Class2Simi: A New Perspective on Learning with Label Noise
arxiv(2020)
摘要
Label noise is ubiquitous in the era of big data. Deep learning algorithms can easily fit the noise and thus cannot generalize well without properly modeling the noise. In this paper, we propose a new perspective on dealing with label noise called Class2Simi. Specifically, we transform the training examples with noisy class labels into pairs of examples with noisy similarity labels and propose a deep learning framework to learn robust classifiers directly with the noisy similarity labels. Note that a class label shows the class that an instance belongs to; while a similarity label indicates whether or not two instances belong to the same class. It is worthwhile to perform the transformation: We prove that the noise rate for the noisy similarity labels is lower than that of the noisy class labels, because similarity labels themselves are robust to noise. For example, given two instances, even if both of their class labels are incorrect, their similarity label could be correct. Due to the lower noise rate, Class2Simi achieves remarkably better classification accuracy than its baselines that directly deals with the noisy class labels.
更多查看译文
AI 理解论文
溯源树
样例
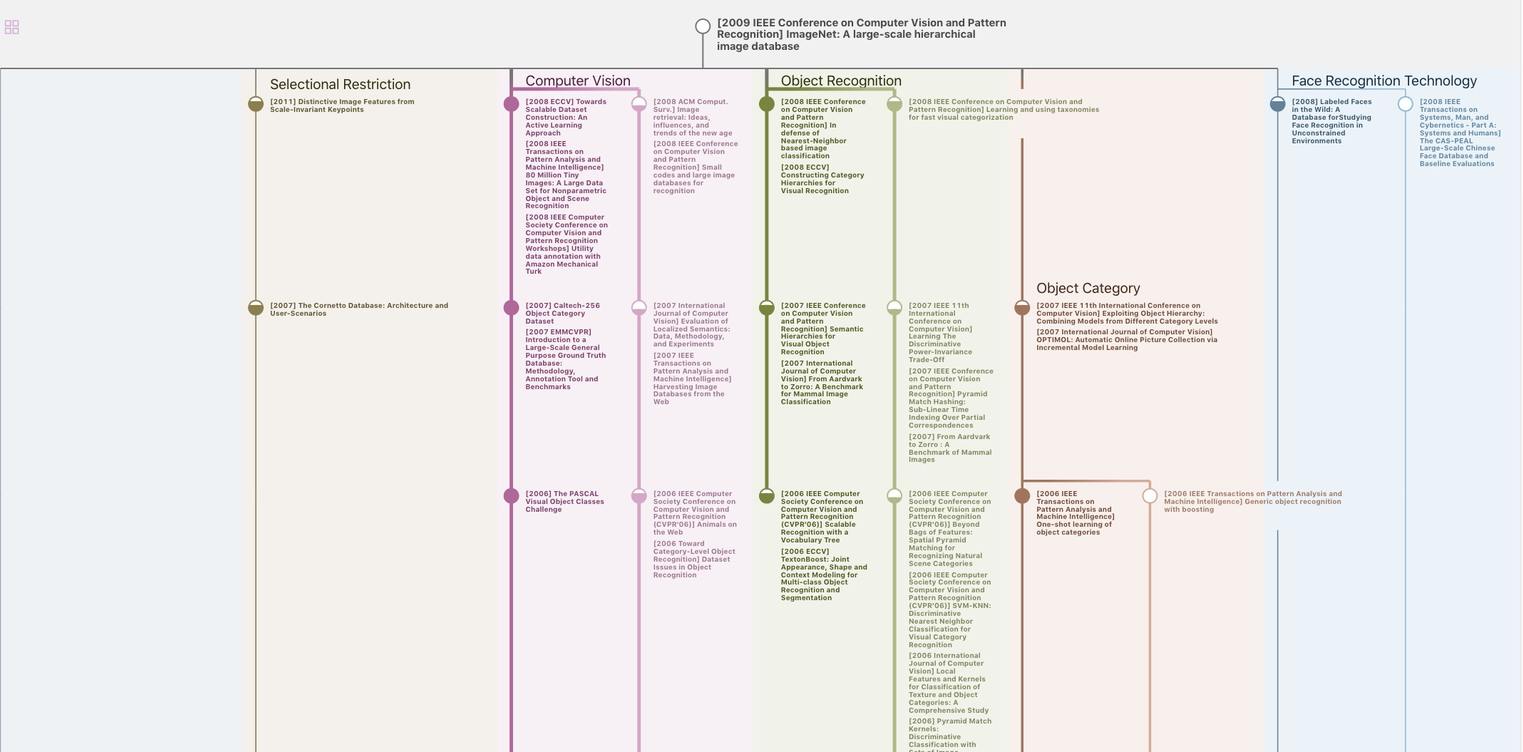
生成溯源树,研究论文发展脉络
Chat Paper
正在生成论文摘要