ClustTR: Clustering Training for Robustness
arxiv(2020)
摘要
This paper studies how encouraging semantically-aligned features during deep neural network training can increase network robustness. Recent works observed that Adversarial Training leads to robust models, whose learnt features appear to correlate with human perception. Inspired by this connection from robustness to semantics, we study the complementary connection: from semantics to robustness. To do so, we provide a tight robustness certificate for distance-based classification models (clustering-based classifiers), which we leverage to propose \emph{ClusTR} (Clustering Training for Robustness), a clustering-based and adversary-free training framework to learn robust models. Interestingly, \textit{ClusTR} outperforms adversarially-trained networks by up to $4\%$ under strong PGD attacks. Moreover, it can be equipped with simple and fast adversarial training to improve the current state-of-the-art in robustness by $16\%$-$29\%$ on CIFAR10, SVHN, and CIFAR100.
更多查看译文
关键词
robustness,clustering
AI 理解论文
溯源树
样例
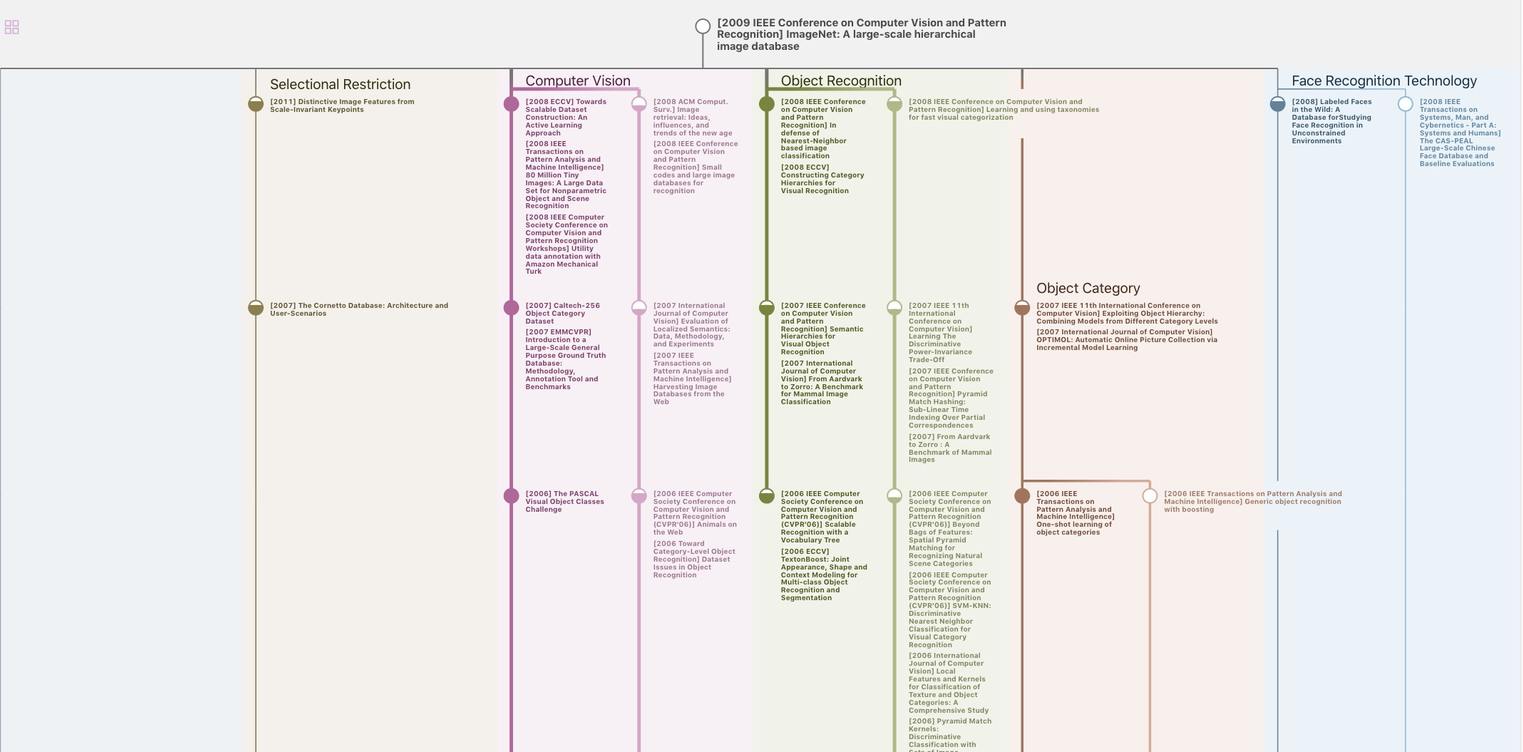
生成溯源树,研究论文发展脉络
Chat Paper
正在生成论文摘要