CoMIR: Contrastive Multimodal Image Representation for Registration
NIPS'20 Proceedings of the 34th International Conference on Neural Information Processing Systems(2020)
摘要
We propose contrastive coding to learn shared, dense image representations,referred to as CoMIRs (Contrastive Multimodal Image Representations). CoMIRsenable the registration of multimodal images where existing registrationmethods often fail due to a lack of sufficiently similar image structures.CoMIRs reduce the multimodal registration problem to a monomodal one, in whichgeneral intensity-based, as well as feature-based, registration algorithms canbe applied. The method involves training one neural network per modality onaligned images, using a contrastive loss based on noise-contrastive estimation(InfoNCE). Unlike other contrastive coding methods, used for, e.g.,classification, our approach generates image-like representations that containthe information shared between modalities. We introduce a novel,hyperparameter-free modification to InfoNCE, to enforce rotational equivarianceof the learnt representations, a property essential to the registration task.We assess the extent of achieved rotational equivariance and the stability ofthe representations with respect to weight initialization, training set, andhyperparameter settings, on a remote sensing dataset of RGB and near-infraredimages. We evaluate the learnt representations through registration of abiomedical dataset of bright-field and second-harmonic generation microscopyimages; two modalities with very little apparent correlation. The proposedapproach based on CoMIRs significantly outperforms registration ofrepresentations created by GAN-based image-to-image translation, as well as astate-of-the-art, application-specific method which takes additional knowledgeabout the data into account. Code is available at:https://github.com/MIDA-group/CoMIR.
更多查看译文
AI 理解论文
溯源树
样例
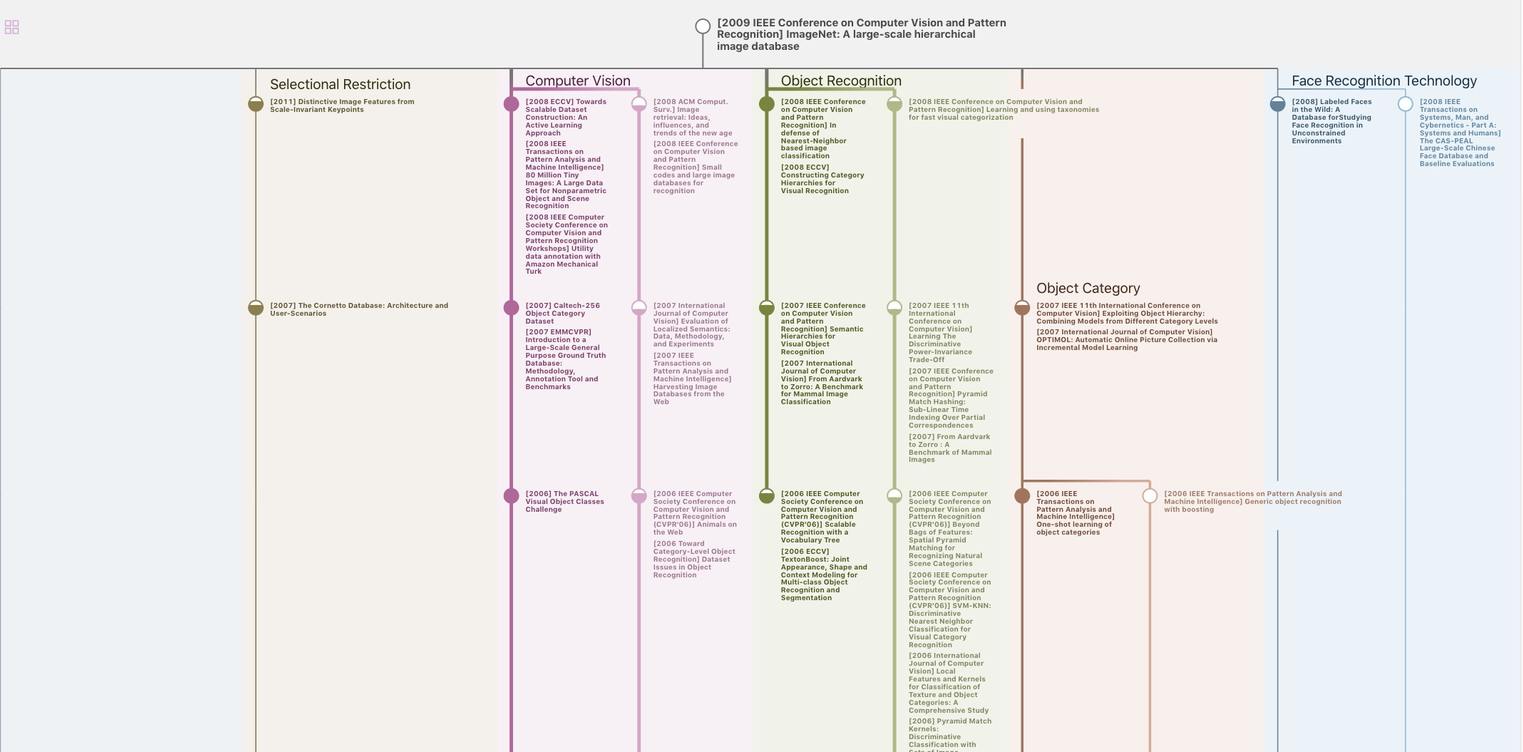
生成溯源树,研究论文发展脉络
Chat Paper
正在生成论文摘要