How Interpretable and Trustworthy are GAMs?
Knowledge Discovery and Data Mining(2021)
摘要
ABSTRACTGeneralized additive models (GAMs) have become a leading model class for interpretable machine learning. However, there are many algorithms for training GAMs, and these can learn different or even contradictory models, while being equally accurate. Which GAM should we trust? In this paper, we quantitatively and qualitatively investigate a variety of GAM algorithms on real and simulated datasets. We find that GAMs with high feature sparsity (only using a few variables to make predictions) can miss patterns in the data and be unfair to rare subpopulations. Our results suggest that inductive bias plays a crucial role in what interpretable models learn and that tree-based GAMs represent the best balance of sparsity, fidelity and accuracy and thus appear to be the most trustworthy GAM models.
更多查看译文
关键词
Generalized Additive Models, Interpretability, Inductive Bias
AI 理解论文
溯源树
样例
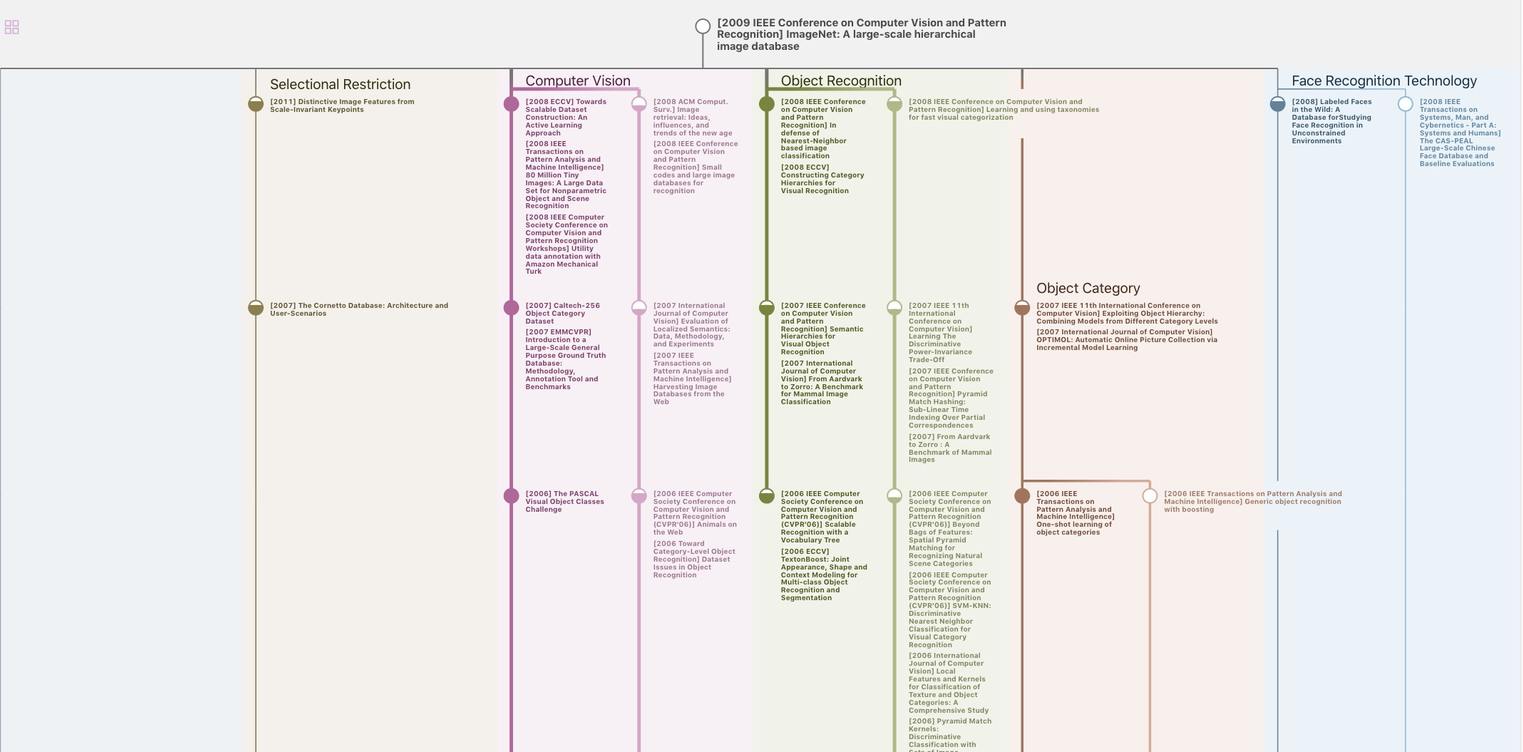
生成溯源树,研究论文发展脉络
Chat Paper
正在生成论文摘要