret of each algorithm and playing the algorithms such that all empirical regrets are ensured to be of the same order, the overall regret balancing strategy achieves a regret that is close to the regret of the optimal base algorithm. Our strategy requires an upper bound on the optimal base regret as input, and the performance of the strategy depends on the tightness of the upper bound. We show that having this prior knowledge is necessary in order to achieve a near-optimal regret. Further, we show that any near-optimal model selection strategy implicitly performs a form of regret balancing.
更多查看译文
关键词
bandit,rl model selection
AI 理解论文
溯源树
样例
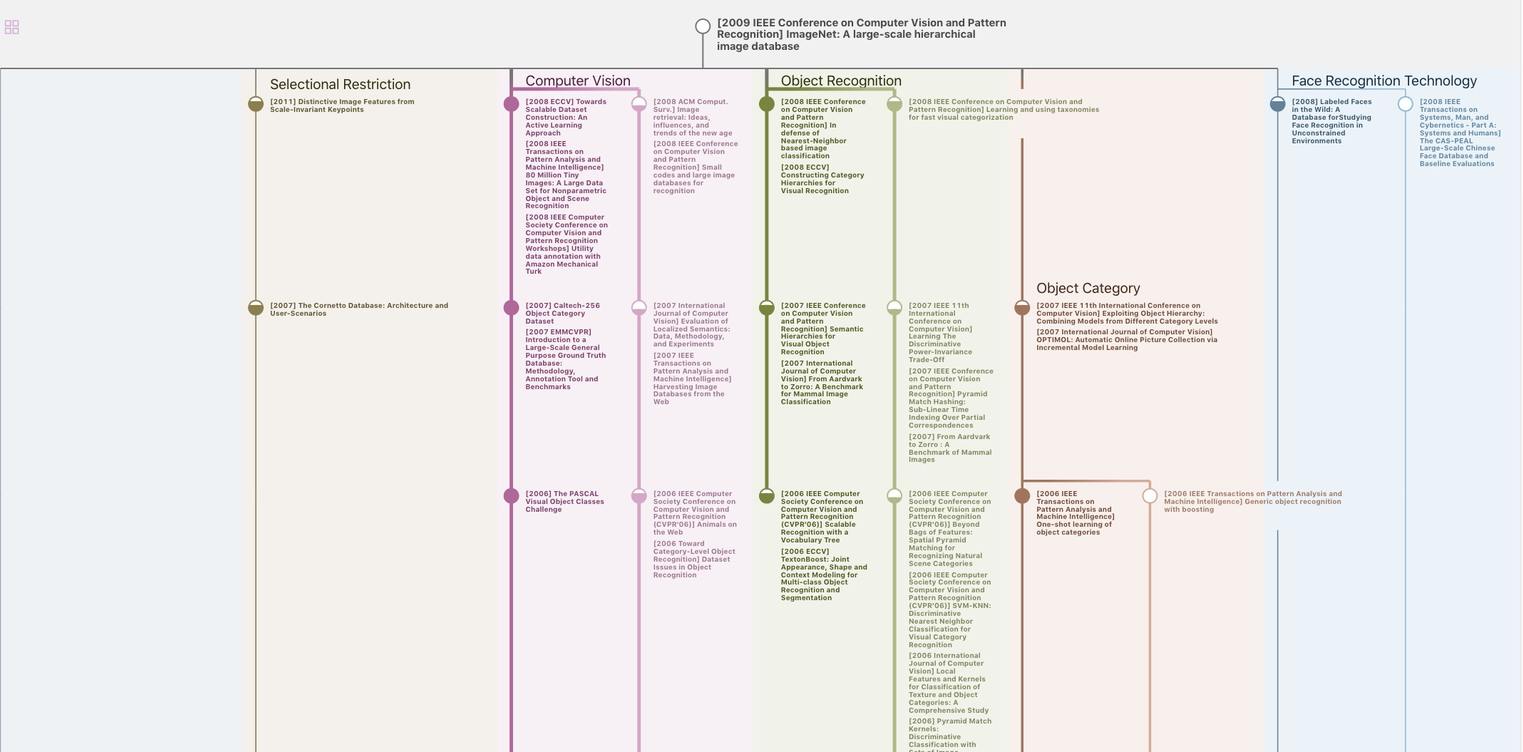
生成溯源树,研究论文发展脉络
Chat Paper
正在生成论文摘要