Development Of A Stochastic Traffic Environment With Generative Time-Series Models For Improving Generalization Capabilities Of Autonomous Driving Agents
2020 IEEE INTELLIGENT VEHICLES SYMPOSIUM (IV)(2020)
摘要
Automated lane changing is a critical feature for advanced autonomous driving systems. In recent years, reinforcement learning (RL) algorithms trained on traffic simulators yielded successful results in computing lane changing policies that strike a balance between safety, agility and compensating for traffic uncertainty. However, many RL algorithms exhibit simulator bias and policies trained on simple simulators do not generalize well to realistic traffic scenarios. In this work, we develop a data driven traffic simulator by training a generative adverserial network (GAN) on real life trajectory data. The simulator generates randomized trajectories that resembles real life traffic interactions between vehicles, which enables training the RL agent on much richer and realistic scenarios. We demonstrate through simulations that RL agents that are trained on GAN-based traffic simulator has stronger generalization capabilities compared to RL agents trained on simple rule-driven simulators.
更多查看译文
关键词
stochastic traffic environment,generative time-series models,improving generalization capabilities,autonomous driving agents,automated lane changing,critical feature,advanced autonomous driving systems,reinforcement learning algorithms,traffic simulators,successful results,lane changing policies,compensating,traffic uncertainty,RL algorithms,simulator bias,simple simulators,realistic traffic scenarios,data driven traffic simulator,generative adverserial network,life trajectory data,life traffic interactions,RL agent,richer scenarios,GAN-based traffic simulator,stronger generalization capabilities,simple rule-driven simulators
AI 理解论文
溯源树
样例
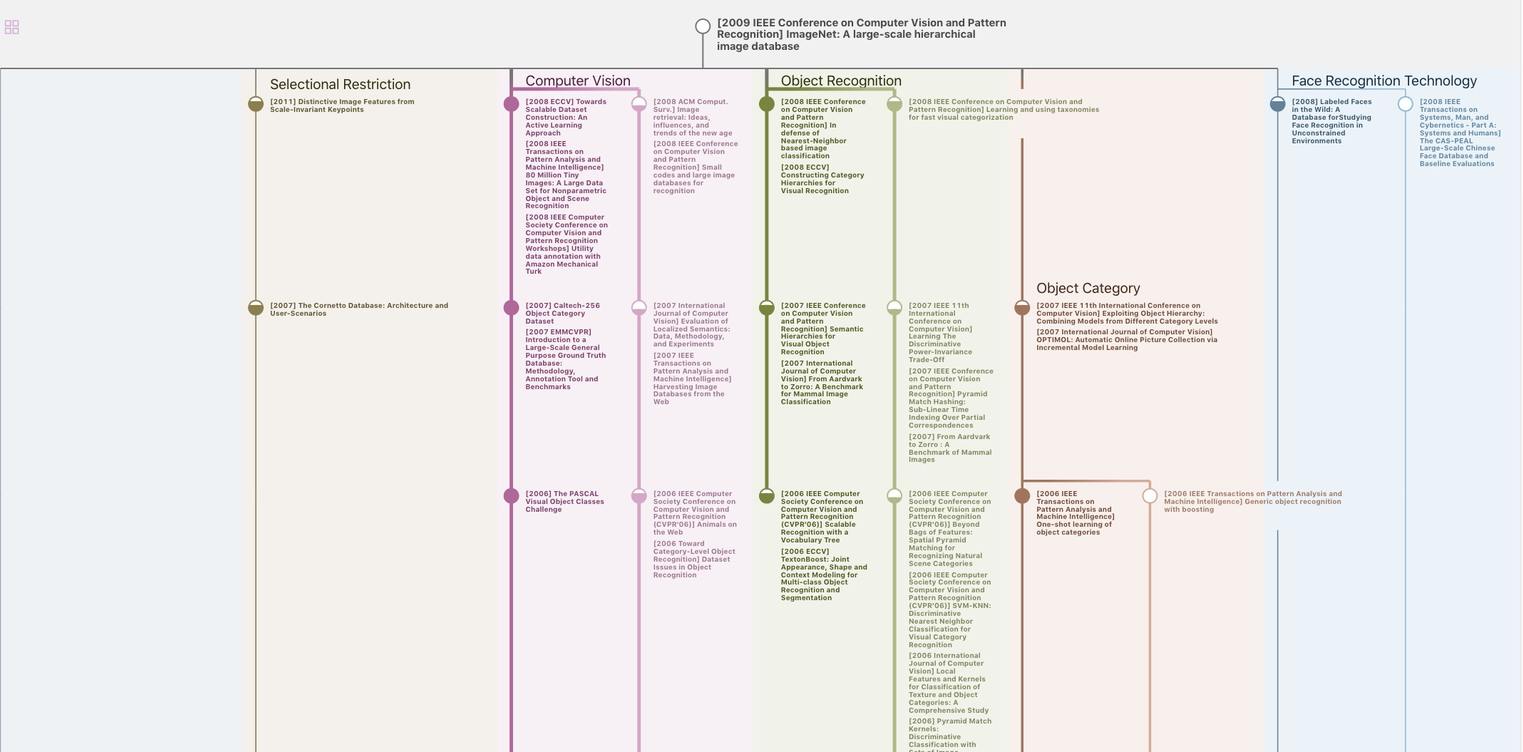
生成溯源树,研究论文发展脉络
Chat Paper
正在生成论文摘要