The Impact of Non-stationarity on Generalisation in Deep Reinforcement Learning
arxiv(2021)
摘要
Non-stationarity arises in Reinforcement Learning (RL) even in stationary environments. Most RL algorithms collect new data throughout training, using a non-stationary behaviour policy. Furthermore, training targets in RL can change even with a fixed state distribution when the policy, critic, or bootstrap values are updated. We study these types of non-stationarity in supervised learning settings as well as in RL, finding that they can lead to worse generalisation performance when using deep neural network function approximators. Consequently, to improve generalisation of deep RL agents, we propose Iterated Relearning (ITER). ITER augments standard RL training by repeated knowledge transfer of the current policy into a freshly initialised network, which thereby experiences less non-stationarity during training. Experimentally, we show that ITER improves performance on the challenging generalisation benchmarks ProcGen and Multiroom.
更多查看译文
关键词
deep reinforcement learning,reinforcement learning,generalisation,non-stationarity
AI 理解论文
溯源树
样例
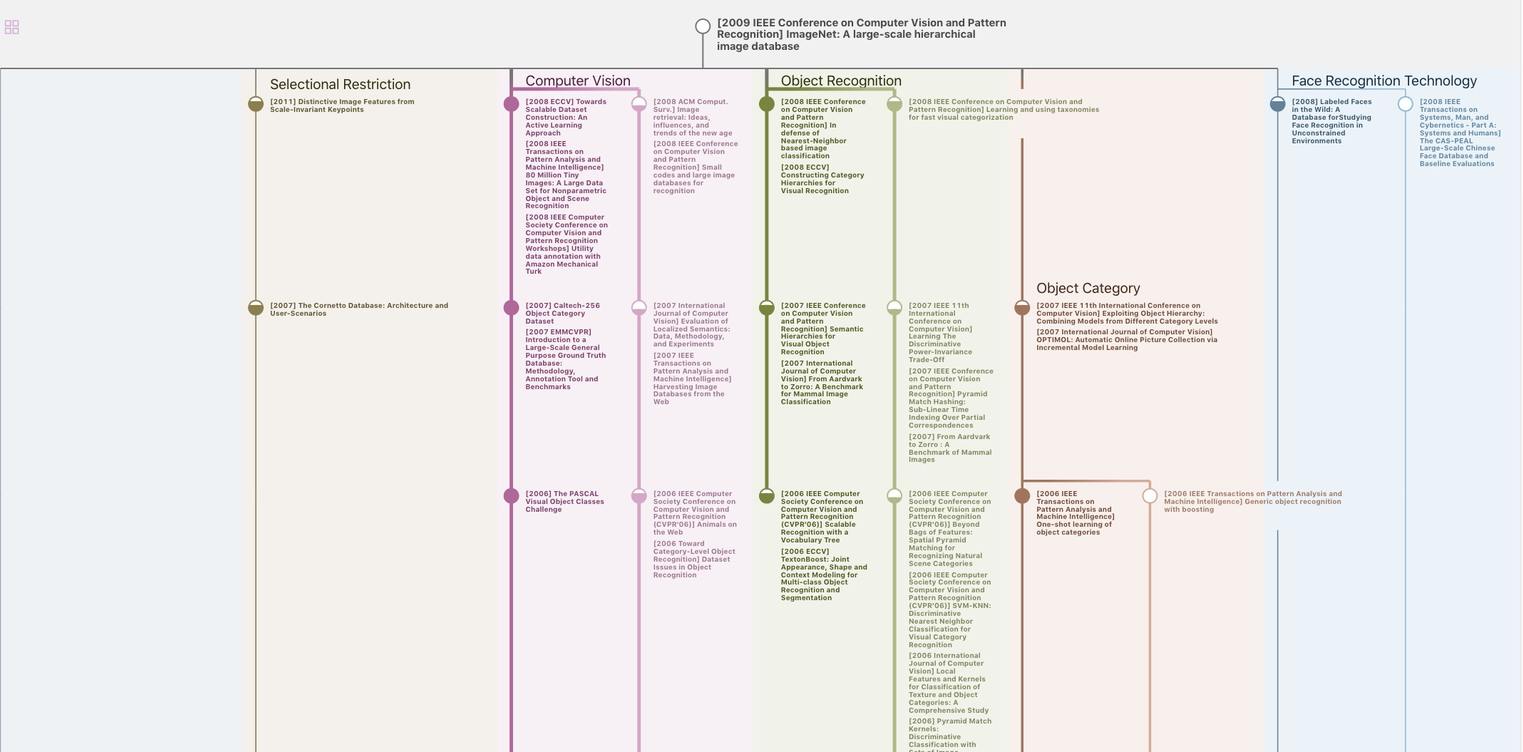
生成溯源树,研究论文发展脉络
Chat Paper
正在生成论文摘要